Towards Functionally Robust AI Accelerators
2021 IEEE Microelectronics Design & Test Symposium (MDTS)(2021)
摘要
Recent advances in deep learning can be attributed to the continued performance improvement of hardware processors and artificial intelligence (AI) accelerators. In addition to conventional CMOS accelerators based on Von Neumann architecture, emerging technologies such as silicon photonics, memristors, and monolithic 3D (M3D) integration are being explored as post-Moore's law alternatives. However, the energy efficiency and performance of emerging AI accelerators can be catastrophically impacted by faults due to fabrication-process variations, thermal crosstalk, and aging. In this paper, we analyze the performance of several emerging AI accelerators in the presence of different uncertainties, and present low-cost methods to assess the significance of faults and mitigate their effects. We show that across all technologies, the impact of uncertainties on the performance can vary significantly based on the fault type and the parameters of the affected component. Therefore, the fault criticality-assessment techniques presented in this paper are necessary for yield improvement.
更多查看译文
关键词
deep learning,continued performance improvement,hardware processors,artificial intelligence accelerators,conventional CMOS accelerators,Von Neumann architecture,monolithic 3D integration,post-Moore's law alternatives,emerging AI accelerators,functionally robust AI accelerators,fault criticality-assessment techniques
AI 理解论文
溯源树
样例
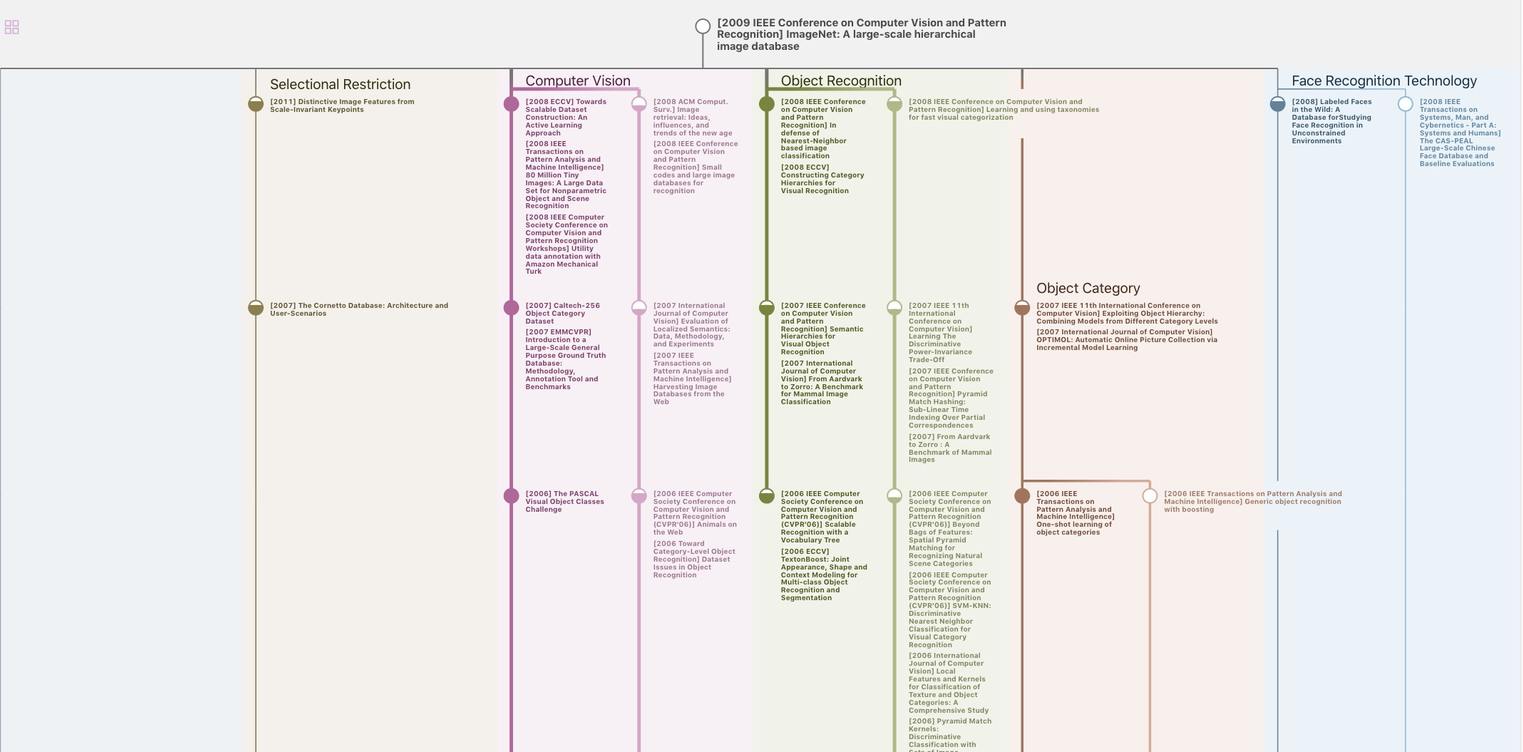
生成溯源树,研究论文发展脉络
Chat Paper
正在生成论文摘要