SSL Enables Learning from Sparse Rewards in Image-Goal Navigation.
International Conference on Machine Learning(2022)
摘要
Visual navigation through novel environments is an essential skill for intelligent household robots. Reinforcement learning (RL) with dense reward shaping is the standard approach for such visual navigation problems. Dense rewards are used despite the fact that they penalize exploration—a key skill for navigating in new environments—because of the commonly held belief that sparse rewards do not work. In this paper, we present a surprising finding. We demonstrate that combining sparse rewards with self-supervised learning (SSL) not only makes them work, but also outperforms dense rewards, which is the first result of this kind. We apply our approach alongside data augmentation to train a general-purpose agent for image-goal navigation (ImageNav), in which the goal is presented as an image captured from the target location. These techniques improve navigation success from 64% to 72% (+8), path efficiency—measured by success weighted by path length (SPL)—from 0.50 to 0.62 (+0.12), and also lead to a +0.06 absolute improvement in SPL over the state-of-the-art.
更多查看译文
AI 理解论文
溯源树
样例
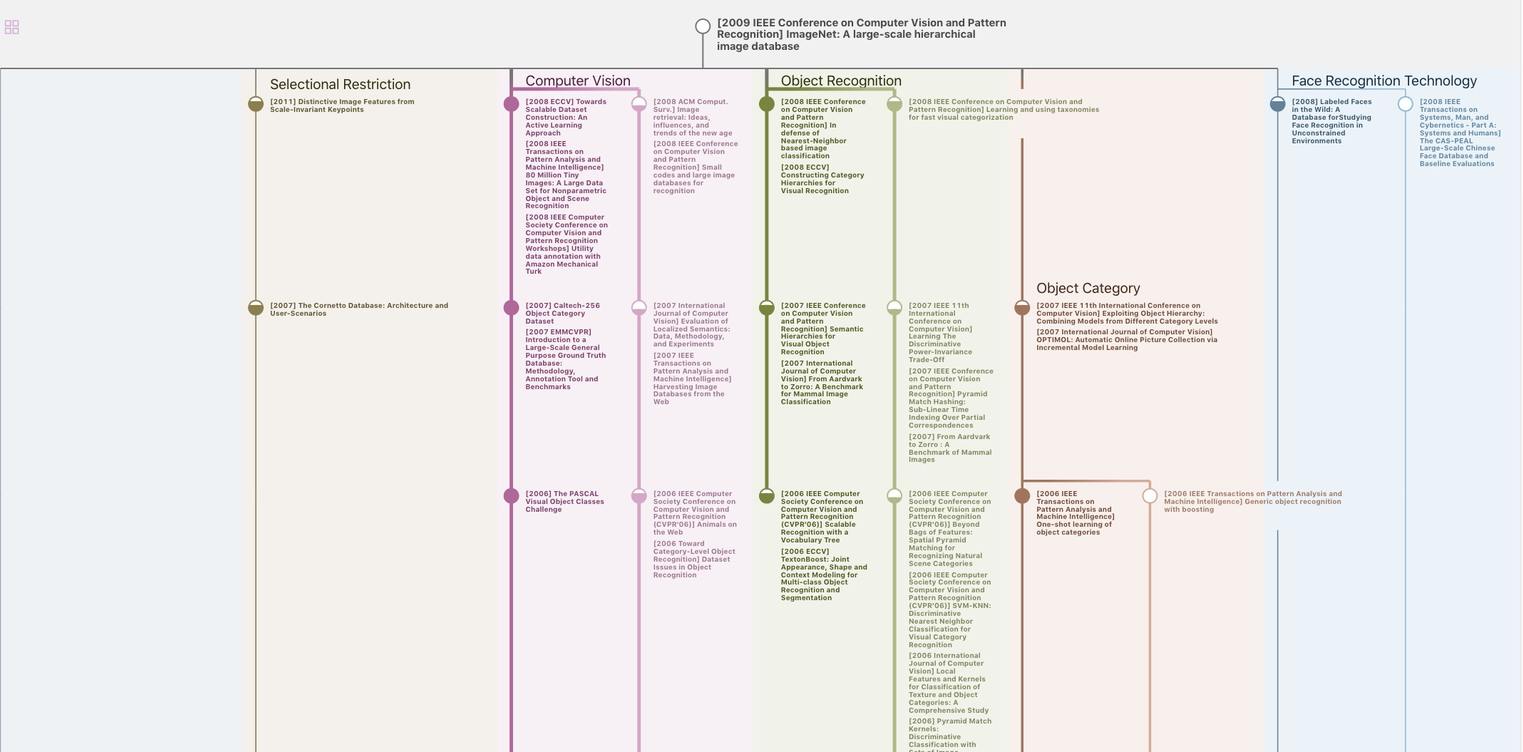
生成溯源树,研究论文发展脉络
Chat Paper
正在生成论文摘要