TabLLM: Few-shot Classification of Tabular Data with Large Language Models
arxiv(2023)
摘要
We study the application of large language models to zero-shot and few-shot classification of tabular data. We prompt the large language model with a serialization of the tabular data to a natural-language string, together with a short description of the classification problem. In the few-shot setting, we fine-tune the large language model using some labeled examples. We evaluate several serialization methods including templates, table-to-text models, and large language models. Despite its simplicity, we find that this technique outperforms prior deep-learning-based tabular classification methods on several benchmark datasets. In most cases, even zero-shot classification obtains non-trivial performance, illustrating the method's ability to exploit prior knowledge encoded in large language models. Unlike many deep learning methods for tabular datasets, this approach is also competitive with strong traditional baselines like gradient-boosted trees, especially in the very-few-shot setting.
更多查看译文
关键词
classification,large language
AI 理解论文
溯源树
样例
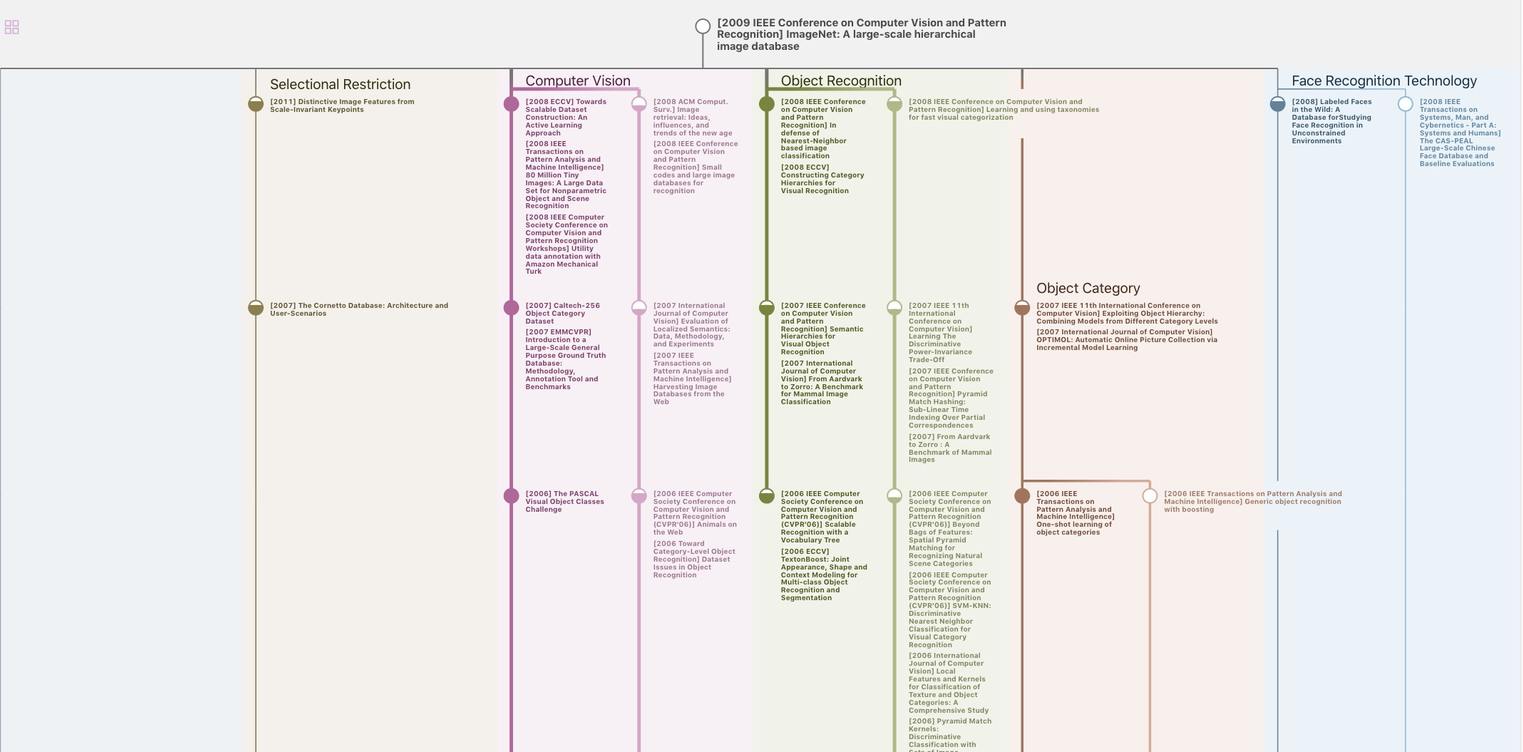
生成溯源树,研究论文发展脉络
Chat Paper
正在生成论文摘要