Learning Robot Manipulation Skills From Human Demonstration Videos Using Two-Stream 2-D/3-D Residual Networks With Self-Attention
IEEE Transactions on Cognitive and Developmental Systems(2023)
摘要
Learning manipulation skills from observing human demonstration video is a promising aspect for intelligent robotic systems. Recent advances in Video-to-Command (V2C) provide an end-to-end approach to translate a video into robot plans. However, simultaneous V2C and action segmentation remain a major challenge for bimanual manipulations with fine-grained actions. Another concern is the generalization capability of end-to-end approaches in dealing with varied task parameters as well as environmental changes between the learned skills and the one-shot task demonstration for the robot to replay. In this article, we propose a two-stream network for robots to learn and segment manipulation subactions from human demonstration videos. Our framework with the self-attention mechanism can segment learned skills and generate action commands simultaneously. To arrive at refined plans in situations of underspecified or redundant human demonstrations, we utilize PDDL-based skill scripts to model the semantics of demonstrated activities and infer latent movements. Experimental results on the extended manipulation data set indicate that our approach generates more accurate commands than the state-of-the-art methods. Real-world experiment results on a Baxter robotic arm also demonstrated the feasibility of our method in reproducing fine-grained actions from video demonstrations.
更多查看译文
关键词
Learning from Demonstration (LfD),robot manipulation,self-attention,skills learning,Video-to-Command (V2C)
AI 理解论文
溯源树
样例
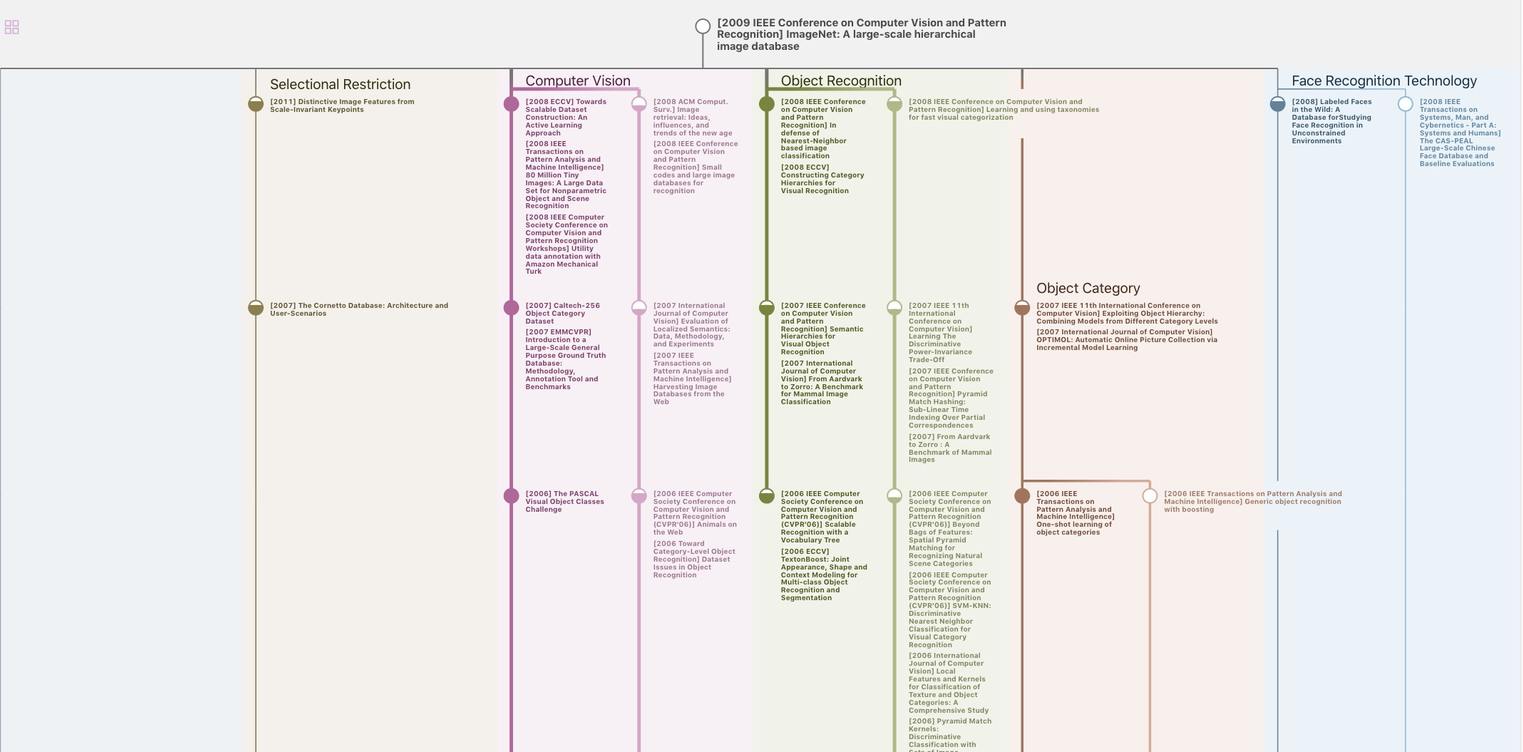
生成溯源树,研究论文发展脉络
Chat Paper
正在生成论文摘要