DRIP: Domain Refinement Iteration with Polytopes for Backward Reachability Analysis of Neural Feedback Loops
arxiv(2023)
摘要
Safety certification of data-driven control techniques remains a major open problem. This work investigates backward reachability as a framework for providing collision avoidance guarantees for systems controlled by neural network (NN) policies. Because NNs are typically not invertible, existing methods conservatively assume a domain over which to relax the NN, which causes loose over-approximations of the set of states that could lead the system into the obstacle (i.e., backprojection (BP) sets). To address this issue, we introduce DRIP, an algorithm with a refinement loop on the relaxation domain, which substantially tightens the BP set bounds. Furthermore, we introduce a formulation that enables directly obtaining closed-form representations of polytopes to bound the BP sets tighter than prior work, which required solving linear programs and using hyper-rectangles. Furthermore, this work extends the NN relaxation algorithm to handle polytope domains, which further tightens the bounds on BP sets. DRIP is demonstrated in numerical experiments on control systems, including a ground robot controlled by a learned NN obstacle avoidance policy.
更多查看译文
关键词
Artificial neural networks, Control systems, Reachability analysis, Safety, Collision avoidance, Heuristic algorithms, Aerospace electronics, Neural networks, data-driven control, safety verification, reachability analysis
AI 理解论文
溯源树
样例
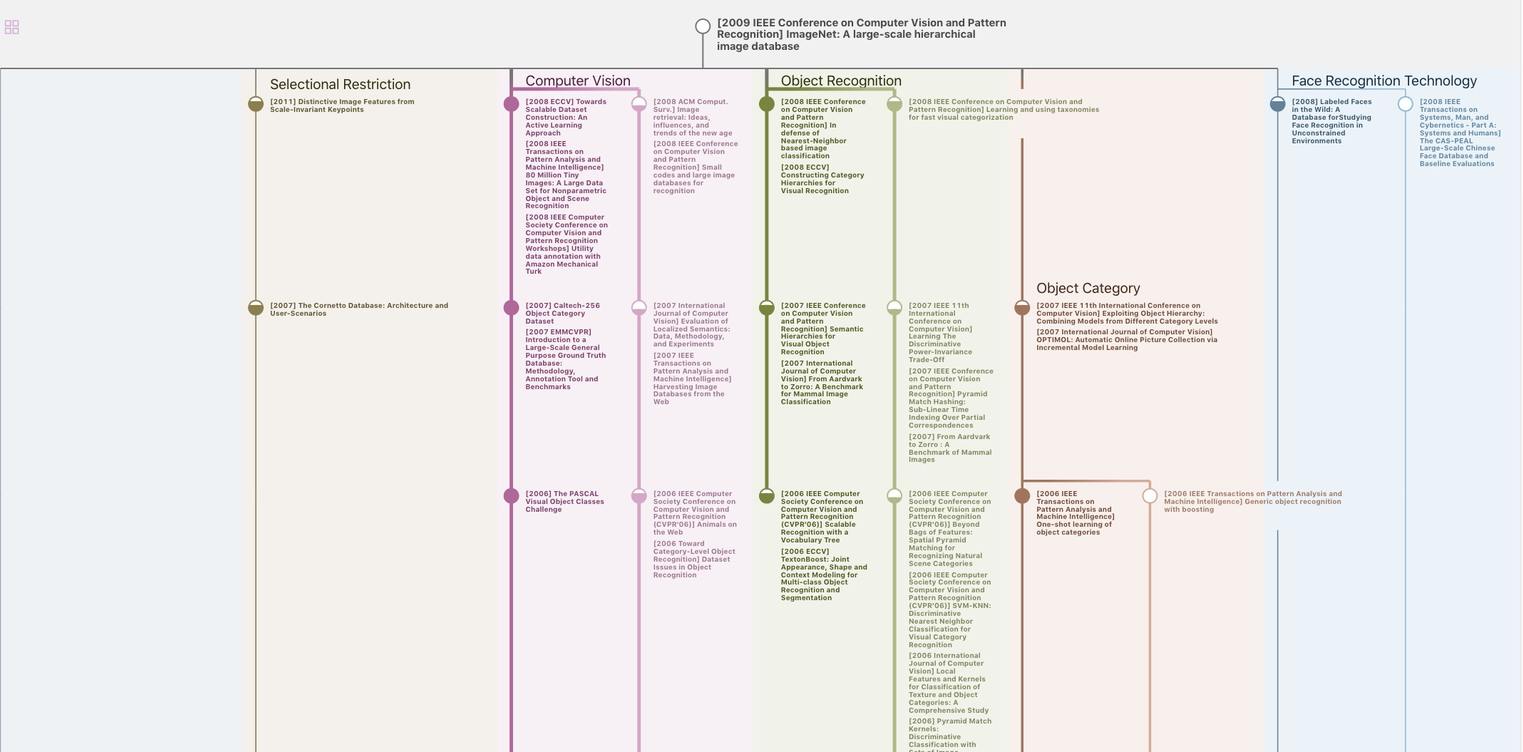
生成溯源树,研究论文发展脉络
Chat Paper
正在生成论文摘要