Few-shot graph learning with robust and energy-efficient memory-augmented graph neural network (MAGNN) based on homogeneous computing-in-memory
Symposium on VLSI Technology (VLSI Technology)(2022)
摘要
Learning graph structured data from limited examples on-the-fly is a key challenge to smart edge devices. Here, we present the first chip-level demonstration of few-shot graph learning which homogeneously implements both the controller and associative memory of a memory-augmented graph neural network using a 1T1R resistive random-access memory (RRAM). Leveraging the in-memory computing paradigm, we validated the high end-to-end accuracy of 78% (GPU baseline 80%) and robustness on node classification of CORA dataset, while achieved 70-fold reduction in latency and 60-fold reduction in energy consumption compared with conventional digital systems.
更多查看译文
关键词
1T1R resistive random-access memory,in-memory computing paradigm,high end-to-end accuracy,few-shot graph learning,robust energy-efficient memory,smart edge devices,chip-level demonstration,controller,associative memory,energy-efficient memory-augmented graph neural network,homogeneous computing-in-memory,graph structured data learning,RRAM,node classification,CORA dataset,energy consumption,conventional digital systems,MAGNN
AI 理解论文
溯源树
样例
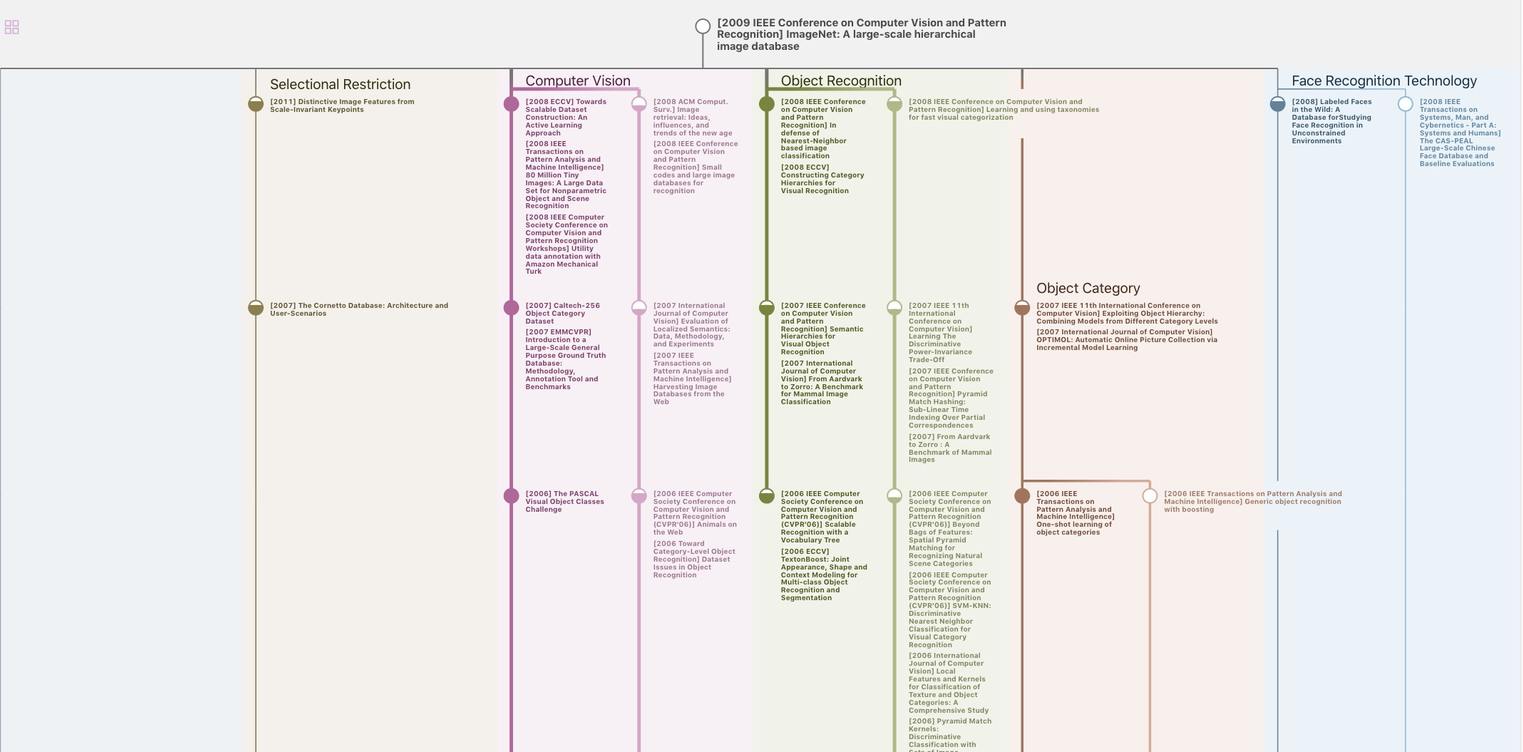
生成溯源树,研究论文发展脉络
Chat Paper
正在生成论文摘要