Using big data and federated learning for generating energy efficiency recommendations
INTERNATIONAL JOURNAL OF DATA SCIENCE AND ANALYTICS(2022)
摘要
Internet of Things (IoT) devices are becoming popular solutions for smart home and office environments and contribute the most to energy efficiency. The most common implementation of such solutions relies on smart home systems that are hosted on the cloud. They collect data from a multitude of sensors, process it in real-time on the cloud and deliver immediate actions to sets of actuators that are installed locally. In this work, we present the (EM) ^3 project (Consumer Engagement towards Energy Saving Behaviour by Means of Exploiting Micro Moments and Mobile Recommendation Systems), which combines data collection, information abstraction, timed recommendations for energy saving actions and automations that promote energy saving in a household or office setup. The advantage of the (EM) ^3 project is that each room or office setup is controlled locally on an edge device, thus removing the need to share data to the cloud. The current article details on the data and information processing aspects of the (EM) ^3 solution, which efficiently handles thousands of sensor events on a daily basis and provides useful analytics and recommendations to the end user to support habit change. It also demonstrates the scalability of the solution by simulating a simple scenario of distributed data collection and processing on the edge nodes, which takes advantage of federated learning in order to adapt to the needs of multiple users without exposing their privacy.
更多查看译文
关键词
Big data collection, Edge information processing, Information abstraction, Habit change, Timed recommendations
AI 理解论文
溯源树
样例
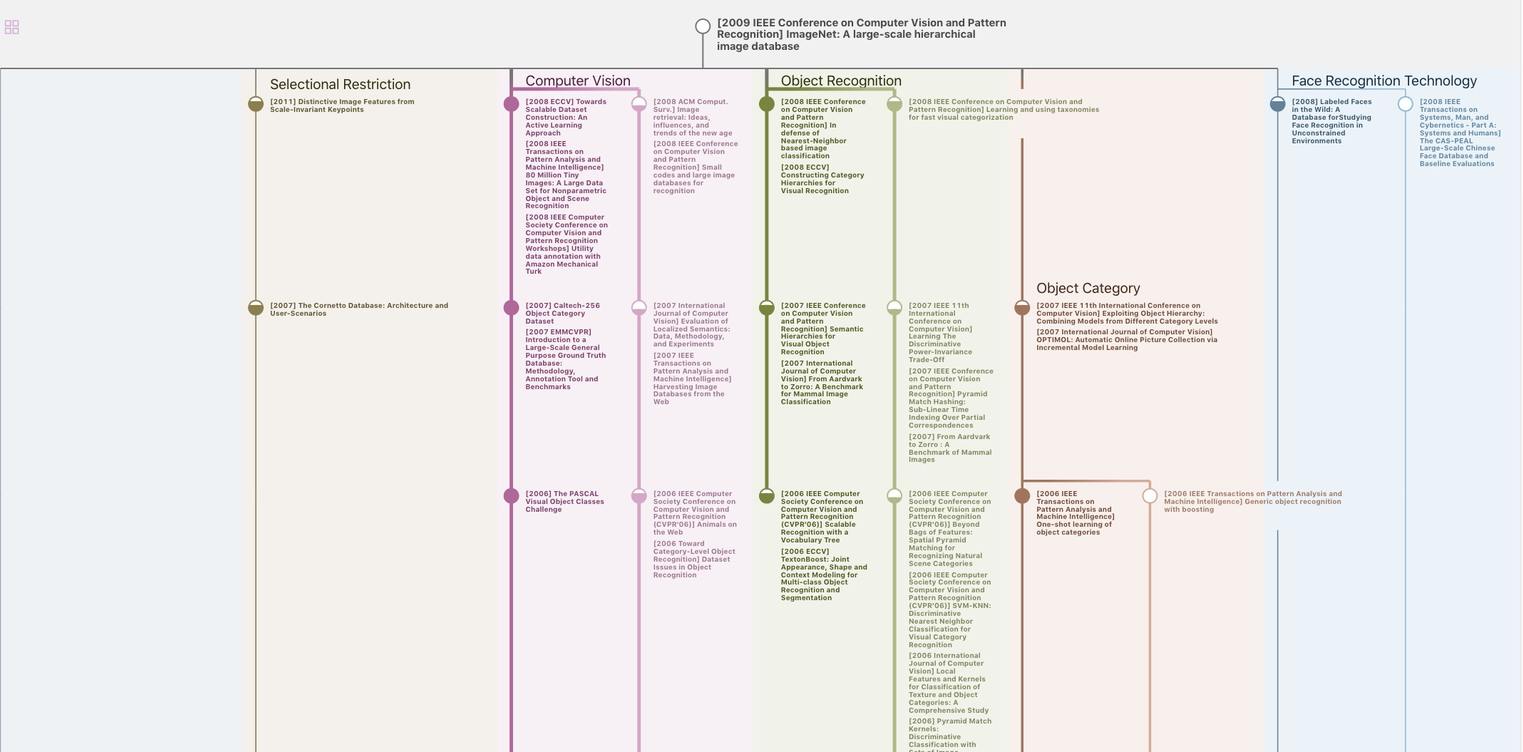
生成溯源树,研究论文发展脉络
Chat Paper
正在生成论文摘要