Visual Loop Closure Detection With Instance Segmentation and Image Inpainting in Dynamic Scenes Using Wearable Camera
IEEE SENSORS JOURNAL(2022)
摘要
Dynamic interference is an important factor that affects the accuracy of loop closure detection in visual SLAM. Traditional methods that remove the feature points in dynamic regions are prone to insufficient image features, which will decrease the reliability of loop closure detection. To overcome this problem, a novel loop closure detection approach based on image inpainting and feature selection is proposed. A dynamic object instance segmentation guided image inpainting network is utilized to segment and repair dynamic regions in scene images. To avoid the negative influence of image inpainting, an image quality evaluation network is used to confine the extraction of Superpoint features only in the properly inpainted patches. Therefore, valid Superpoint features only in the areas with high inpainting qualities are selected as the input of the Bag of Words model for loop-closure detection. We tested the proposed method on both the KITTI dataset and a wearable camera system. Experimental results indicate that the proposed method improves the accuracy of loop closure detection in dynamic scenes.
更多查看译文
关键词
Feature extraction, Semantics, Image segmentation, Visualization, Vehicle dynamics, Simultaneous localization and mapping, Image quality, Loop closure detection, instance segmentation, image inpainting, image quality assessment, visual SLAM
AI 理解论文
溯源树
样例
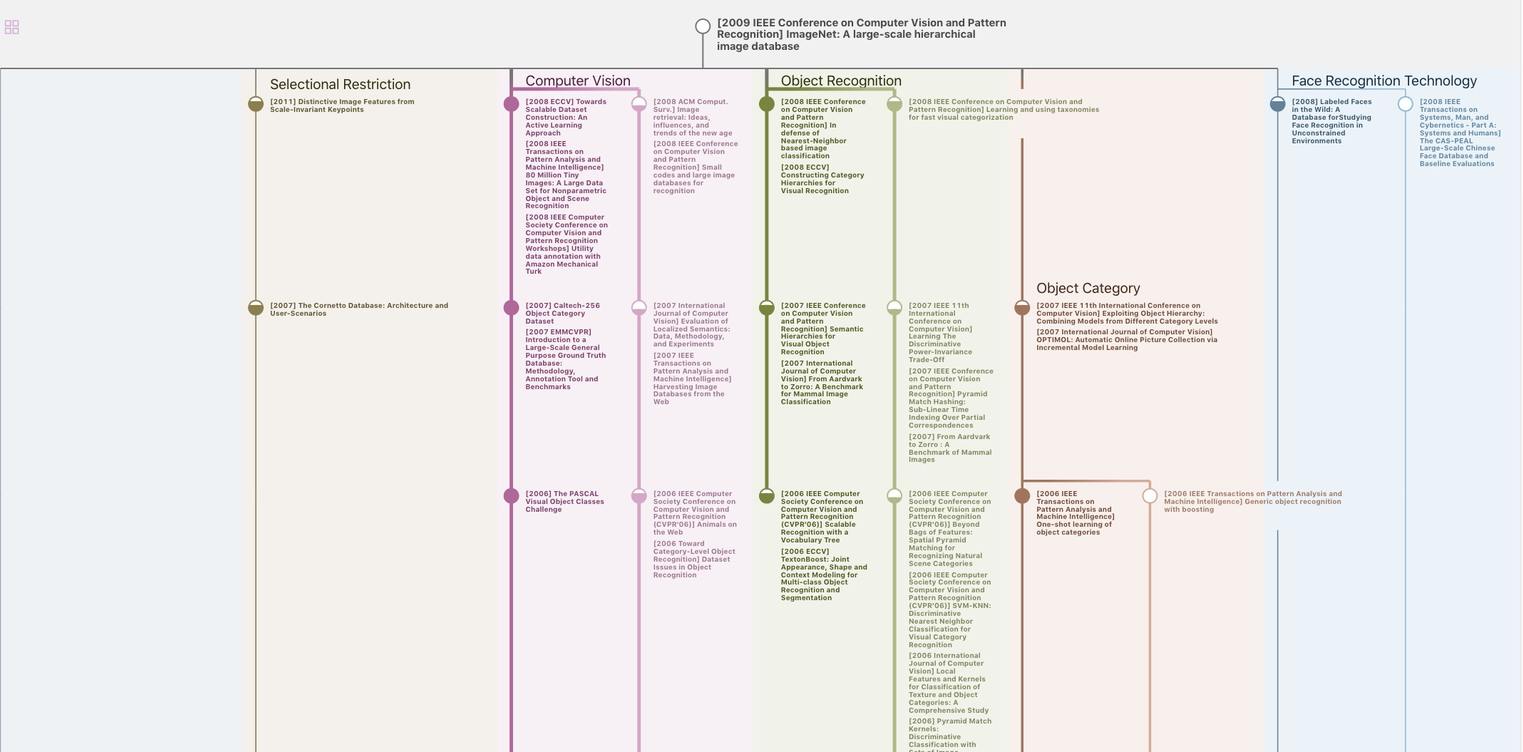
生成溯源树,研究论文发展脉络
Chat Paper
正在生成论文摘要