NetMoST: A network-based machine learning approach for subtyping schizophrenia using polygenic SNP allele biomarkers.
arxiv(2023)
摘要
Subtyping neuropsychiatric disorders like schizophrenia is essential for improving the diagnosis and treatment of complex diseases. Subtyping schizophrenia is challenging because it is polygenic and genetically heterogeneous, rendering the standard symptom-based diagnosis often unreliable and unrepeatable. We developed a novel network-based machine-learning approach, netMoST, to subtyping psychiatric disorders. NetMoST identifies polygenic risk SNP-allele modules from genome-wide genotyping data as polygenic haplotype biomarkers (PHBs) for disease subtyping. We applied netMoST to subtype a cohort of schizophrenia subjects into three distinct biotypes with differentiable genetic, neuroimaging and functional characteristics. The PHBs of the first biotype (36.9% of all patients) were related to neurodevelopment and cognition, the PHBs of the second biotype (28.4%) were enriched for neuroimmune functions, and the PHBs of the third biotype (34.7%) were associated with the transport of calcium ions and neurotransmitters. Neuroimaging patterns provided additional support to the new biotypes, with unique regional homogeneity (ReHo) patterns observed in the brains of each biotype compared with healthy controls. Our findings demonstrated netMoST's capability for uncovering novel biotypes of complex diseases such as schizophrenia. The results also showed the power of exploring polygenic allelic patterns that transcend the conventional GWAS approaches.
更多查看译文
关键词
polygenic snp allele biomarkers,schizophrenia,machine learning,network-based
AI 理解论文
溯源树
样例
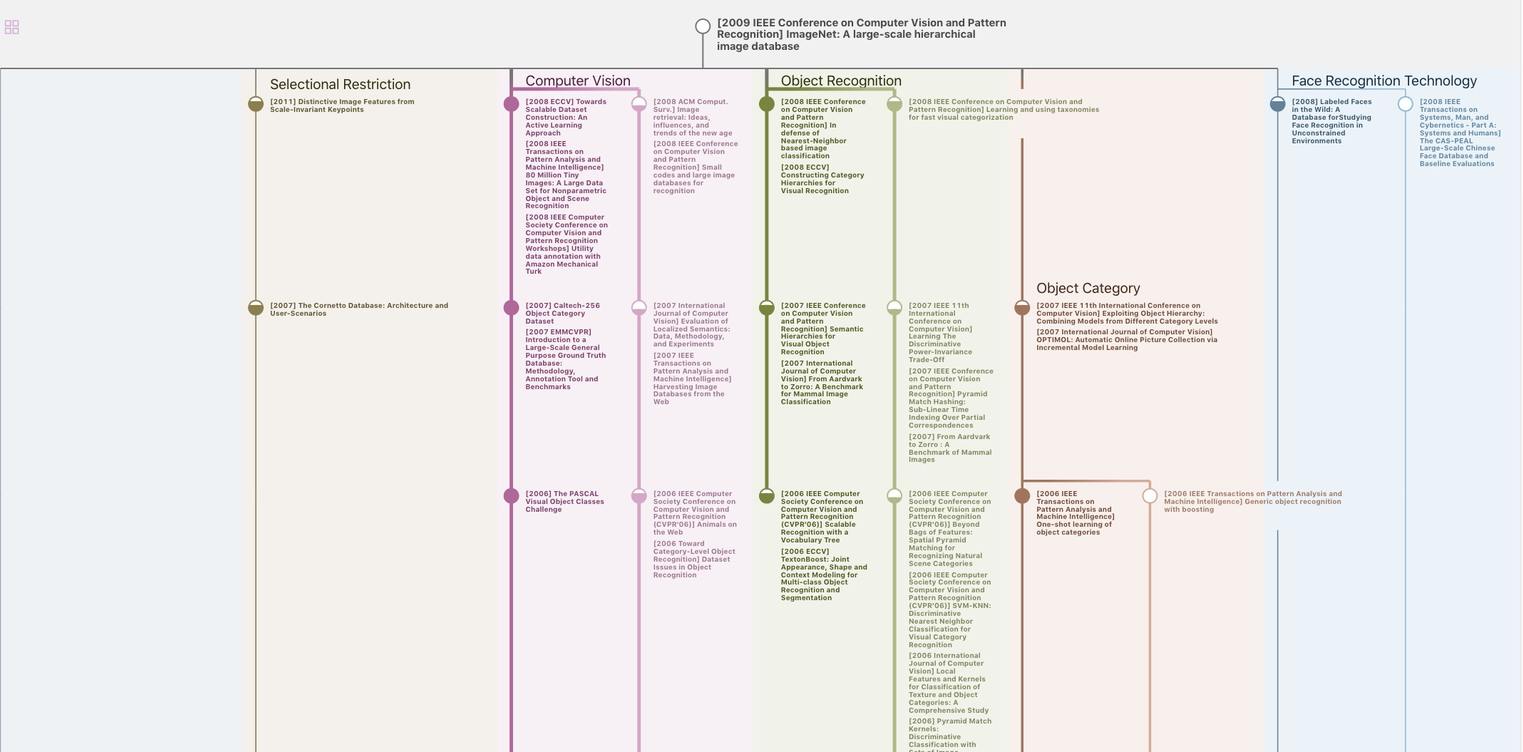
生成溯源树,研究论文发展脉络
Chat Paper
正在生成论文摘要