Individualized Tracking of Neurocognitive-State-Dependent Eye-Movement Features Using Mobile Devices
Proceedings of the ACM on Interactive, Mobile, Wearable and Ubiquitous Technologies(2023)
摘要
With current clinical techniques, it is difficult to assess a patient's neurodegenerative disease (e.g., Alzheimer's) state accurately and frequently. The most widely used tests are qualitative or only performed intermittently, motivating the need for quantitative, accurate, and unobtrusive metrics to track disease progression. Clinical studies have shown that saccade latency (an eye movement measure of reaction time) and error rate (the proportion of eye movements in the wrong direction) may be significantly affected by neurocognitive diseases. Nevertheless, how these features change over time as a disease progresses is underdeveloped due to the constrained recording setup. In this work, our goal is to first understand how these features change over time in healthy individuals. To do so, we used a mobile app to frequently and accurately measure these features outside of the clinical environment from 80 healthy participants. We analyzed their longitudinal characteristics and designed an individualized longitudinal model using a Gaussian process. With a system that can measure eye-movement features on a much finer timescale in a broader population, we acquired a better understanding of eye-movement features from healthy individuals and provided research directions in understanding whether eye-movement features can be used to track neurocognitive states.
更多查看译文
关键词
Gaussian Process,longitudinal model,mobile health monitoring,neurodegenerative diseases,saccade directional error rate,saccade latency
AI 理解论文
溯源树
样例
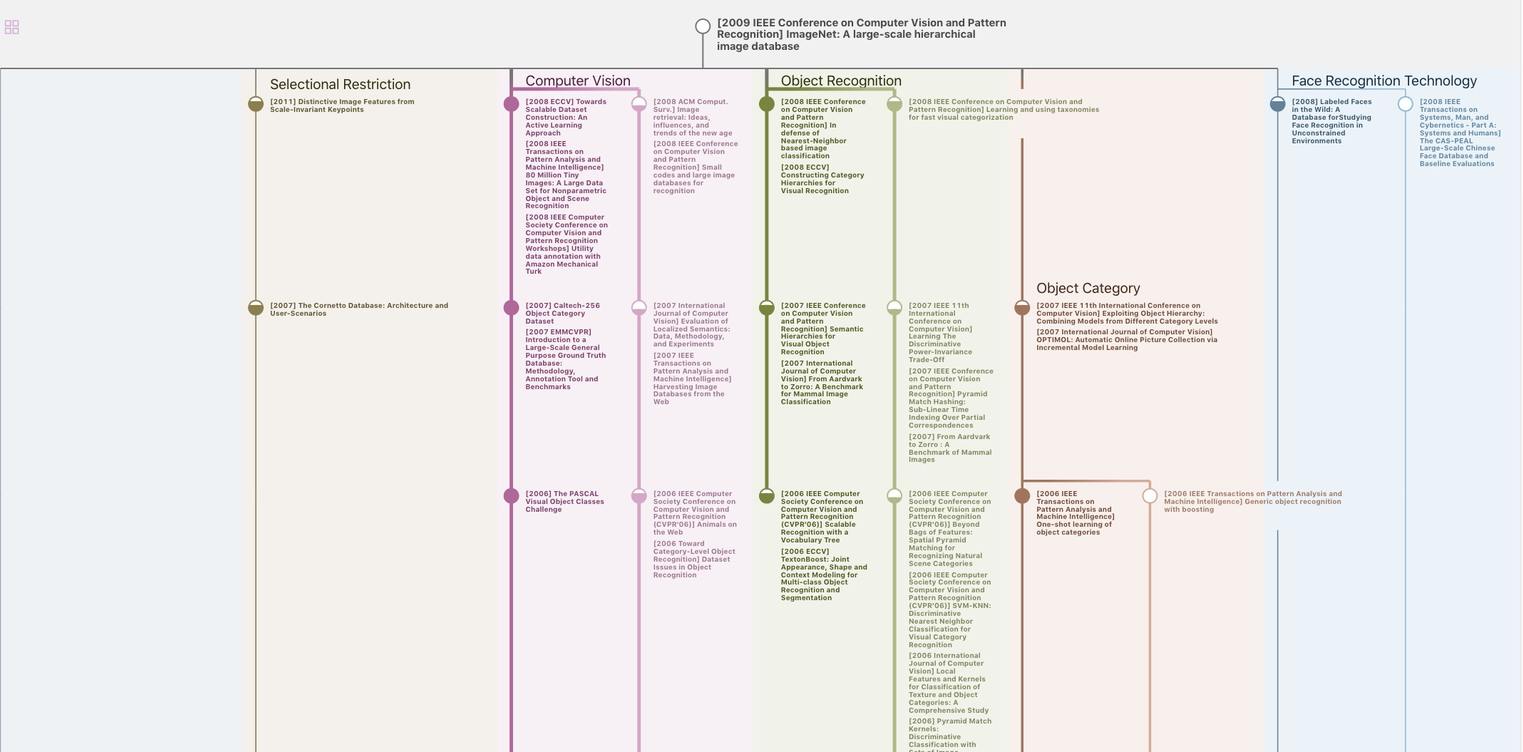
生成溯源树,研究论文发展脉络
Chat Paper
正在生成论文摘要