Reinforcement Learning for Spectrum Prediction and EE Maximization in D2D Communication
2022 IEEE International Conference on Signal Processing and Communications (SPCOM)(2022)
摘要
This paper proposes a reinforcement learning (RL) based Q-learning to address the issues of joint spectrum prediction (SP) and device-to-device (D2D) data communication in cognitive radio (CR) framework. An optimization problem is formulated that addresses energy efficiency (EE) maximization of D2D communications under the constraints of its total transmit power and a certain data transmission rate while meeting an interference threshold and cooperation rate in primary user (PU) transmission. The high accuracy in SP offers reward as an improvement on EE while a compulsion of meeting an interference threshold and a penalty on PU data transmission are made based on the relative degree of wrong prediction. A large set of simulation results shows that the proposed method offers 30% gain in EE while 20% reduction in data collision with PU over the existing works.
更多查看译文
关键词
cooperation rate,primary user transmission,interference threshold,PU data transmission,wrong prediction,data collision,reinforcement learning,spectrum prediction,EE maximization,D2D Communication,device-to-device data communication,cognitive radio,optimization problem,addresses energy efficiency maximization,data transmission rate
AI 理解论文
溯源树
样例
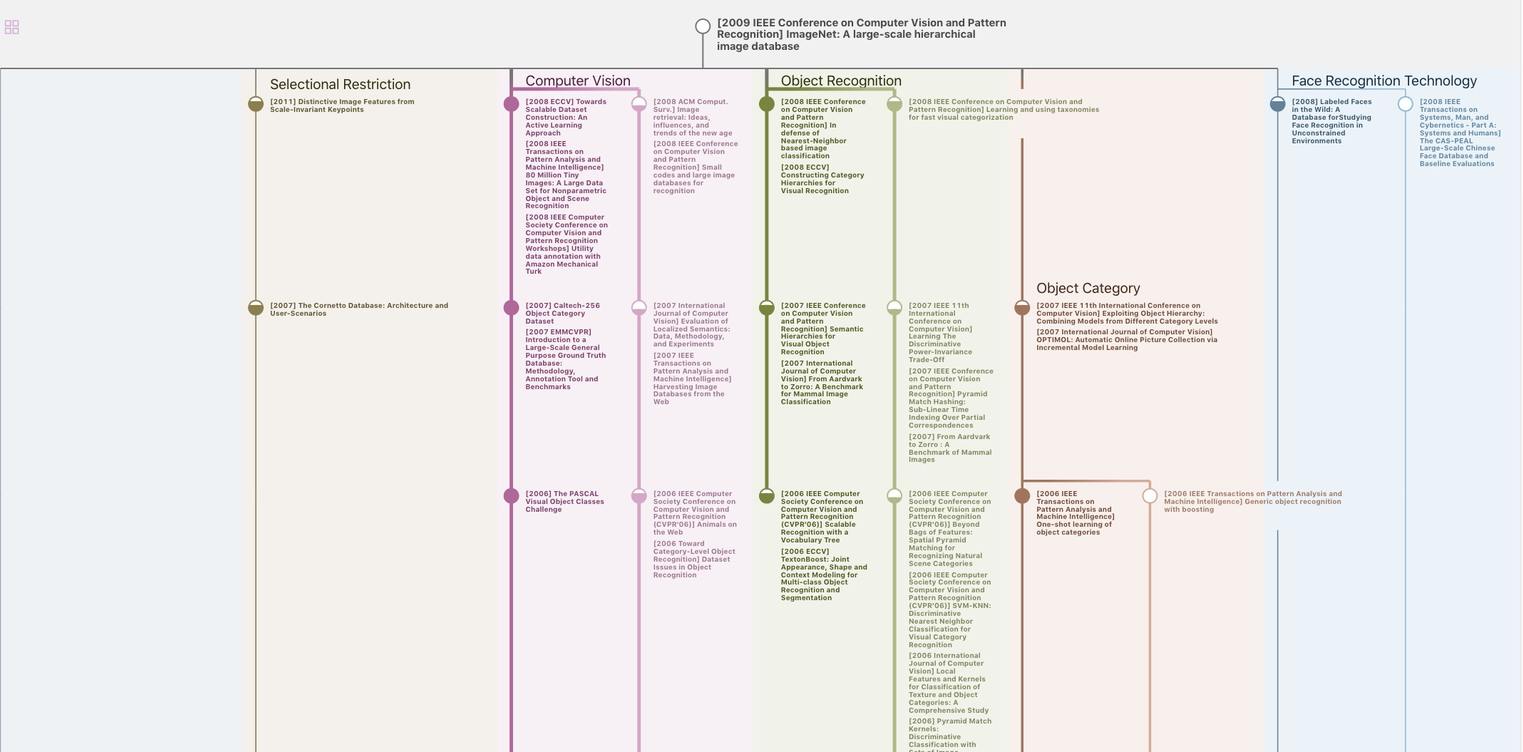
生成溯源树,研究论文发展脉络
Chat Paper
正在生成论文摘要