Generalized Deep 3D Shape Prior via Part-Discretized Diffusion Process
CVPR 2023(2023)
摘要
We develop a generalized 3D shape generation prior model, tailored for multiple 3D tasks including unconditional shape generation, point cloud completion, and cross-modality shape generation, etc. On one hand, to precisely capture local fine detailed shape information, a vector quantized variational autoencoder (VQ-VAE) is utilized to index local geometry from a compactly learned codebook based on a broad set of task training data. On the other hand, a discrete diffusion generator is introduced to model the inherent structural dependencies among different tokens. In the meantime, a multi-frequency fusion module (MFM) is developed to suppress high-frequency shape feature fluctuations, guided by multi-frequency contextual information. The above designs jointly equip our proposed 3D shape prior model with high-fidelity, diverse features as well as the capability of cross-modality alignment, and extensive experiments have demonstrated superior performances on various 3D shape generation tasks.
更多查看译文
关键词
3dqd,diffusion,part-discretized
AI 理解论文
溯源树
样例
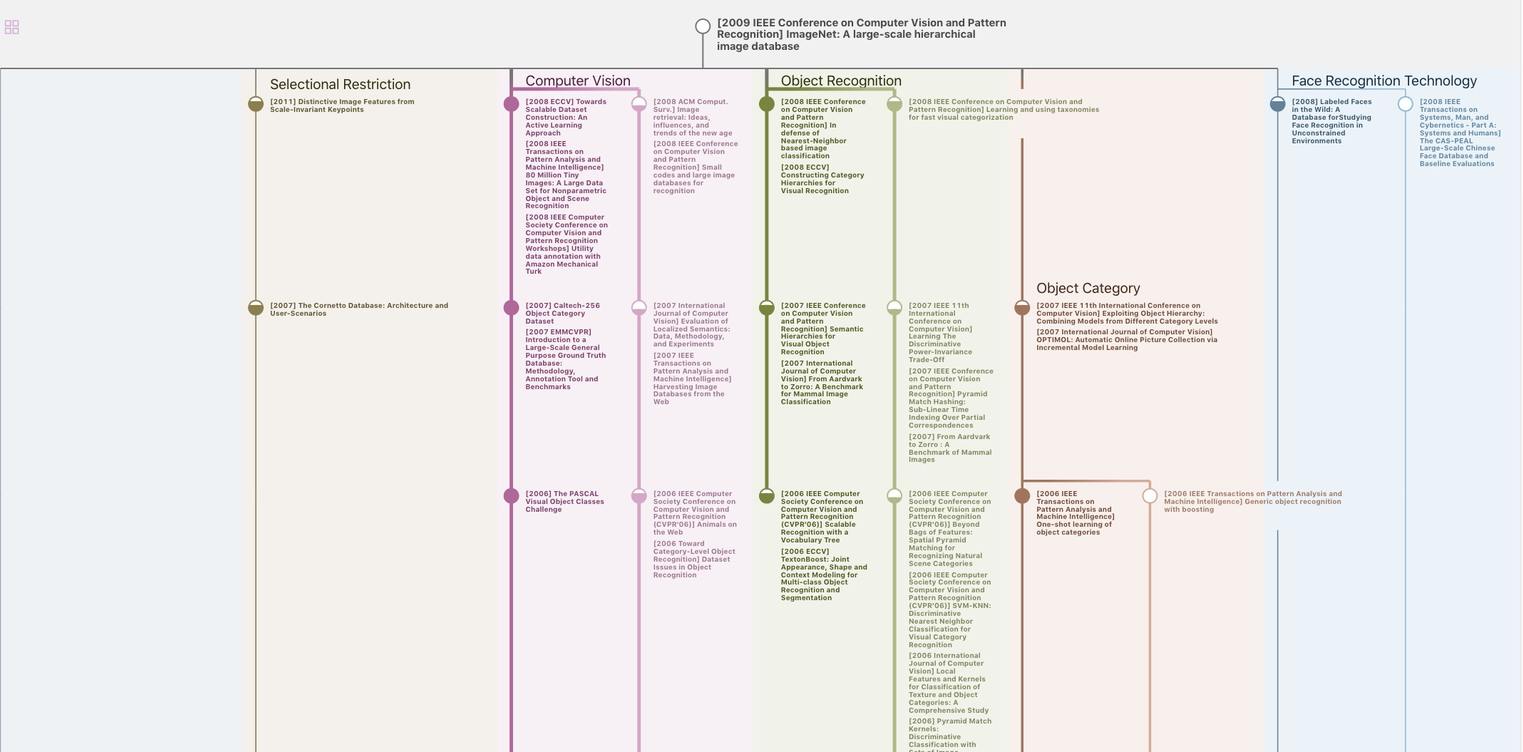
生成溯源树,研究论文发展脉络
Chat Paper
正在生成论文摘要