Proposed Big Data Architecture for Facial Recognition Using Machine Learning
AIMS Electronics and Electrical Engineering(2021)
1. Institute of Systems Science | 2. Department of Information Technology
Abstract
With the abundance of raw data generated from various sources including social networks, big data has become essential in acquiring, processing, and analyzing heterogeneous data from multiple sources for real-time applications. In this paper, we propose a big data framework suitable for pre‑processing and classification of image as well as text analytics by employing two key workflows, called big data (BD) pipeline and machine learning (ML) pipeline. Our unique end-to-end workflow integrates data cleansing, data integration, data transformation and data reduction processes, followed by various analytics using suitable machine learning techniques. Further, our model is the first of its kind to augment facial recognition with sentiment analysis in a distributed big data framework. The implementation of our model uses state-of-the-art distributed technologies to ingest, prepare, process and analyze big data for generating actionable data insights by employing relevant ML algorithms such as k-NN, logistic regression and decision tree. In addition, we demonstrate the application of our big data framework to facial recognition system using open sources by developing a prototype as a use case. We also employ sentiment analysis on non-repetitive semi structured public data (text) such as user comments, image tagging, and other information associated with the facial images. We believe our work provides a novel approach to intersect Big Data, ML and Face Recognition and would create new research to alleviate some of the challenges associated with big data processing in real world applications.
MoreTranslated text
Key words
big data,machine learning,social networks,sentiment analysis,facial recognition,distributed computing
求助PDF
上传PDF
View via Publisher
AI Read Science
AI Summary
AI Summary is the key point extracted automatically understanding the full text of the paper, including the background, methods, results, conclusions, icons and other key content, so that you can get the outline of the paper at a glance.
Example
Background
Key content
Introduction
Methods
Results
Related work
Fund
Key content
- Pretraining has recently greatly promoted the development of natural language processing (NLP)
- We show that M6 outperforms the baselines in multimodal downstream tasks, and the large M6 with 10 parameters can reach a better performance
- We propose a method called M6 that is able to process information of multiple modalities and perform both single-modal and cross-modal understanding and generation
- The model is scaled to large model with 10 billion parameters with sophisticated deployment, and the 10 -parameter M6-large is the largest pretrained model in Chinese
- Experimental results show that our proposed M6 outperforms the baseline in a number of downstream tasks concerning both single modality and multiple modalities We will continue the pretraining of extremely large models by increasing data to explore the limit of its performance
Upload PDF to Generate Summary
Must-Reading Tree
Example
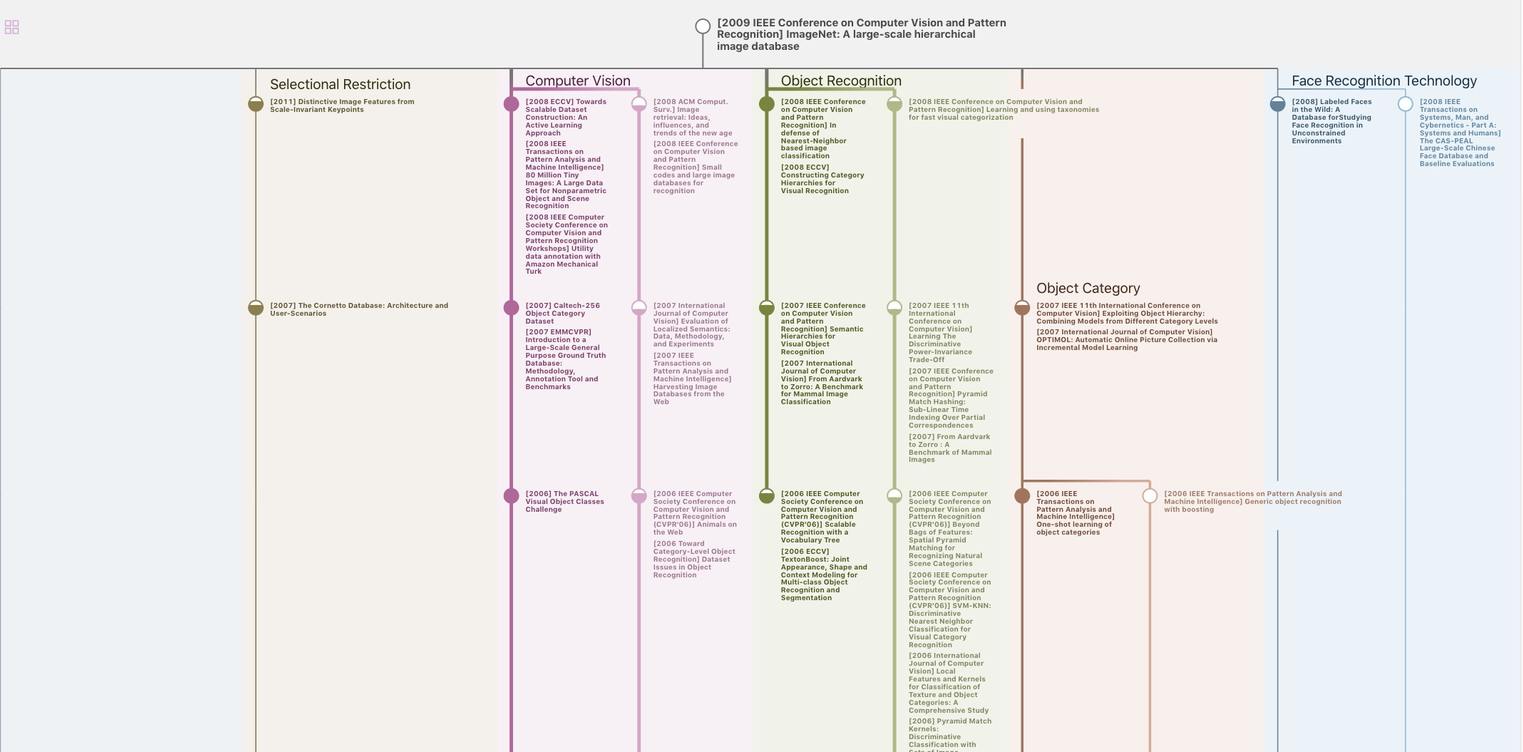
Generate MRT to find the research sequence of this paper
Related Papers
MOBILE INFORMATION SYSTEMS 2022
被引用1
Vaccine Sentiment Analysis Using BERT + NBSVM and Geo-Spatial Approaches.
JOURNAL OF SUPERCOMPUTING 2023
被引用10
Security Threats in Healthcare Big Data: A Comparative Study
2023 International Conference on Computational Intelligence and Sustainable Engineering Solutions (CISES) 2023
被引用0
Deep CNN with Late Fusion for Real Time Multimodal Emotion Recognition
EXPERT SYSTEMS WITH APPLICATIONS 2024
被引用1
A Hybrid Facial Recognition Approach Based on Grasshopper Optimization Method
PROCEEDINGS OF THE 4TH INTERNATIONAL COMPUTER SCIENCES AND INFORMATICS CONFERENCE (ICSIC 2022) AIP Conference Proceedings 2023
被引用0
Data Disclaimer
The page data are from open Internet sources, cooperative publishers and automatic analysis results through AI technology. We do not make any commitments and guarantees for the validity, accuracy, correctness, reliability, completeness and timeliness of the page data. If you have any questions, please contact us by email: report@aminer.cn
Chat Paper