Learning Transferable Human-Object Interaction Detector with Natural Language Supervision
2022 IEEE/CVF CONFERENCE ON COMPUTER VISION AND PATTERN RECOGNITION (CVPR 2022)(2022)
摘要
It is difficult to construct a data collection including all possible combinations of human actions and interacting objects due to the combinatorial nature of human-object interactions (HOI). In this work, we aim to develop a transferable HOI detector for unseen interactions. Existing HOI detectors often treat interactions as discrete labels and learn a classifier according to a predetermined category space. This is inherently inapt for detecting unseen interactions which are out of the predefined categories. Conversely, we treat independent HOI labels as the natural language supervision of interactions and embed them into a joint visual-and-text space to capture their correlations. More specifically, we propose a new HOI visual encoder to detect the interacting humans and objects, and map them to a joint feature space to perform interaction recognition. Our visual encoder is instantiated as a Vision Transformer with new learnable HOI tokens and a sequence parser to generate unique HOI predictions. It distills and leverages the transferable knowledge from the pretrained CLIP model to perform the zero-shot interaction detection. Experiments on two datasets, SWIG-HOI and HICO-DET, validate that our proposed method can achieve a notable mAP improvement on detecting both seen and unseen HOIs. Our code is available at https://github.com/scwangdyd/promting_hoi.
更多查看译文
关键词
Recognition: detection,categorization,retrieval,Action and event recognition
AI 理解论文
溯源树
样例
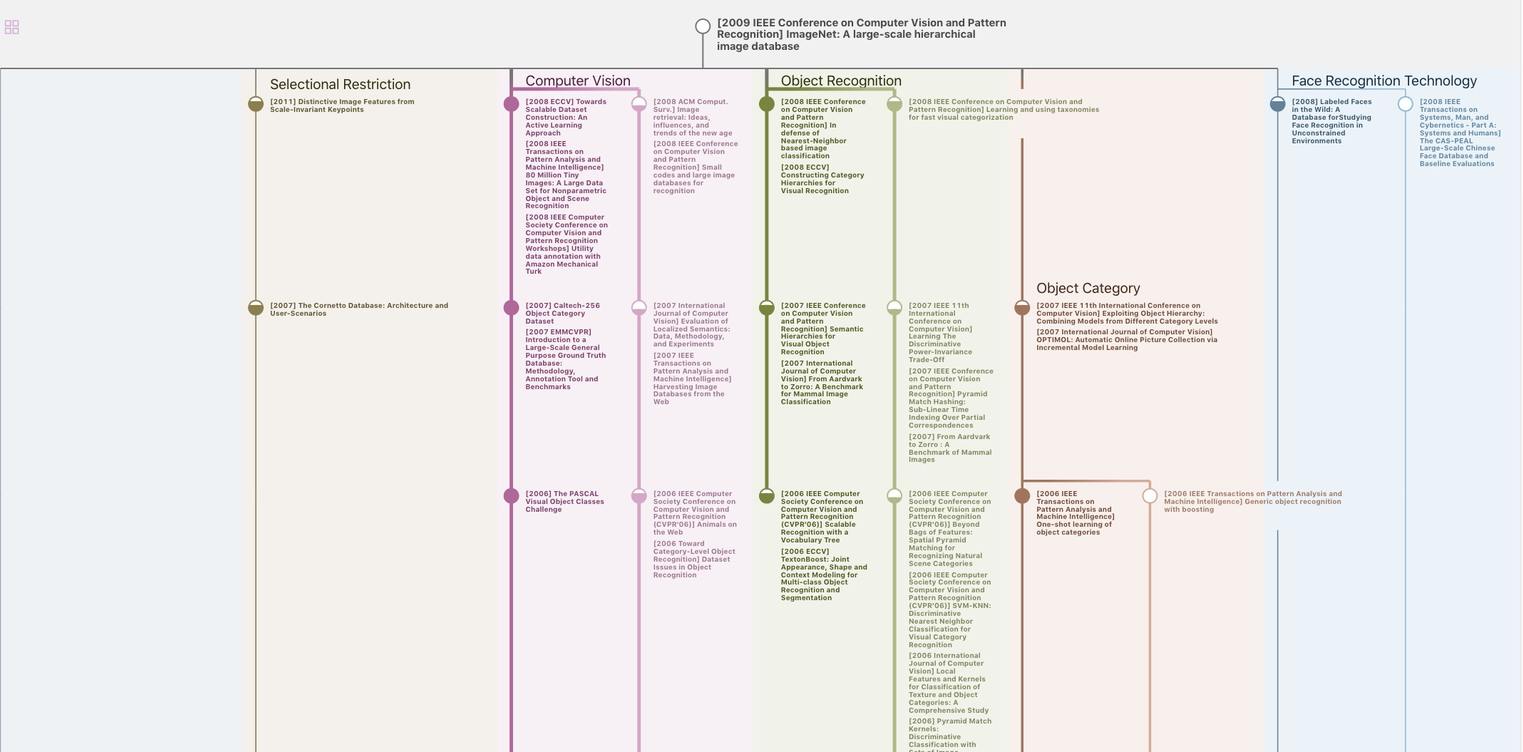
生成溯源树,研究论文发展脉络
Chat Paper
正在生成论文摘要