Effects of the Rotor Mechanical Offset Angle on the Electromagnetic Performance of Dual Stator Wound-Field Flux Switching Machines
2023 IEEE International Magnetic Conference - Short Papers (INTERMAG Short Papers)(2023)
Department of Electrical Engineering | Department of Electrical and Computer Engineering | Electrical and Information Engineering
Abstract
This study is concerned with the effect of the rotor mechanical offset (MO) angle on the electromagnetic performance of dual stator wound-field flux switching machines (DSWFFSMs). The focus is to find the optimum initial rotor position so that control of the machine can be facilitated. Using finite element analysis (FEA) and based on a cocktail of simulations, key electromagnetic performance indices such as average torque, torque ripple, power factor and efficiency are studied under dynamic on-load state, with the optimum MO angle established at 8°. The experimental test prototype has been developed and the test results are promised future extended paper version.
MoreTranslated text
Key words
Dual stator,electromagnetic performance,finite element analysis,mechanical offset,wound-field flux switching machine
求助PDF
上传PDF
View via Publisher
AI Read Science
AI Summary
AI Summary is the key point extracted automatically understanding the full text of the paper, including the background, methods, results, conclusions, icons and other key content, so that you can get the outline of the paper at a glance.
Example
Background
Key content
Introduction
Methods
Results
Related work
Fund
Key content
- Pretraining has recently greatly promoted the development of natural language processing (NLP)
- We show that M6 outperforms the baselines in multimodal downstream tasks, and the large M6 with 10 parameters can reach a better performance
- We propose a method called M6 that is able to process information of multiple modalities and perform both single-modal and cross-modal understanding and generation
- The model is scaled to large model with 10 billion parameters with sophisticated deployment, and the 10 -parameter M6-large is the largest pretrained model in Chinese
- Experimental results show that our proposed M6 outperforms the baseline in a number of downstream tasks concerning both single modality and multiple modalities We will continue the pretraining of extremely large models by increasing data to explore the limit of its performance
Upload PDF to Generate Summary
Must-Reading Tree
Example
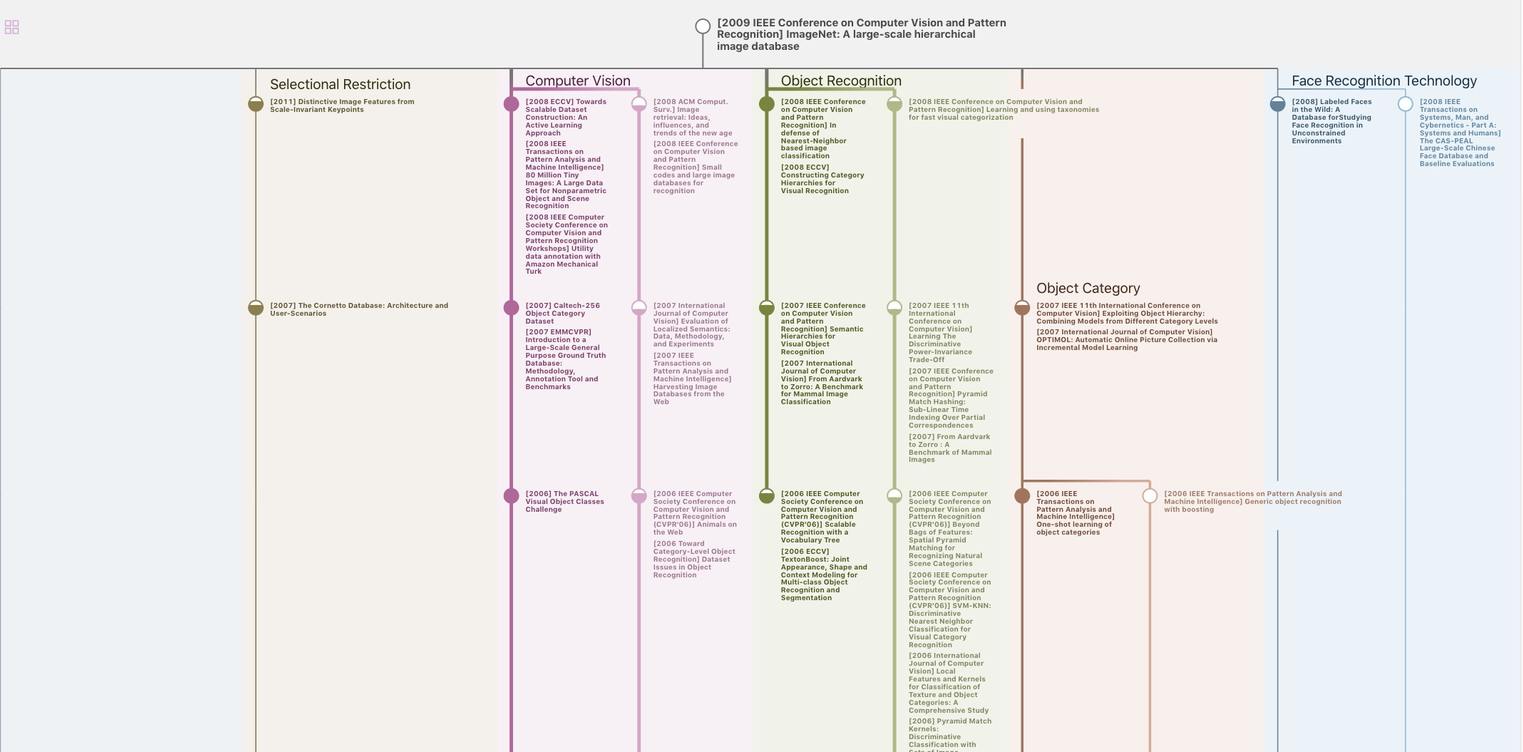
Generate MRT to find the research sequence of this paper
Data Disclaimer
The page data are from open Internet sources, cooperative publishers and automatic analysis results through AI technology. We do not make any commitments and guarantees for the validity, accuracy, correctness, reliability, completeness and timeliness of the page data. If you have any questions, please contact us by email: report@aminer.cn
Chat Paper
GPU is busy, summary generation fails
Rerequest