An Energy-Efficient High CSNR XNOR and Accumulation Scheme for BNN
IEEE Transactions on Circuits and Systems II: Express Briefs(2022)
摘要
In this brief, we present an energy-efficient and high compute signal-to-noise ratio (CSNR) XNOR and accumulation (XAC) scheme for binary neural networks (BNNs). Transmission gates achieve a large compute signal margin (CSM) and high CSNR for accurate XAC operation. The 10T1C XNOR SRAM bit-cell performs the in-memory XAC operation without pre-charging the larger bitline capacitances and significantly reducing energy consumption per XAC operation. The validation of the proposed XAC scheme is done through the post-layout simulations in 65nm CMOS technology with
$V_{DD}$
of 1 V. The achieved 1 ns of latency and 2.36 fJ of energy consumption per XAC operation are (
$7.2\times $
,
$7.2\times $
) and (
$2\times $
,
$1.31\times $
) lower than state-of-the-art digital and analog compute in-memory (CIM) XAC schemes respectively. The proposed XAC design achieves
$8.6\times $
improvement in figure-of-merit (FoM), over prior state-of-the-art. Moreover, (
$\sigma /\mu $
) average of 0.2% from Monte Carlo simulations show that proposed XAC scheme is robust against systematic mismatch and process variations.
更多查看译文
关键词
Accuracy,accumulation,artificial intelligence (AI),compute signal margin (CSM),compute signal to noise ratio (CSNR),energy-efficiency,latency,neuron,SRAM
AI 理解论文
溯源树
样例
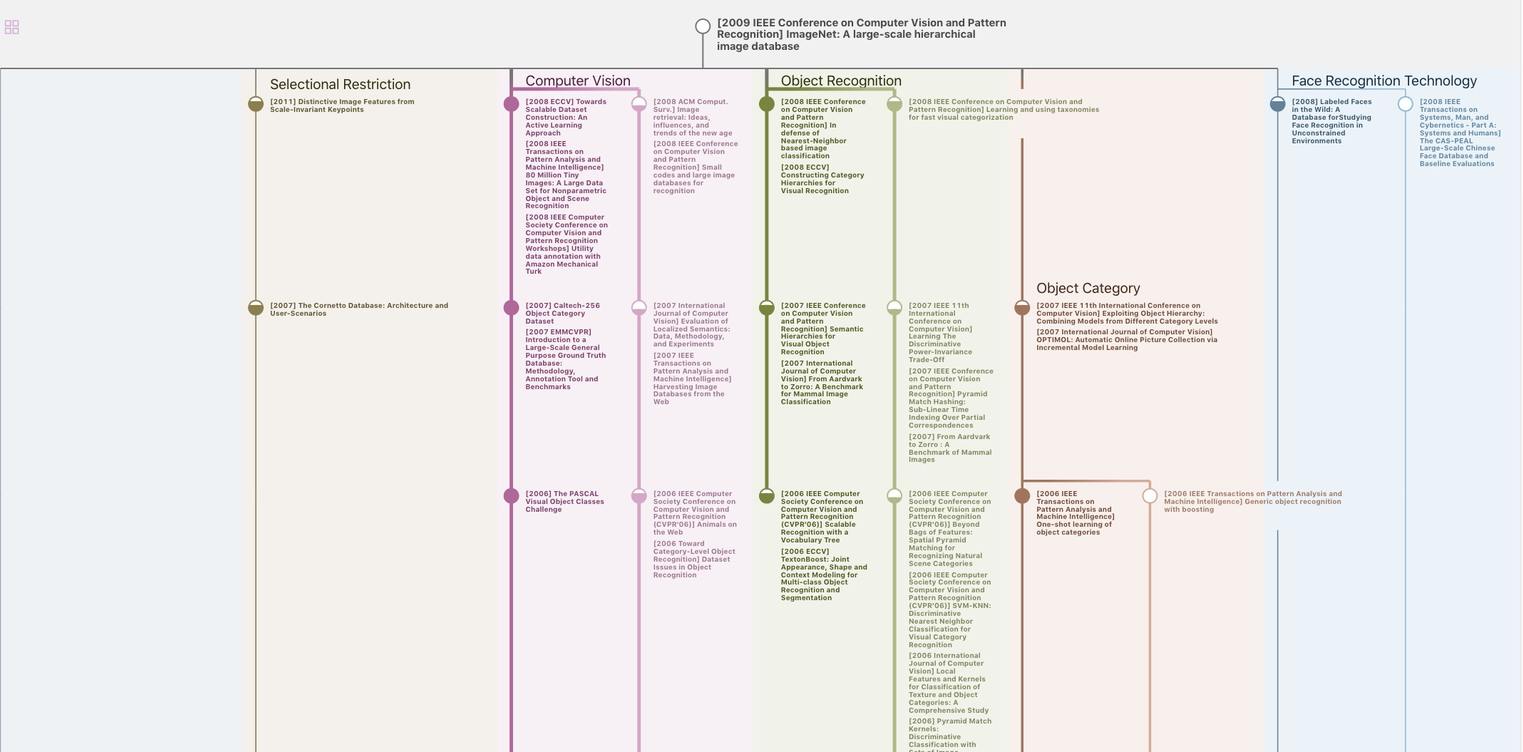
生成溯源树,研究论文发展脉络
Chat Paper
正在生成论文摘要