Deep Learning From Multiple Noisy Annotators as A Union.
IEEE transactions on neural networks and learning systems(2022)
摘要
Crowdsourcing is a popular solution for large-scale data annotations. So far, various end-to-end deep learning methods have been proposed to improve the practical performance of learning from crowds. Despite their practical effectiveness, most of them have two major limitations--they do not hold learning consistency and suffer from computational inefficiency. In this article, we propose a novel method named UnionNet, which is not only theoretically consistent but also experimentally effective and efficient. Specifically, unlike existing methods that either fit a given label from each annotator independently or fuse all the labels into a reliable one, we concatenate the one-hot encoded vectors of crowdsourced labels provided by all the annotators, which takes all the labeling information as a union and coordinates multiple annotators. In this way, we can directly train an end-to-end deep neural network by maximizing the likelihood of this union with only a parametric transition matrix. We theoretically prove the learning consistency and experimentally show the effectiveness and efficiency of our proposed method.
更多查看译文
关键词
Training,Deep learning,Labeling,Noise measurement,Neural networks,Standards,Learning systems,Annotators,crowdsourcing,noisy labels,transition matrix
AI 理解论文
溯源树
样例
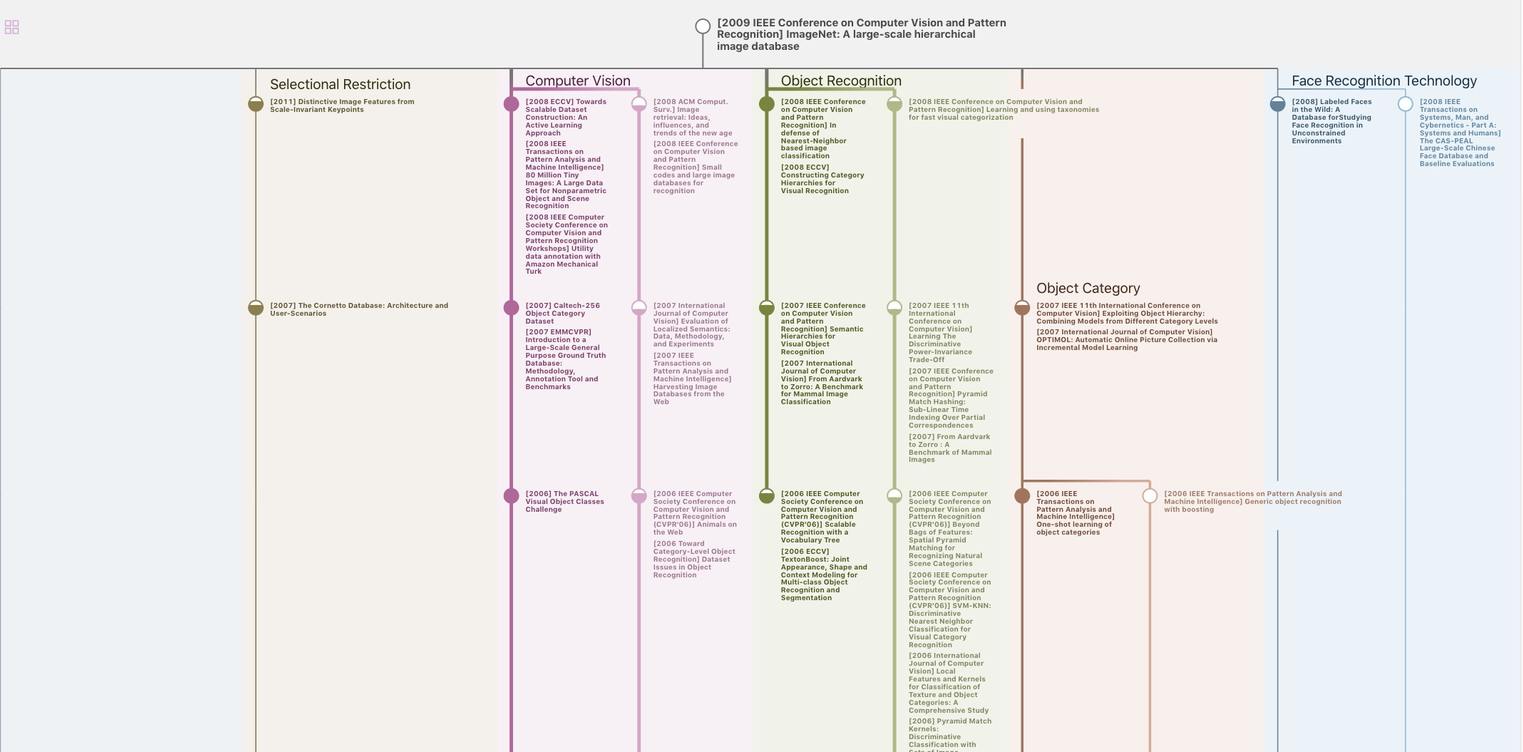
生成溯源树,研究论文发展脉络
Chat Paper
正在生成论文摘要