Unsupervised Model-based Pre-training for Data-efficient Control from Pixels
arxiv(2022)
摘要
Controlling artificial agents from visual sensory data is an arduous task. Reinforcement learning (RL) algorithms can succeed in this but require large amounts of interactions between the agent and the environment. To alleviate the issue, unsupervised RL proposes to employ self-supervised interaction and learning, for adapting faster to future tasks. Yet, whether current unsupervised strategies improve generalization capabilities is still unclear, especially in visual control settings. In this work, we design an effective unsupervised RL strategy for data-efficient visual control. First, we show that world models pre-trained with data collected using unsupervised RL can facilitate adaptation for future tasks. Then, we analyze several design choices to adapt efficiently, effectively reusing the agents' pre-trained components, and learning and planning in imagination, with our hybrid planner, which we dub Dyna-MPC. By combining the findings of a large-scale empirical study, we establish an approach that strongly improves performance on the Unsupervised RL Benchmark, requiring 20$\times$ less data to match the performance of supervised methods. The approach also demonstrates robust performance on the Real-Word RL benchmark, hinting that the approach generalizes to noisy environments.
更多查看译文
关键词
unsupervised reinforcement learning,world models,planning
AI 理解论文
溯源树
样例
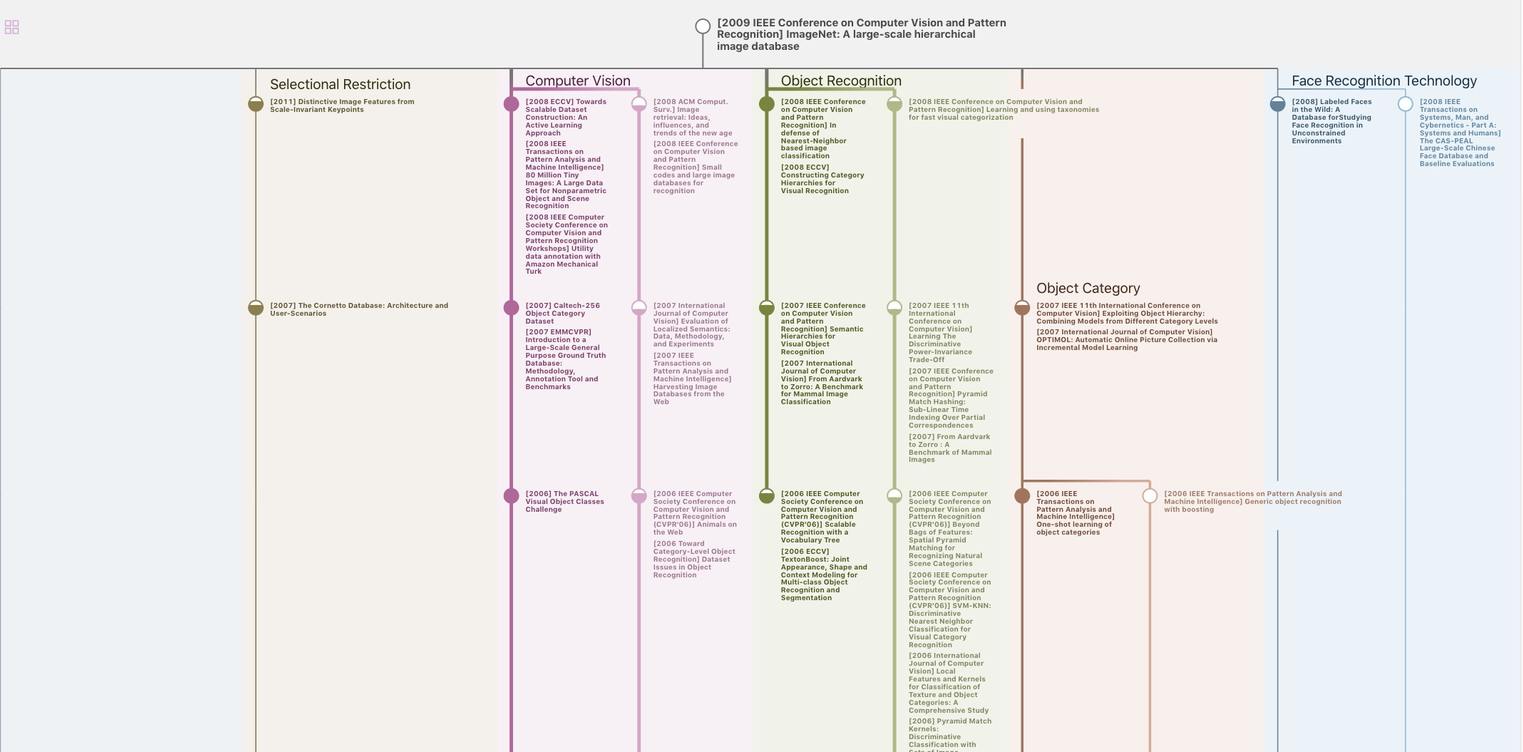
生成溯源树,研究论文发展脉络
Chat Paper
正在生成论文摘要