From English to More Languages: Parameter-Efficient Model Reprogramming for Cross-Lingual Speech Recognition
CoRR(2023)
摘要
In this work, we propose a new parameter-efficient learning framework based on neural model reprogramming for cross-lingual speech recognition, which can \textbf{re-purpose} well-trained English automatic speech recognition (ASR) models to recognize the other languages. We design different auxiliary neural architectures focusing on learnable pre-trained feature enhancement that, for the first time, empowers model reprogramming on ASR. Specifically, we investigate how to select trainable components (i.e., encoder) of a conformer-based RNN-Transducer, as a frozen pre-trained backbone. Experiments on a seven-language multilingual LibriSpeech speech (MLS) task show that model reprogramming only requires 4.2% (11M out of 270M) to 6.8% (45M out of 660M) of its original trainable parameters from a full ASR model to perform competitive results in a range of 11.9% to 8.1% WER averaged across different languages. In addition, we discover different setups to make large-scale pre-trained ASR succeed in both monolingual and multilingual speech recognition. Our methods outperform existing ASR tuning architectures and their extension with self-supervised losses (e.g., w2v-bert) in terms of lower WER and better training efficiency.
更多查看译文
关键词
Cross-lingual speech recognition,model reprogramming,pre-trained adaptation,and foundation speech models
AI 理解论文
溯源树
样例
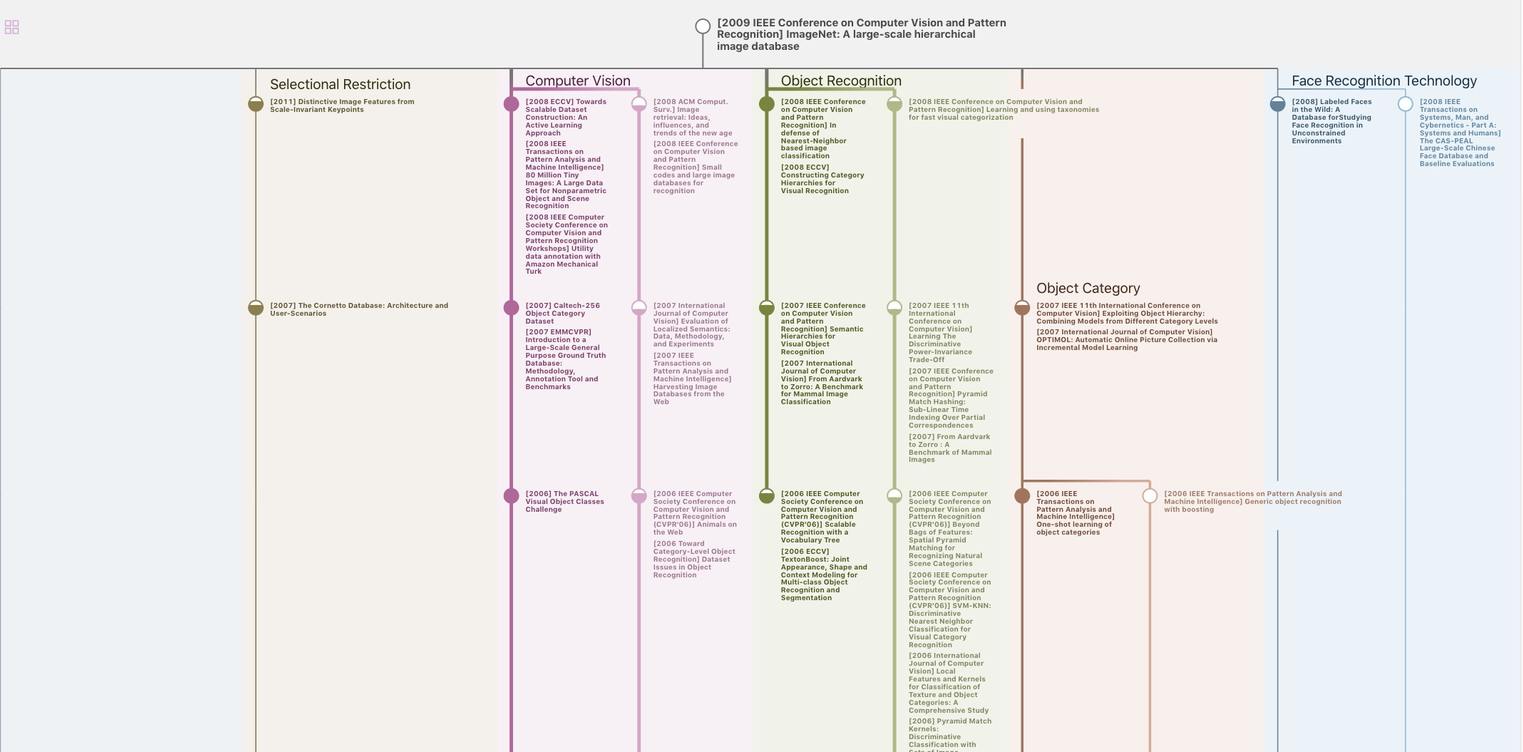
生成溯源树,研究论文发展脉络
Chat Paper
正在生成论文摘要