Data-Driven Modelling of Gene Expression States in Breast Cancer and their Prediction from Routine Whole Slide Images
bioRxiv (Cold Spring Harbor Laboratory)(2023)
摘要
Identification of gene expression state of a cancer patient from routine pathology imaging and characterization of its phenotypic effects have significant clinical and therapeutic implications. However, prediction of expression of individual genes from whole slide images (WSIs) is challenging due to co-dependent or correlated expression of multiple genes. Here, we use a purely data-driven approach to first identify groups of genes with co-dependent expression and then predict their status from (WSIs) using a bespoke graph neural network. These gene groups allow us to capture the gene expression state of a patient with a small number of binary variables that are biologically meaningful and carry histopathological insights for clinically and therapeutic use cases. Prediction of gene expression state based on these gene groups allows associating histological phenotypes (cellular composition, mitotic counts, grading, etc.) with underlying gene expression patterns and opens avenues for gaining significant biological insights from routine pathology imaging directly.
更多查看译文
关键词
gene expression states,breast cancer,prediction,data-driven
AI 理解论文
溯源树
样例
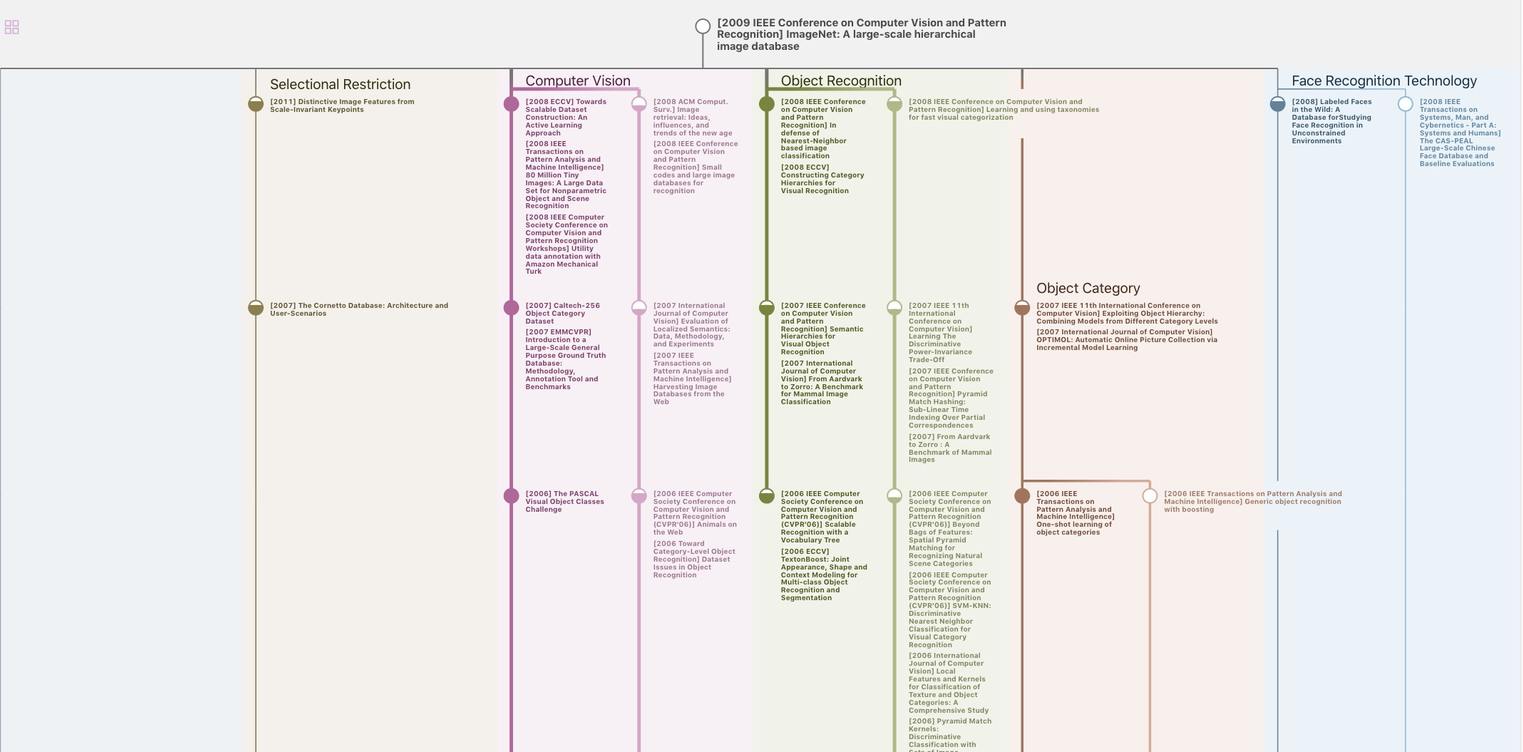
生成溯源树,研究论文发展脉络
Chat Paper
正在生成论文摘要