Federating Unlabeled Samples: A Semi-supervised Collaborative Framework for Whole Slide Image Analysis.
IDEAL(2022)
摘要
Over the last decades, deep learning-based algorithms have witnessed tremendous progress in the medical field to assist pathologists in clinical decisions and reduce their workload. For these models to reach their full potential, access to large and diverse datasets is essential, but collaborations between hospitals are highly limited by privacy-related regulations. At the same time, medical institutions do not always have specialized pathologists to diagnose biopsies and label local data. To address these limitations, federated learning gained traction to enable multi-institution model training without sharing sensitive patient data. However, this technique is still in its infancy when it comes to digital pathology applications, and does not consider institutions with unlabeled data in federations. In this paper, we introduce a novel semi-supervised federated learning approach that promotes multi-institutional training of deep learning models while integrating unlabeled collaborating data sources into the federated setup. The experimental results show a better performance for models trained under a federated setting with both labeled and unlabeled data. Optimally, this framework will also bring the promise of assisting clinical decisions in hospitals that do not have specialized pathologists.
更多查看译文
关键词
whole slide image analysis,unlabeled samples,semi-supervised
AI 理解论文
溯源树
样例
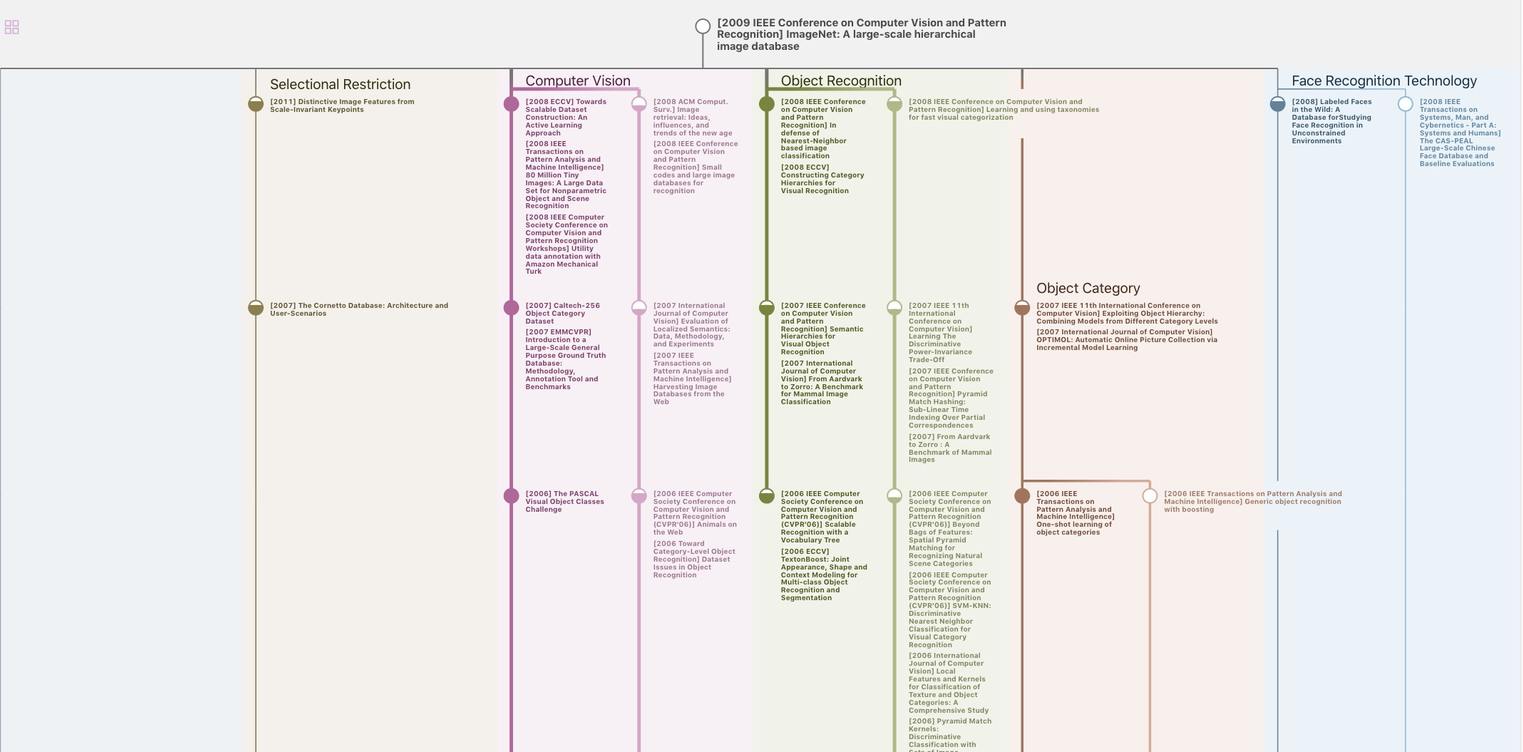
生成溯源树,研究论文发展脉络
Chat Paper
正在生成论文摘要