Spatial Semantic Learning for Travel Time Estimation.
Knowledge Science, Engineering and Management (KSEM)(2022)
摘要
Travel time estimation is a crucial and fundamental problem in Intelligent Transportation Systems (ITS), which benefits multiple downstream applications such as route planning, ride-sharing and taxi dispatching. Most previous work mainly focuses on the spatial space of a single form, which are incomplete representations of trajectories thus leading to inaccurate estimation results because multi-semantic context information has not been fully extracted and only one form of spatial representations are exploited. Additionally, many researchers use recurrent neural networks to extract temporal dependencies inside trajectories, which leads to uneven allocation of weight and brings about time-consuming iterative propagation problems. In this paper, we propose a Spatial Semantic learning model for Travel time Estimation (SSTE), to generate multi-view spatial representations for trajectories. This model represents trajectories in Euclidean space and non-Euclidean space simultaneously to learn different spatial representations from the latent road networks. Furthermore, a self-supervised segment embedding module named Seg2Vec is designed to produce spatial semantic segment embeddings. Seg2Vec exploits edge-level semantic representations of segments in non-Euclidean space, which takes road type and co-occurrence into consideration simultaneously. A sequence learning component with high computational efficiency is utilized to aggregate spatiotemporal relations, multi-semantic segment embeddings, and external properties of the query trajectory. Extensive experiments are conducted on two real-world trajectory datasets. The experimental results demonstrate that our SSTE significantly outperforms existing models.
更多查看译文
关键词
Sequence learning,Segment embedding,Spatial semantic learning,Travel time estimation,Intelligent transportation system
AI 理解论文
溯源树
样例
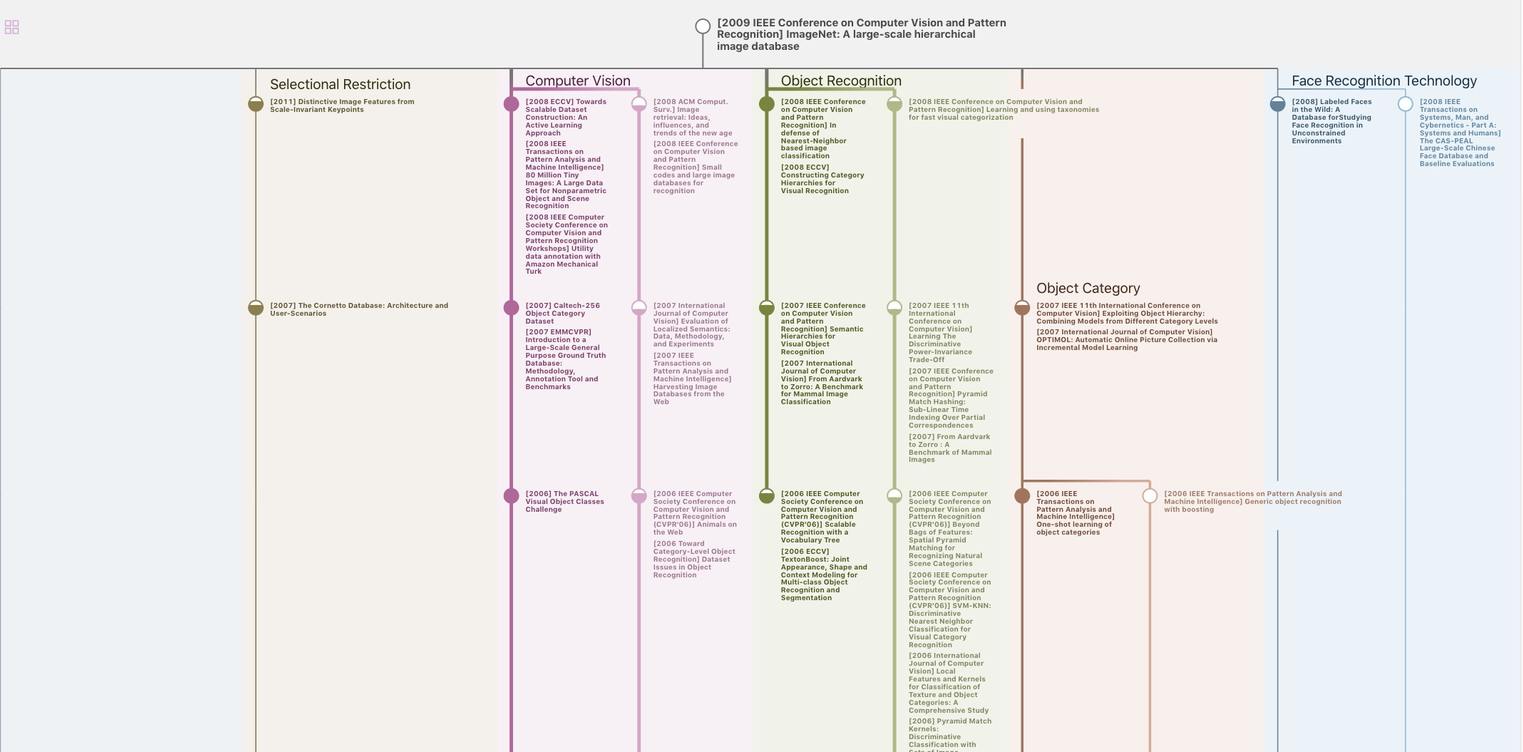
生成溯源树,研究论文发展脉络
Chat Paper
正在生成论文摘要