Deep Momentum Multi-Marginal Schr\"odinger Bridge
arxiv(2023)
摘要
Reconstructing population dynamics using only samples from distributions at coarse time intervals is a crucial challenge. Recent data-driven approaches such as flow-based models or Schr\"odinger Bridge models have demonstrated appealing performance, yet the inferred sample trajectories either fail to account for the underlying stochasticity or are unnecessarily rigid. In this article, we propose $\underline{D}$eep $\underline{M}$omentum Multi-Marginal $\underline{S}$chr\"odinger $\underline{B}$ridge(DMSB), a novel computational framework that learns the smooth measure-valued spline for stochastic systems without violating the position marginal constraints across time. We first extend the scalable mean matching objective used in the state space SB algorithm into the phase space. We next carefully craft a multi-constraint optimization training method based on Bregman Iteration that enables effective phase space means matching training for the high-dimensional dataset. We demonstrate that the resulting training algorithm significantly outperforms baselines on both synthetic datasets and a real-world single-cell RNA sequence dataset.
更多查看译文
关键词
bridge,multi-marginal
AI 理解论文
溯源树
样例
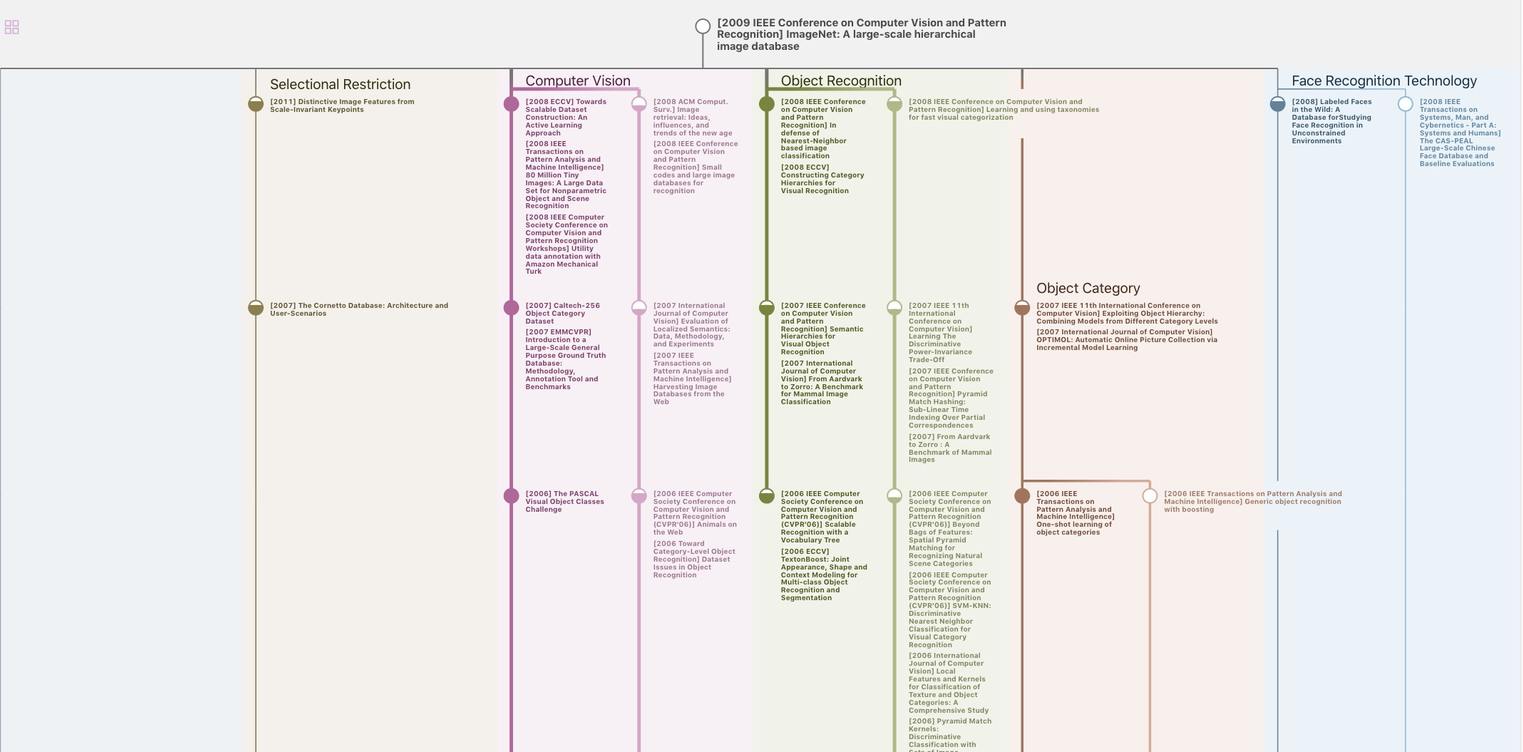
生成溯源树,研究论文发展脉络
Chat Paper
正在生成论文摘要