Multimodal Classification of Safety-Report Observations
APPLIED SCIENCES-BASEL(2022)
摘要
Featured Application This work's contributions can be applied to the development of automatic systems for detecting and assessing safety issues in work places and public spaces, given observations that contain multimedia cues. Modern businesses are obligated to conform to regulations to prevent physical injuries and ill health for anyone present on a site under their responsibility, such as customers, employees and visitors. Safety officers (SOs) are engineers, who perform site audits to businesses, record observations regarding possible safety issues and make appropriate recommendations. In this work, we develop a multimodal machine-learning architecture for the analysis and categorization of safety observations, given textual descriptions and images taken from the location sites. For this, we utilize a new multimodal dataset, Safety4All, which contains 5344 safety-related observations created by 86 SOs in 486 sites. An observation consists of a short issue description, written by the SOs, accompanied with images where the issue is shown, relevant metadata and a priority score. Our proposed architecture is based on the joint fine tuning of large pretrained language and image neural network models. Specifically, we propose the use of a joint task and contrastive loss, which aligns the text and vision representations in a joint multimodal space. The contrastive loss ensures that inter-modality representation distances are maintained, so that vision and language representations for similar samples are close in the shared multimodal space. We evaluate the proposed model on three tasks, namely, priority classification of input observations, observation assessment and observation categorization. Our experiments show that inspection scene images and textual descriptions provide complementary information, signifying the importance of both modalities. Furthermore, the use of the joint contrastive loss produces strong multimodal representations and outperforms a baseline simple model in tasks fusion. In addition, we train and release a large transformer-based language model for the Greek language based on the Electra architecture.
更多查看译文
关键词
occupational safety and health (OSH), safety reports, multimodal fusion, text-visual, contrastive learning, text classification
AI 理解论文
溯源树
样例
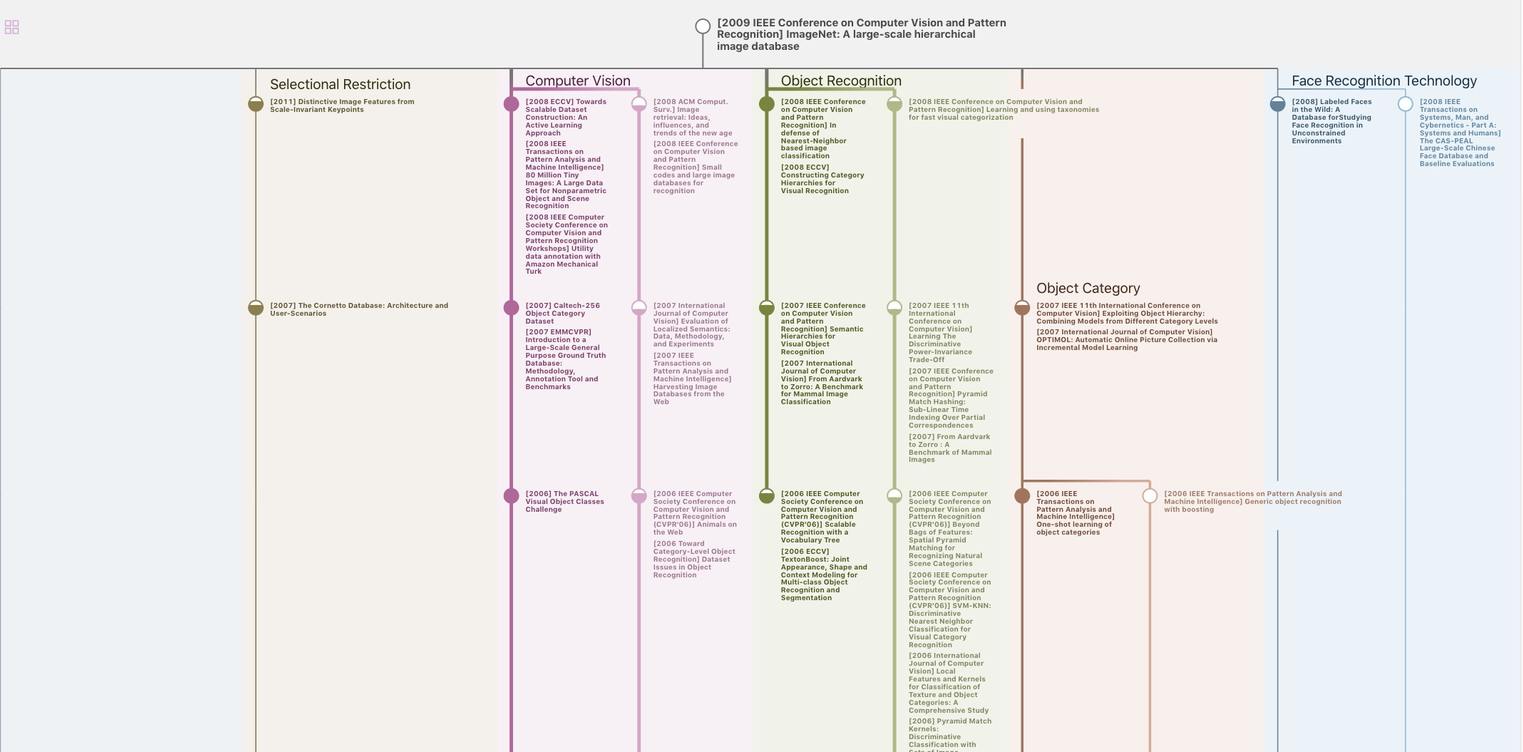
生成溯源树,研究论文发展脉络
Chat Paper
正在生成论文摘要