MOB-FL: Mobility-Aware Federated Learning for Intelligent Connected Vehicles
ICC 2023 - IEEE INTERNATIONAL CONFERENCE ON COMMUNICATIONS(2023)
摘要
Federated learning (FL) is a promising approach to enable the future Internet of vehicles consisting of intelligent connected vehicles (ICVs) with powerful sensing, computing and communication capabilities. We consider a base station (BS) coordinating nearby ICVs to train a neural network in a collaborative yet distributed manner, in order to limit data traffic and privacy leakage. However, due to the mobility of vehicles, the connections between the BS and ICVs are short-lived, which affects the resource utilization of ICVs, and thus, the convergence speed of the training process. In this paper, we propose an accelerated FL-ICV framework, by optimizing the duration of each training round and the number of local iterations, for better convergence performance of FL. We propose a mobility-aware optimization algorithm called MOB-FL, which aims at maximizing the resource utilization of ICVs under short-lived wireless connections, so as to increase the convergence speed. Simulation results based on the beam selection and the trajectory prediction tasks verify the effectiveness of the proposed solution.
更多查看译文
关键词
Intelligent connected vehicles,federated learning,mobility
AI 理解论文
溯源树
样例
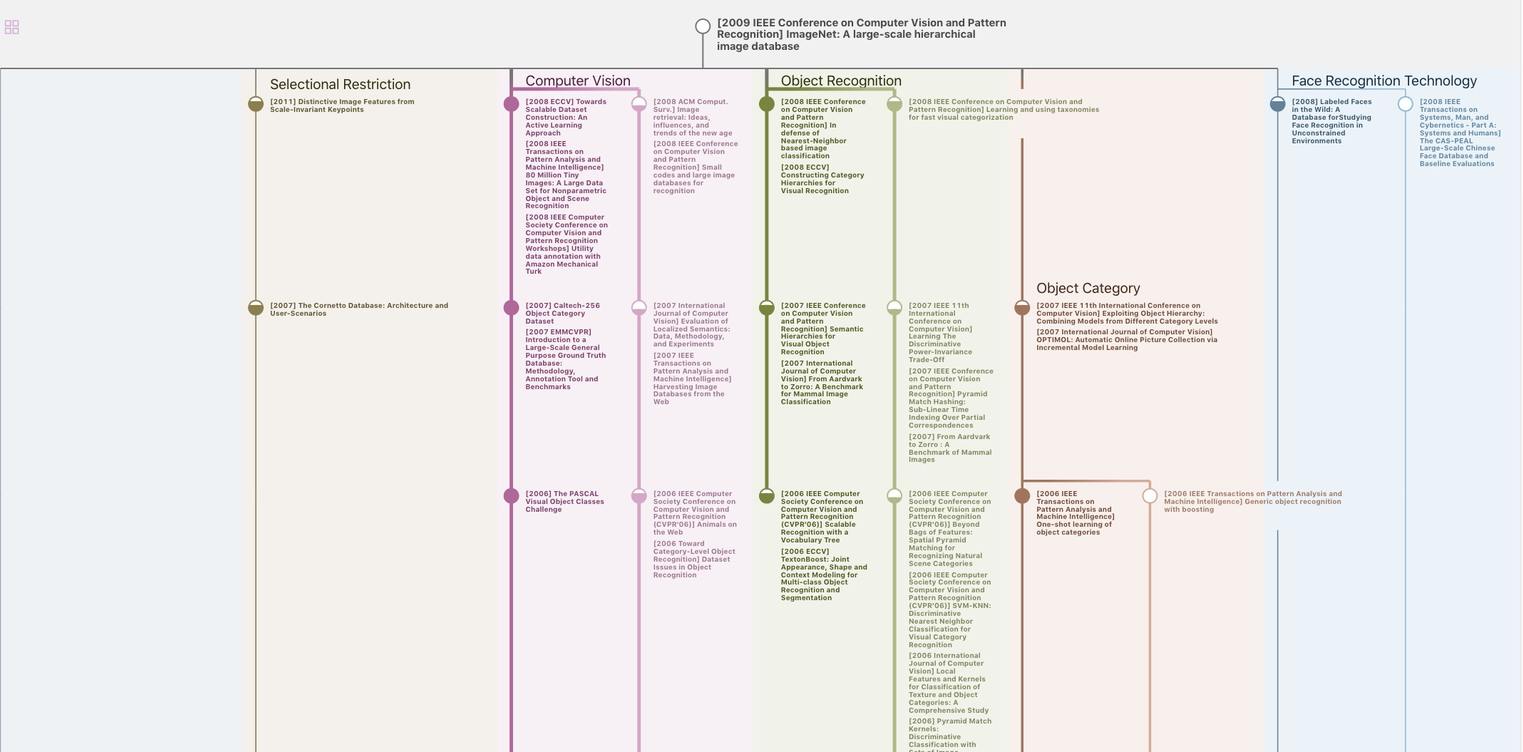
生成溯源树,研究论文发展脉络
Chat Paper
正在生成论文摘要