Approximating Relu Networks by Single-Spike Computation.
ICIP(2022)
摘要
Developing energy-saving neural network models is a topic of rapidly increasing interest in the artificial intelligence community. Spiking neural networks (SNNs) are biologically inspired models that strive to leverage the energy efficiency stemming from a long process of evolution under limited resources. In this paper we propose a SNN model where each neuron integrates piecewise linear postsynaptic potentials caused by input spikes and a positive bias, and spikes maximally once. Transformation of such a network into the ANN domain yields an approximation of a standard ReLU network, leading to a facilitated training based on backpropagation and an adaptation of the batch normalization. With backpropagation-trained weights, SNN inference offers a sparse-signal and low-latency classification, which can be readily adapted for a stream of input patterns, lending itself to an efficient hardware implementation. The supervised classification of MNIST and Fashion-MNIST datasets, using this approach, provides accuracy close to that of an ANN and surpassing other single-spike SNNs.
更多查看译文
关键词
spiking neural network, one spike per neuron, image processing, ReLU, efficient classification
AI 理解论文
溯源树
样例
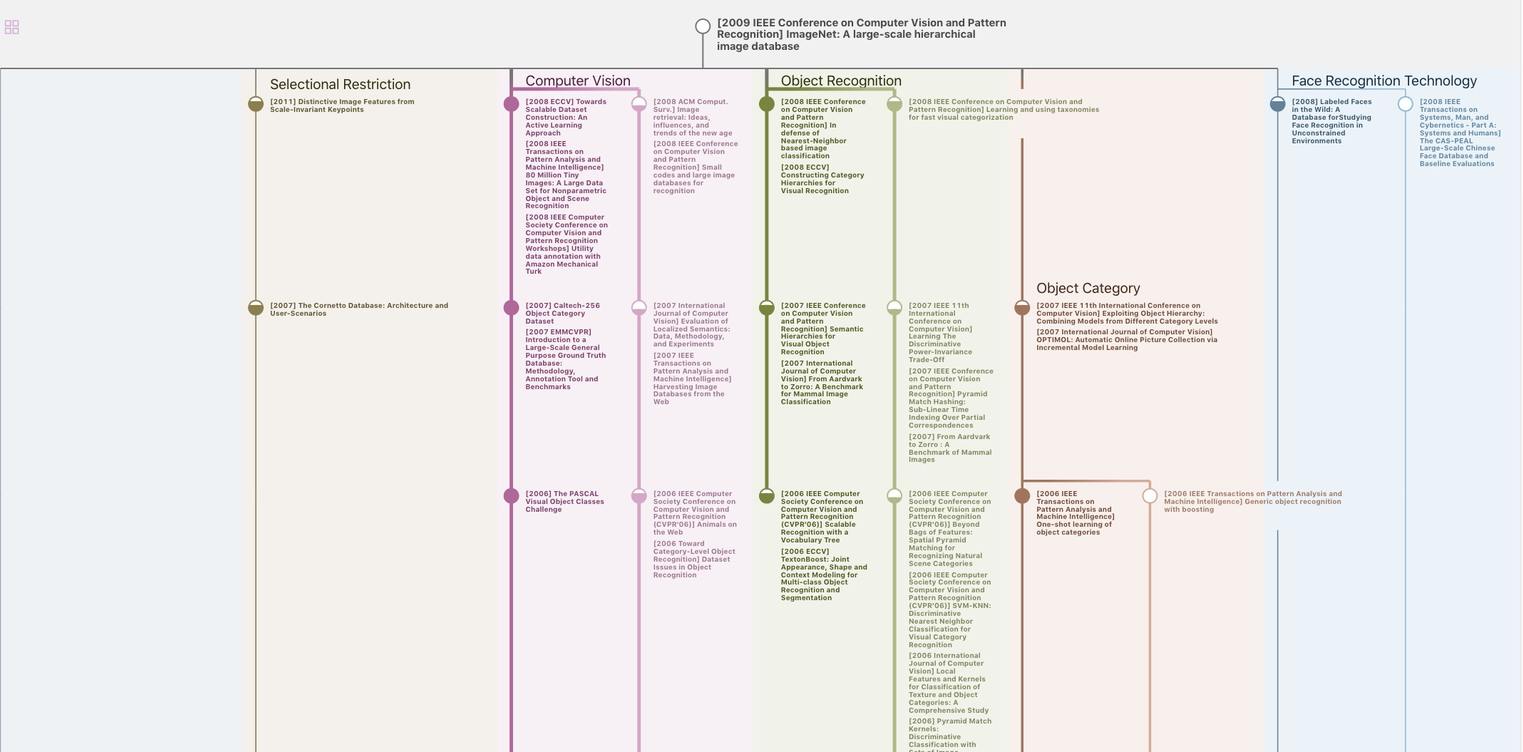
生成溯源树,研究论文发展脉络
Chat Paper
正在生成论文摘要