Identifying Targeted and Generalized Offensive Speech from Anti-asian Social Media Conversations.
CSoNet(2022)
摘要
During the Covid-19 pandemic Asian-Americans have been targets of prejudice and negative stereotyping. There has also been volumes of counter speech condemning this jaundiced attitude. Ironically, however, the dialogue on both sides is filled with offensive and abusive language. While abusive language directed at Asians encourages violence and hate crimes against this ethnic group, the use of derogatory language to insult alternative points of view showcases utter lack of respect and exploits people’s fears to stir up social tensions. It is thus important to identify and demote both types of offensive content from anti-Asian social media conversations. The goal of this paper is to present a machine learning framework that can achieve the dual objective of detecting targeted anti-Asian bigotry as well as generalized offensive content. Tweets were collected using the hashtag #chinavirus . Each tweet was annotated in two ways; either it condemned or condoned anti-Asian bias, and whether it was offensive or non-offensive. A rich set of features both from the text and accompanying numerical data were extracted. These features were used to train conventional machine learning and deep learning models. Our results show that the Random Forest classifier can detect both generalized and targeted offensive content with around 0.88 accuracy and F1-score. Our results are promising from two perspectives. First, our approach outperforms contemporary efforts on detecting online abuse against Asian-Americans. Second, our unified approach detects both offensive speech targeted specifically at Asian-Americans and also identifies its generalized form which has the potential to mobilize a large number of people in socially challenging situations.
更多查看译文
关键词
generalized offensive speech,targeted,anti-asian
AI 理解论文
溯源树
样例
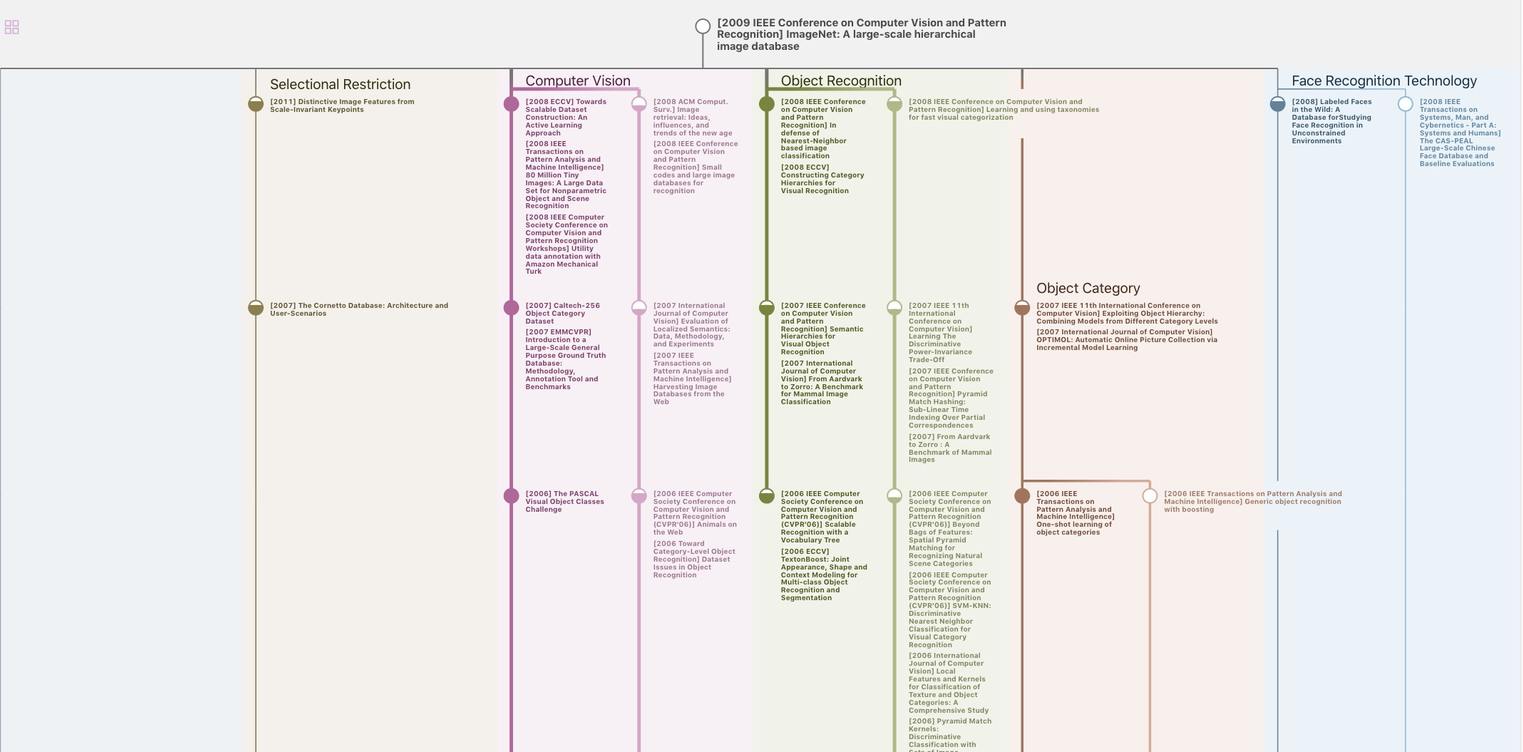
生成溯源树,研究论文发展脉络
Chat Paper
正在生成论文摘要