Risk-sensitive MPCs with Deep Distributional Inverse RL for Autonomous Driving
2022 IEEE/RSJ International Conference on Intelligent Robots and Systems (IROS)(2022)
摘要
In robot learning from demonstration (LfD), a visual representation of a cost function inferred from Inverse Reinforcement Learning (IRL) provides an intuitive tool for humans to quickly interpret the underlying objectives of the demonstration. The inferred cost function can be used by controllers, for example, Model Predictive Controllers (MPCs). In this work, we improve the recently developed IRL-MPC framework, by enhancing it in a risk-sensitive formulation to be more applicable for safety-critical applications like autonomous driving. Our risk-sensitive MPCs together with the distributional costmap demonstrate lower collision rates in the CARLA simulator for autonomous driving tasks compared to other learning-based baseline methods.
更多查看译文
关键词
autonomous driving tasks,deep distributional Inverse RL,distributional costmap,inferred cost function,intuitive tool,Inverse Reinforcement Learning,learning-based baseline methods,Model Predictive Controllers,recently developed IRL-MPC framework,risk-sensitive formulation,risk-sensitive MPCs,robot learning,safety-critical applications,underlying objectives,visual representation
AI 理解论文
溯源树
样例
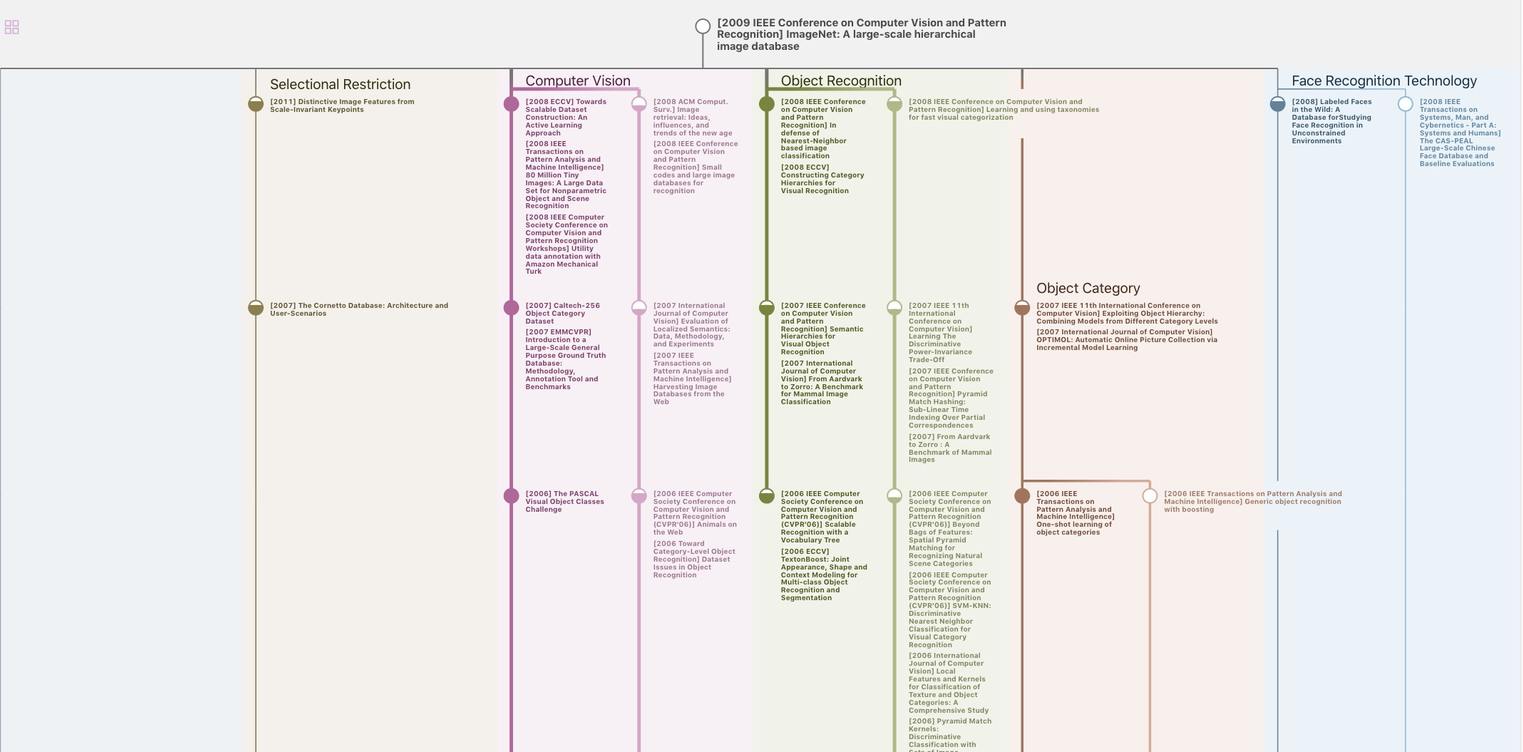
生成溯源树,研究论文发展脉络
Chat Paper
正在生成论文摘要