COREN: Multi-Modal Co-Occurrence Transformer Reasoning Network for Image-Text Retrieval
NEURAL PROCESSING LETTERS(2022)
摘要
Cross-modal image-text retrieval aims at retrieving the images according to the given query texts and vice versa, which is a challenging task due to the inherent heterogeneous gap between computer vision and natural language processing. Most previous methods mine the intra-modal interactions and inter-modal interactions independently, which may lead to a fragmented understanding of the visual-linguistic modalities. Different from them, in this paper, we address this challenge by proposing a unified multi-modal Co-Occurrence transformer Reasoning Network, dubbed as COREN, to comprehensively discover the semantic correlations of the two modalities. Specifically, we resort to a unified multi-modal transformer encoder to decompose the intra-modal and inter-modal co-occurrence relationships reasoning into a two-stage learning architecture. In the first learning stage, we utilize the multi-modal transformer as a shared siamese encoder for both visual and textual branch to reason the intra-modal co-occurrence relationships. In this way, we obtain modality-specific contextualized representations for each input image and text instance, and the model is equipped with the representation and reasoning ability of both visual and textual entities. In the second learning stage, we stack the visual and textual features together and jointly feed them into the same multi-modal transformer encoder to reason the inter-modal co-occurrence relationships between the two modalities. Additionally, we propose a novel Adaptive Similarity Aggregation (ASA) module to achieve a more accurate cross-modal similarity measurement based on the generated contextualized representations. The experimental results on benchmark datasets demonstrate the effectiveness and superiority of our proposed method.
更多查看译文
关键词
Cross-modal co-occurrence relationships,Transformer encoder,Image-text retrieval,Multi-modal analysis
AI 理解论文
溯源树
样例
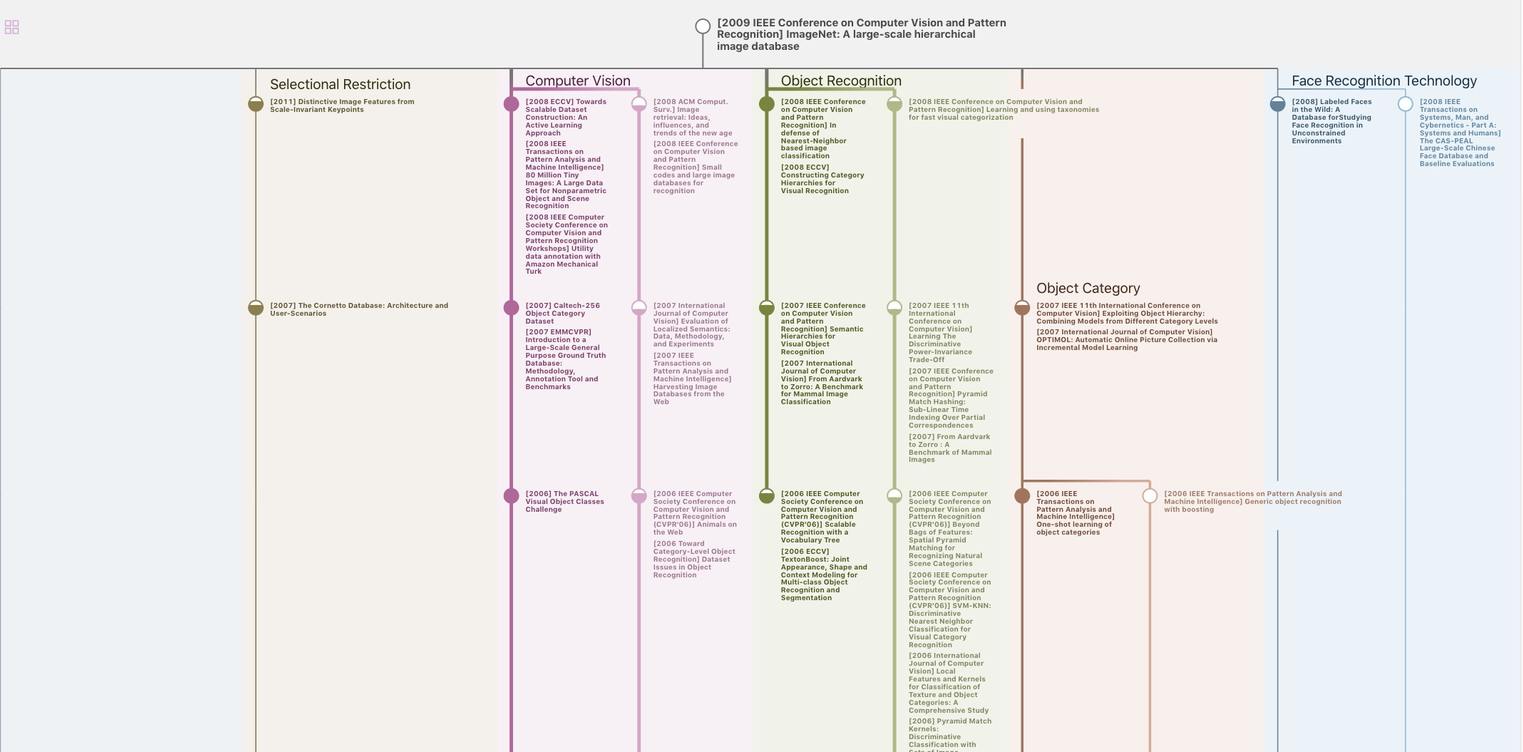
生成溯源树,研究论文发展脉络
Chat Paper
正在生成论文摘要