Improving Obstacle Boundary Representations in Predictive Occupancy Mapping
Robotics and autonomous systems(2022)
Abstract
Predictive, inference-based occupancy mapping has been used successfully in many instances to create accurate and descriptive maps from sparse data, defining occupied space in a manner suitable to support autonomous navigation. However, one key drawback of inferring occupancy based largely on the proximity of range sensor observations is inaccuracy at the boundary between occupied and free space, where sparse coverage by the sensor data can be misinterpreted. To obtain a more accurate representation of the boundary between free and occupied space, we propose several modifications to a recently published occupancy mapping algorithm that uses Bayesian generalized kernel inference. In particular, our proposed algorithm distinguishes between unknown map cells with insufficient observations, and those which are uncertain due to disagreement among numerous observations, in a predictive, inference-based occupancy map. This distinction is key to our improved ability to capture ambiguities arising at the boundary between free and occupied space. We validate our approach using synthetic range data from a simulated environment and demonstrate real-time mapping performance using range data acquired by a ground robot operating in an underground mine.
MoreTranslated text
Key words
Mapping,Range sensing,Mobile robotics
AI Read Science
Must-Reading Tree
Example
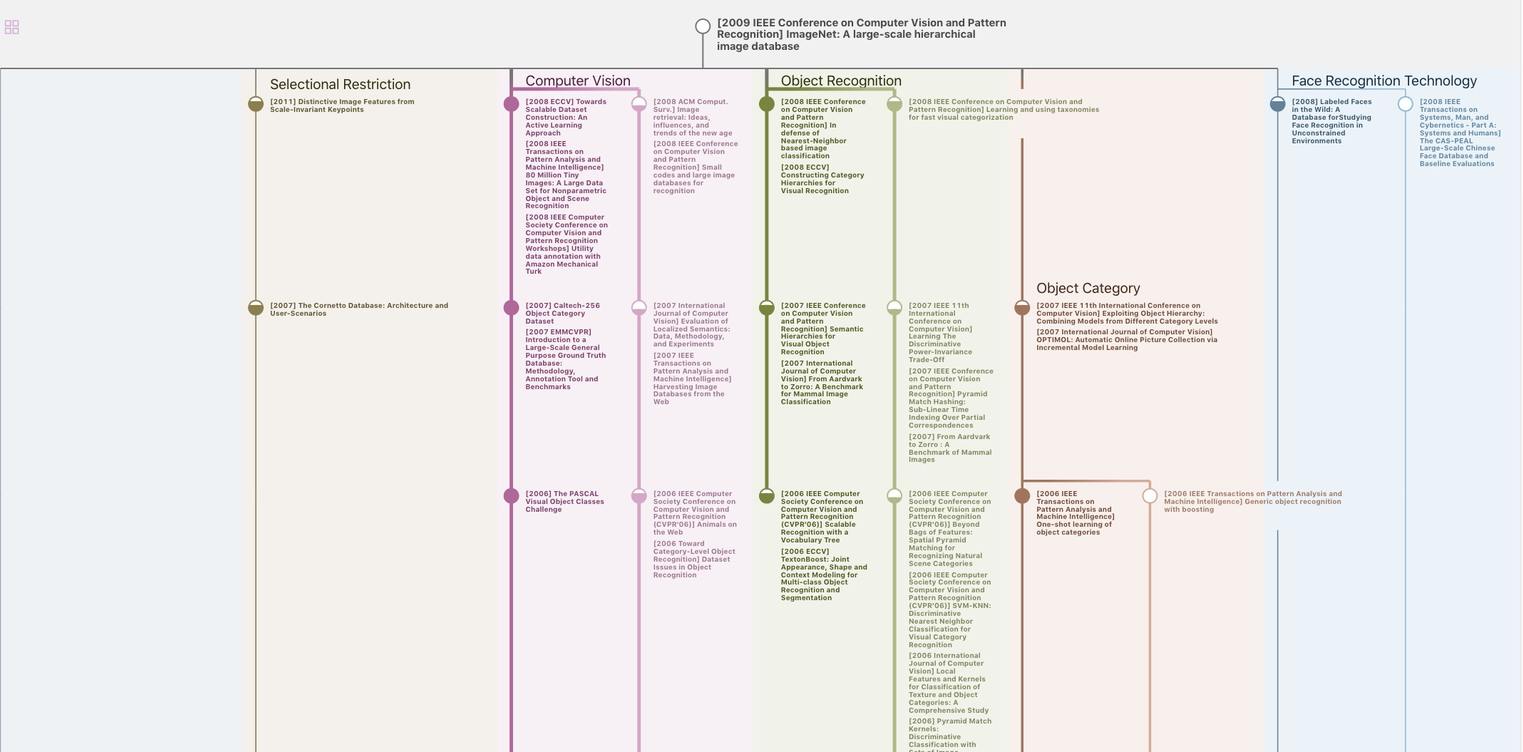
Generate MRT to find the research sequence of this paper
Chat Paper
Summary is being generated by the instructions you defined