Latent transcriptional programs reveal histology-encoded tumor features spanning tissue origins
biorxiv(2023)
摘要
Precision medicine in cancer treatment depends on deciphering tumor phenotypes to reveal the underlying biological processes. Molecular profiles, including transcriptomics, provide an information-rich tumor view, but their high-dimensional features and assay costs can be prohibitive for clinical translation at scale. Recent studies have suggested jointly leveraging histology and genomics as a strategy for developing practical clinical biomarkers. Here, we use machine learning techniques to identify de novo latent transcriptional processes in squamous cell carcinomas (SCCs) and to accurately predict their activity levels directly from tumor histology images. In contrast to analyses focusing on pre-specified, individual genes or sample groups, our latent space analysis reveals sets of genes associated with both histologically detectable features and clinically relevant processes, including immune response, collagen remodeling, and fibrosis. The results demonstrate an approach for discovering clinically interpretable histological features that indicate complex, potentially treatment-informing biological processes.
### Competing Interest Statement
The authors have declared no competing interest.
更多查看译文
关键词
latent transcriptional programs,tumor features,tissue origins,histology-encoded
AI 理解论文
溯源树
样例
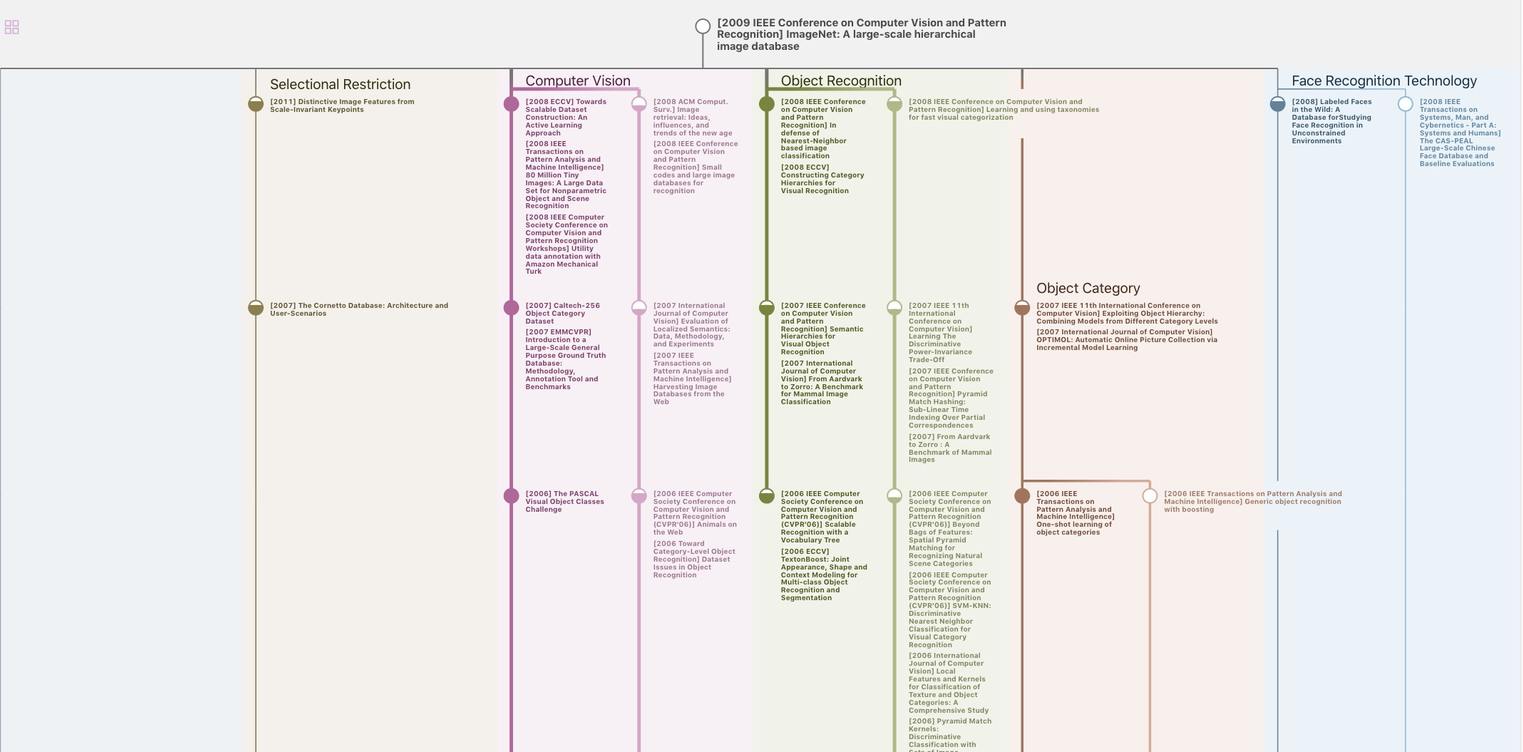
生成溯源树,研究论文发展脉络
Chat Paper
正在生成论文摘要