Progressive Compressed Auto-Encoder for Self-supervised Representation Learning
ICLR 2023(2023)
摘要
Masked Image Modeling (MIM) methods are driven by recovering all masked patches from visible ones. However, patches from the same image are highly correlated and it is redundant to reconstruct all the masked patches in MIM. This redundancy is neglected by existing methods and causes non-negligible overheads in computation and storage that do not necessarily benefit self-supervised learning. In this paper, we present a novel approach named Progressive Compressed AutoEncoder (PCAE) to address this problem by progressively compacting tokens and retaining the least necessary information for representation. In particular, we propose to mitigate the performance degradation caused by token reduction through exploiting the vision transformer to leak information from discarded tokens to the retained ones. Besides, we also propose the progressive discarding strategy to achieve a better trade-off between performance and efficiency. Identifying redundant tokens plays a key role in redundancy reduction. We resolve this issue using a simple yet effective criterion, i.e., we identify redundant tokens according to their similarity to the mean of token sequence. Thanks to the flexible strategy, PCAE can be employed for both pre-training and downstream fine-tuning and, consequently, reduces the computing overhead non-trivially throughout the training pipeline. Experiments show that PCAE achieves comparable performance while at most accelerates 1.9 times throughput compared with MAE for self-supervised learning, and accelerates 15\%-57\% throughput while the performance drop is within 0.6\% for downstream classification.
更多查看译文
关键词
MIM,Transformer,self-supervised learning
AI 理解论文
溯源树
样例
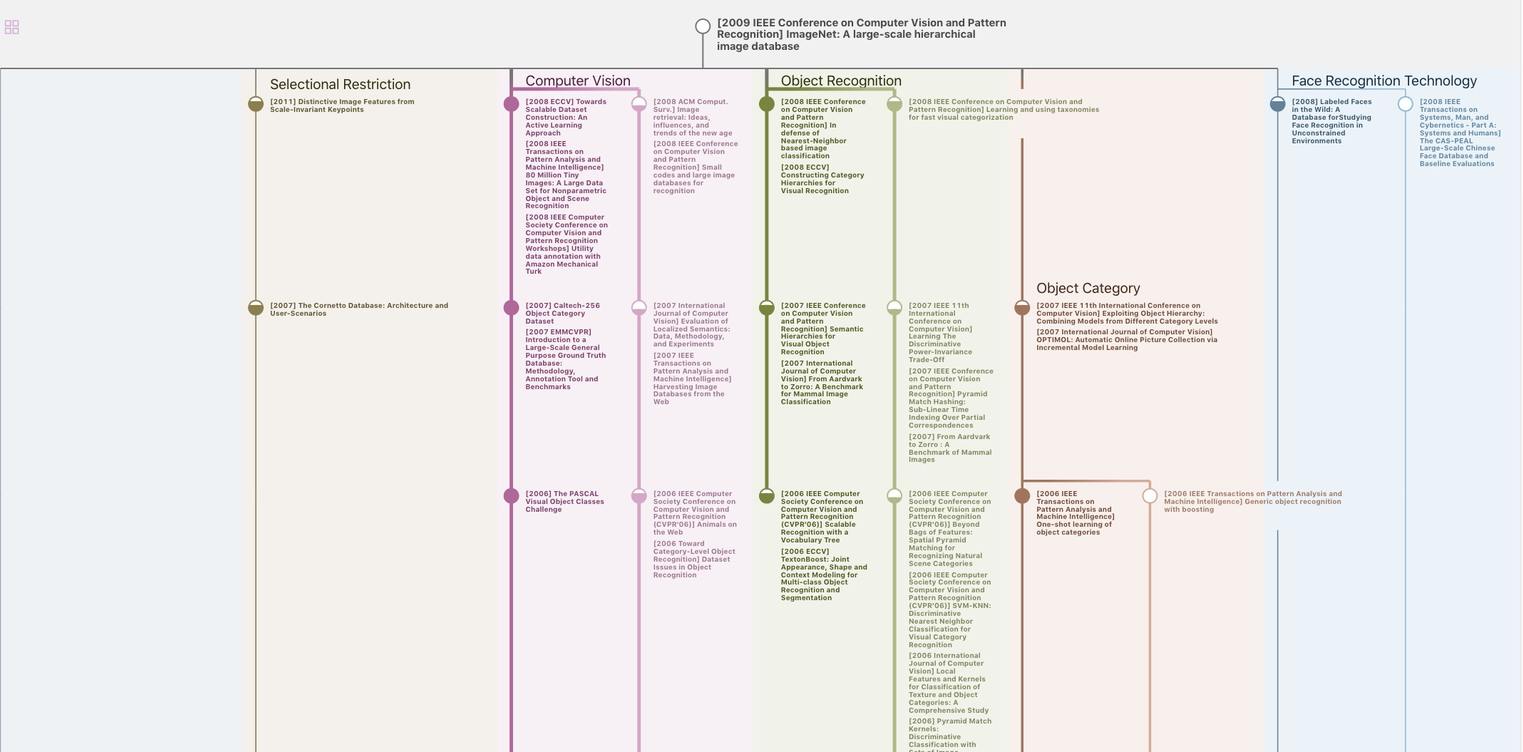
生成溯源树,研究论文发展脉络
Chat Paper
正在生成论文摘要