Adversarially Robust Neural Lyapunov Control
ICLR 2023(2023)
摘要
State-of-the-art learning-based stability control methods for nonlinear robotic systems suffer from the issue of reality gap, which stems from discrepancy of the system dynamics between training and target (test) environments. To mitigate this gap, we propose an adversarially robust neural Lyapunov control (ARNLC) method to improve the robustness and generalization capabilities for Lyapunov theory-based stability control. Specifically, inspired by adversarial learning, we introduce an adversary to simulate the dynamics discrepancy, which is learned through deep reinforcement learning to generate the worst-case perturbations during the controller's training. By alternatively updating the controller to minimize the perturbed Lyapunov risk and the adversary to deviate the controller from its objective, the learned control policy enjoys a theoretical guarantee of stability. Empirical evaluations on five stability control tasks with the uniform and worst-case perturbations demonstrate that ARNLC not only accelerates the convergence to asymptotic stability, but can generalize better in the entire perturbation space.
更多查看译文
AI 理解论文
溯源树
样例
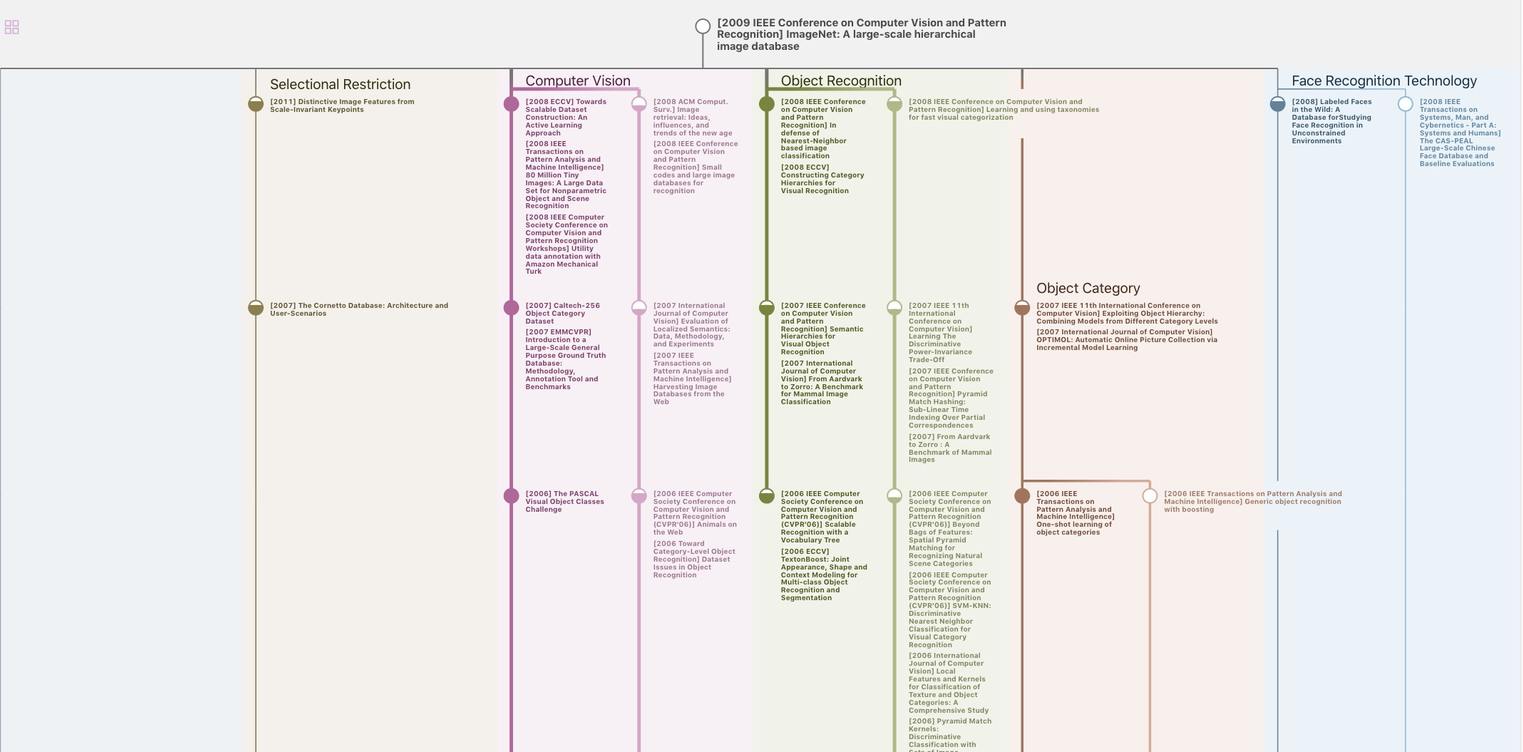
生成溯源树,研究论文发展脉络
Chat Paper
正在生成论文摘要