DeepTrace: Learning to Optimize Contact Tracing in Epidemic Networks with Graph Neural Networks
arxiv(2023)
摘要
The goal of digital contact tracing is to diminish the spread of an epidemic or pandemic by detecting and mitigating public health emergencies using digital technologies. Since the start of the COVID-$19$ pandemic, a wide variety of mobile digital apps have been deployed to identify people exposed to the SARS-CoV-2 coronavirus and to stop onward transmission. Tracing sources of spreading (i.e., backward contact tracing), as has been used in Japan and Australia, has proven crucial as going backwards can pick up infections that might otherwise be missed at superspreading events. How should robust backward contact tracing automated by mobile computing and network analytics be designed? In this paper, we formulate the forward and backward contact tracing problem for epidemic source inference as maximum-likelihood (ML) estimation subject to subgraph sampling. Besides its restricted case (inspired by the seminal work of Zaman and Shah in 2011) when the full infection topology is known, the general problem is more challenging due to its sheer combinatorial complexity, problem scale and the fact that the full infection topology is rarely accurately known. We propose a Graph Neural Network (GNN) framework, named DeepTrace, to compute the ML estimator by leveraging the likelihood structure to configure the training set with topological features of smaller epidemic networks as training sets. We demonstrate that the performance of our GNN approach improves over prior heuristics in the literature and serves as a basis to design robust contact tracing analytics to combat pandemics.
更多查看译文
关键词
epidemic networks
AI 理解论文
溯源树
样例
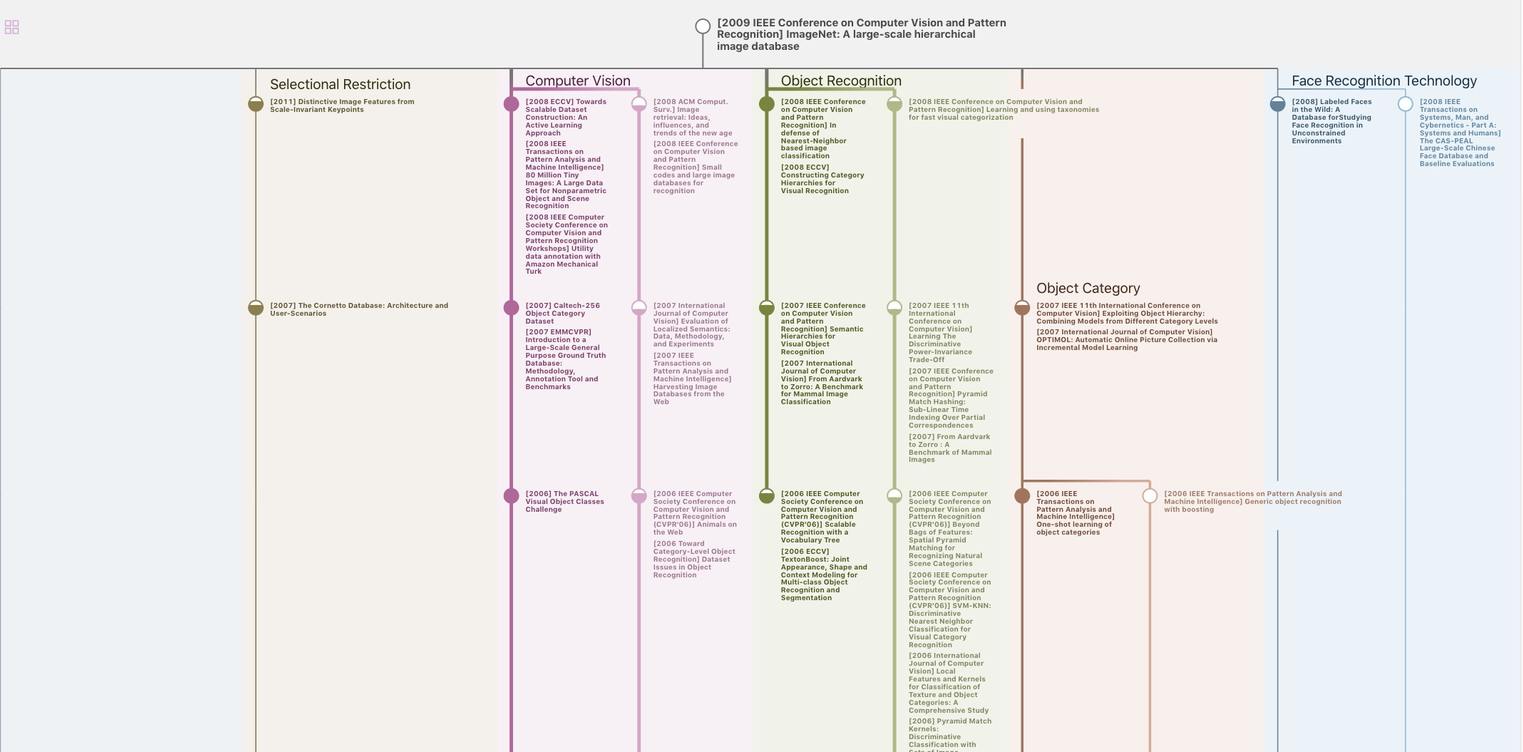
生成溯源树,研究论文发展脉络
Chat Paper
正在生成论文摘要