Privacy-Preserving and Robust Federated Deep Metric Learning
2022 IEEE/ACM 30th International Symposium on Quality of Service (IWQoS)(2022)
摘要
Federated learning, in contrast to traditional learning paradigms, has demonstrated its unique advantages in providing intelligence at the edge. However, existing federated learning approaches focus on the end-to-end classification tasks requiring a simple collaboration procedure where each participant can perform its local training independently. Unfortunately, there are still many tasks relying on learning the distinguishable feature metrics with respect to all the data, which is a different collaboration procedure across training participants. For example, the model for people identification has to ensure the feature representing a person is dissimilar to those representing others. To enable such federated learning for deep metrics (a.k.a federated deep metric learning) is challenging due to the data privacy and procedure robustness issues. With the consideration of these two challenges, this work proposes a novel computing framework for federated deep metric learning. This framework leverages the system-algorithm co-design to address privacy concerns via the Trusted Execution Environment (SGX enclave) and Differential Privacy mechanism. It also introduces a large-scale federated protocol which can robustly and efficiently deal with practical factors like the network fluctuation. We implement and evaluate our computing framework with two settings. One is a real-world implementation with a large number of mobile devices, while the other one is in our controllable environment for conducting experiments in various tasks. Our evaluation results show that our computing framework is able to train federated deep metric learning models with excellent scalability, data privacy preserving, and considerable accuracy even in exception conditions.
更多查看译文
关键词
robust federated deep metric learning,end-to-end classification tasks,simple collaboration procedure,distinguishable feature metrics,deep metrics,large-scale federated protocol,data privacy,system-algorithm co-design,trusted execution environment,SGX enclave,differential privacy mechanism,mobile devices
AI 理解论文
溯源树
样例
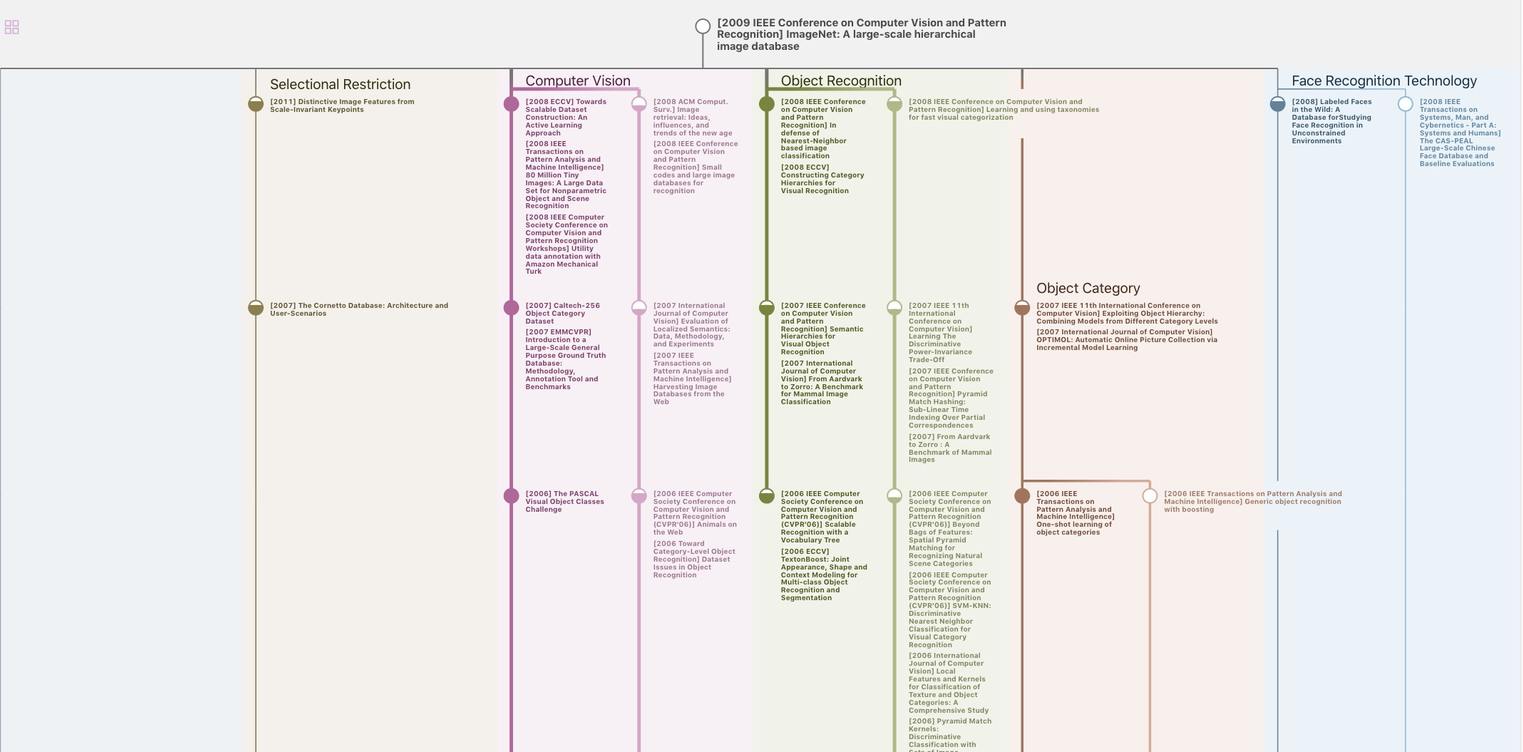
生成溯源树,研究论文发展脉络
Chat Paper
正在生成论文摘要