Model Identification and Control of a Low-cost Mobile Robot with Omnidirectional Wheels Using Differentiable Physics
2022 International Conference on Robotics and Automation (ICRA)(2022)
摘要
We present a new data-driven technique for pre-dicting the motion of a low-cost omnidirectional mobile robot under the influence of motor torques and friction forces. Our method utilizes a novel differentiable physics engine for analytically computing the gradient of the deviation between predicted motion trajectories and real-world trajectories. This allows to automatically learn and fine-tune the unknown friction coefficients on-the-fly, by minimizing a carefully designed loss function using gradient descent. Experiments show that the predicted trajectories are in excellent agreement with their real-world counterparts. Our proposed approach is computationally superior to existing black-box optimization methods, requiring very few real-world samples for accurate trajectory prediction compared to physics-agnostic techniques, such as neural net-works. Experiments also demonstrate that the proposed method allows the robot to quickly adapt to changes in the terrain. Our proposed approach combines the data-efficiency of classical analytical models that are derived from first principles, with the flexibility of data-driven methods, which makes it appropriate for low-cost mobile robots. Project website: https://go.rutgers.edu/mqxn2x6h
更多查看译文
关键词
model identification,low-cost mobile robot,omnidirectional wheels,data-driven technique,low-cost omnidirectional mobile robot,motor torques,friction forces,differentiable physics engine,predicted motion trajectories,real-world trajectories,unknown friction coefficients on-the-fly,carefully designed loss function,gradient descent,black-box optimization methods,real-world samples,accurate trajectory prediction,physics-agnostic techniques,classical analytical models,neural networks
AI 理解论文
溯源树
样例
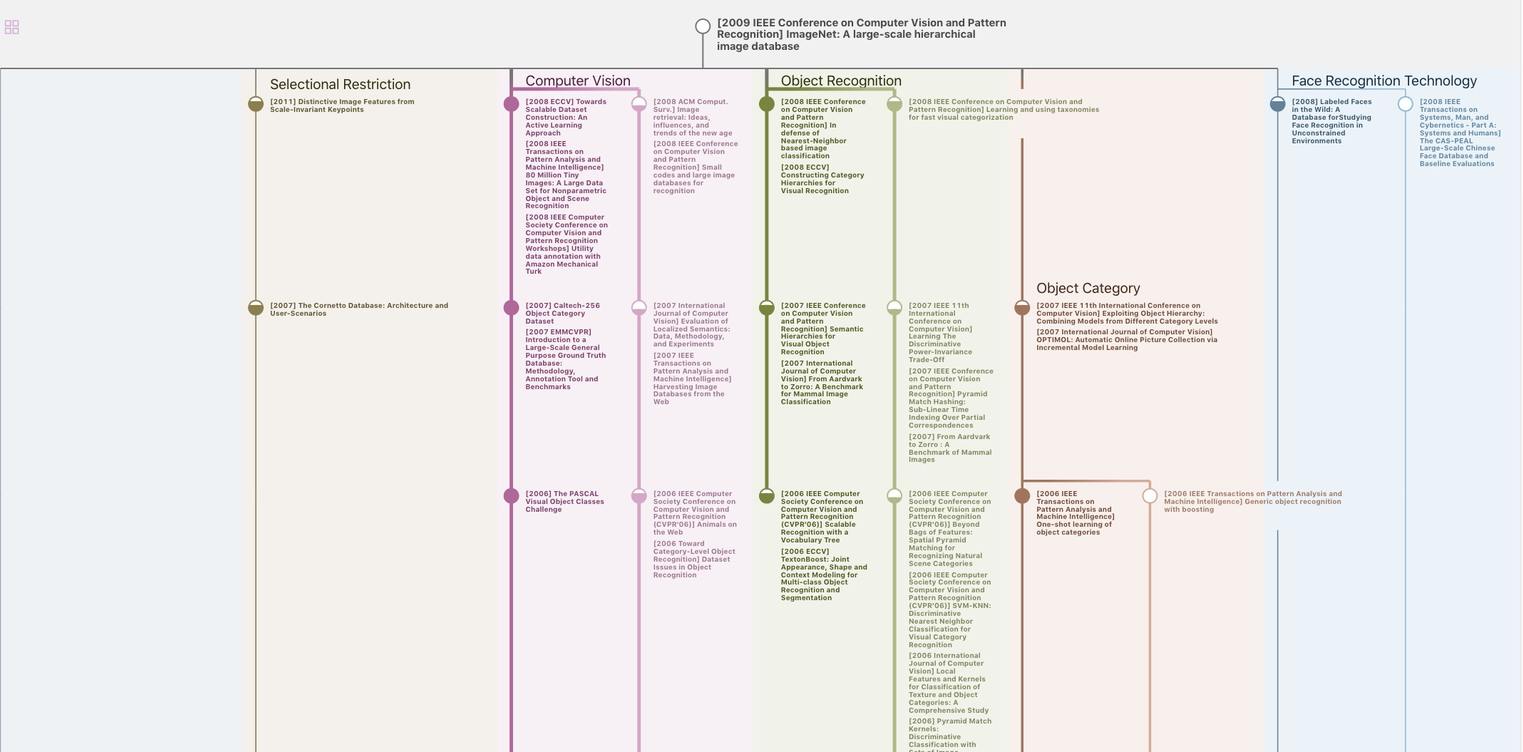
生成溯源树,研究论文发展脉络
Chat Paper
正在生成论文摘要