Towards Ultra-Low Power OFDMA Downlink Demodulation.
ACM International Conference on Embedded Networked Sensor Systems(2022)
Shanghai Jiao Tong Univ | Chinese Acad Sci
Abstract
OFDMA downlink design allowing parallel processing OFDM subcarriers is adopted by a number of commercial wireless standards such as LTE, 5G, and 802.11ax. However, the widespread adoption of OFDMA downlink on low-end IoT devices is stymied due to the existing digital receiver framework's ≈100mW power consumption, which is mainly incurred by LO+mixer, ADC, and complex digital processing. In this paper, we present an ultra-low-power OFDMA downlink demodulation design, which achieves ≈100 μW receiving power. Our basic idea is to transform the current digital demodulation approach into the analog one based on filtering, which avoids those power-hungry components. We achieve this by proposing a series of novel RF front-end hardware designs: 1) a μ W-level two-stage mixing scheme that enables adjustable and precise subcarrier filtering, 2) a quartz crystal-based filter circuit incurring negligible insertion loss, and 3) a passive phase-to-envelope conversion technique enabling low-power non-coherent phase demodulation. We build a prototype to verify the proposed schemes. Experimental and IC simulation results show that: our new design can achieve 130 -- 1500 times power savings depending on the number of subcarriers that need to be processed in parallel, compared with the traditional all-digital design.
MoreTranslated text
求助PDF
上传PDF
View via Publisher
AI Read Science
AI Summary
AI Summary is the key point extracted automatically understanding the full text of the paper, including the background, methods, results, conclusions, icons and other key content, so that you can get the outline of the paper at a glance.
Example
Background
Key content
Introduction
Methods
Results
Related work
Fund
Key content
- Pretraining has recently greatly promoted the development of natural language processing (NLP)
- We show that M6 outperforms the baselines in multimodal downstream tasks, and the large M6 with 10 parameters can reach a better performance
- We propose a method called M6 that is able to process information of multiple modalities and perform both single-modal and cross-modal understanding and generation
- The model is scaled to large model with 10 billion parameters with sophisticated deployment, and the 10 -parameter M6-large is the largest pretrained model in Chinese
- Experimental results show that our proposed M6 outperforms the baseline in a number of downstream tasks concerning both single modality and multiple modalities We will continue the pretraining of extremely large models by increasing data to explore the limit of its performance
Upload PDF to Generate Summary
Must-Reading Tree
Example
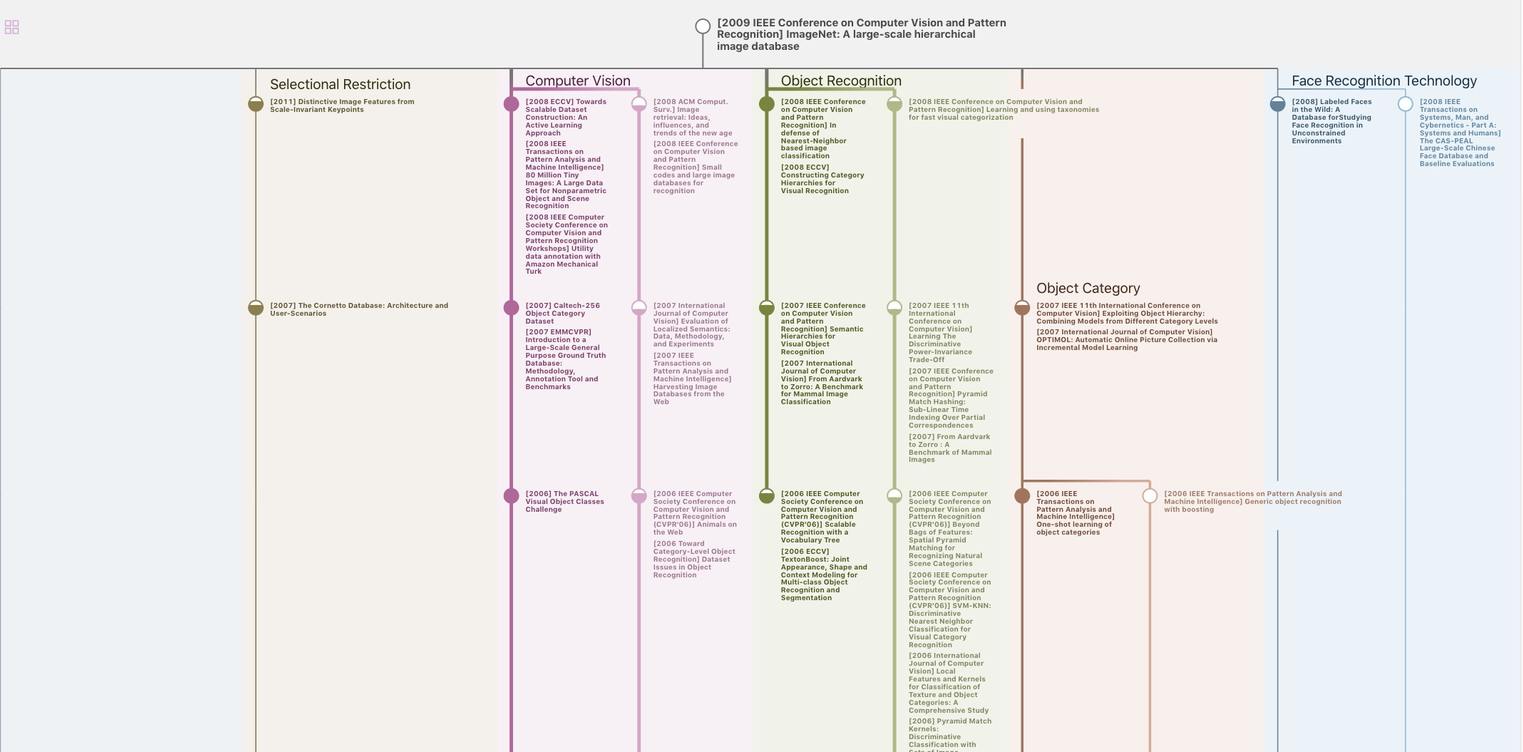
Generate MRT to find the research sequence of this paper
Related Papers
2012
被引用34 | 浏览
2015
被引用73 | 浏览
2014
被引用153 | 浏览
2017
被引用17 | 浏览
2017
被引用37 | 浏览
2018
被引用43 | 浏览
2019
被引用62 | 浏览
2018
被引用18 | 浏览
2017
被引用33 | 浏览
2019
被引用101 | 浏览
2018
被引用52 | 浏览
Data Disclaimer
The page data are from open Internet sources, cooperative publishers and automatic analysis results through AI technology. We do not make any commitments and guarantees for the validity, accuracy, correctness, reliability, completeness and timeliness of the page data. If you have any questions, please contact us by email: report@aminer.cn
Chat Paper