A graph attention fusion network for event-driven traffic speed prediction
Information Sciences(2023)
摘要
Accurate road traffic speed prediction has a critical role in intelligent transportation sys-tems and smart cities. This task is very challenging because of the complexity of road net-work structures, as well as various other unpredictable and ad hoc factors. Most existing traffic speed approaches handle external factors such as weather, holidays, and traffic acci-dents to enhance prediction accuracy. However, they ignore the impacts of social events or only simply embed them instead of learning the spatio-temporal representations. To address this issue, we design a novel framework named an event-aware graph attention fusion network (EGAF-Net) to effectively capture the spatiotemporal dependencies, includ-ing event impacts, in road networks based on an encoder-decoder architecture for traffic speed prediction. First, we utilize an ST-Speed attention block to model the spatial corre-lations among road segments and capture traffic speed changes. Second, we develop a spa-tial event embedding block exploiting a novel algorithm based on the node2vec approach, a new dynamic event graph constructor which produces learnable graphs utilized in graph convolution layers, and a temporal event attention block to learn the spatial and temporal representations of events. Finally, we propose a gated fusion mechanism to fuse the spatio-temporal correlations in road networks and the representations of events. Extensive exper-iments conducted based on the Q-Traffic, Q-Eastern-Traffic and Q-Western-Traffic datasets demonstrate the effectiveness of EGAF-Net over robust baselines.(c) 2022 Published by Elsevier Inc.
更多查看译文
关键词
Traffic speed prediction,Spatiotemporal correlations,Graph attention,Dynamic graph
AI 理解论文
溯源树
样例
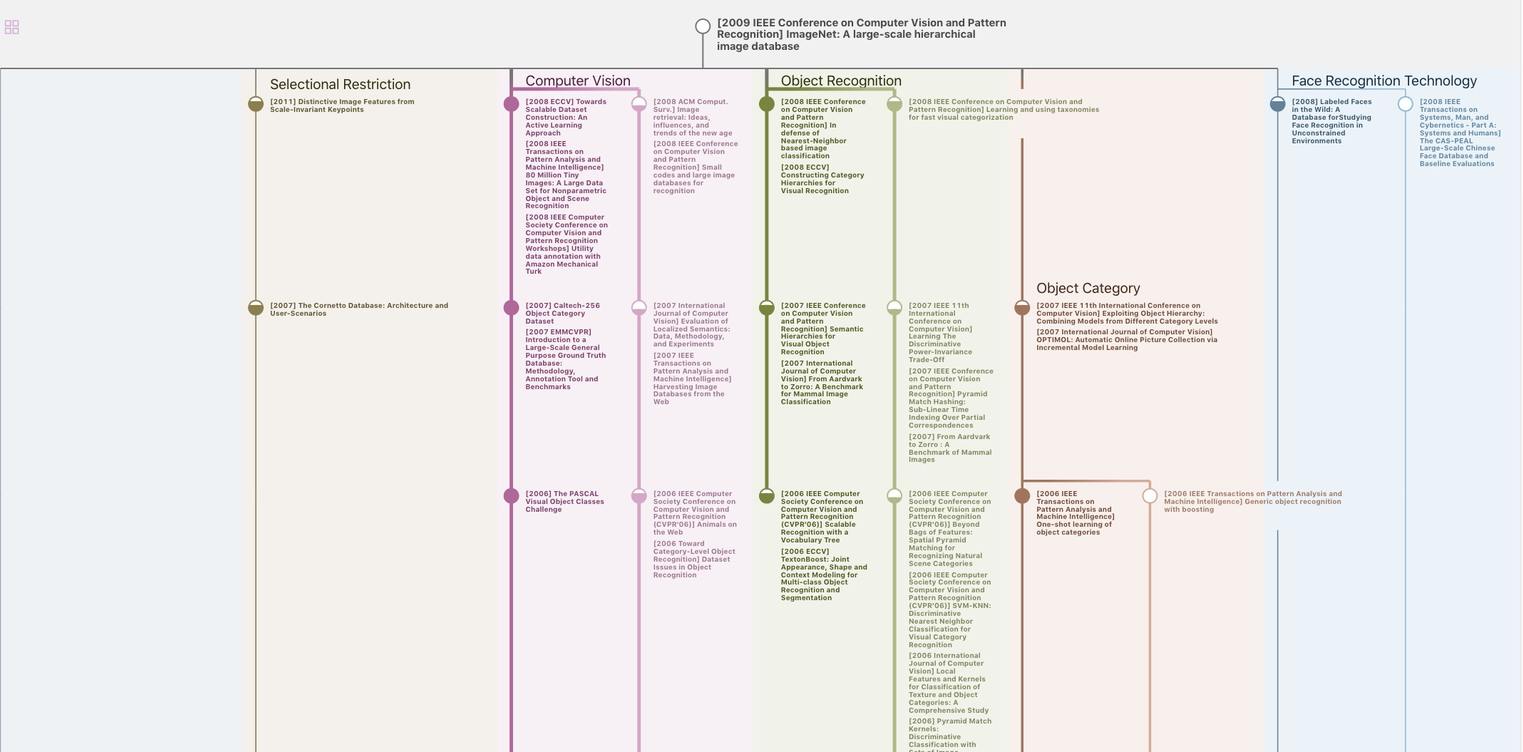
生成溯源树,研究论文发展脉络
Chat Paper
正在生成论文摘要