Continuous Versatile Jumping Using Learned Action Residuals
CoRR(2023)
摘要
Jumping is essential for legged robots to traverse through difficult terrains. In this work, we propose a hierarchical framework that combines optimal control and reinforcement learning to learn continuous jumping motions for quadrupedal robots. The core of our framework is a stance controller, which combines a manually designed acceleration controller with a learned residual policy. As the acceleration controller warm starts policy for efficient training, the trained policy overcomes the limitation of the acceleration controller and improves the jumping stability. In addition, a low-level whole-body controller converts the body pose command from the stance controller to motor commands. After training in simulation, our framework can be deployed directly to the real robot, and perform versatile, continuous jumping motions, including omni-directional jumps at up to 50cm high, 60cm forward, and jump-turning at up to 90 degrees. Please visit our website for more results: https://sites.google.com/view/learning-to-jump.
更多查看译文
关键词
action
AI 理解论文
溯源树
样例
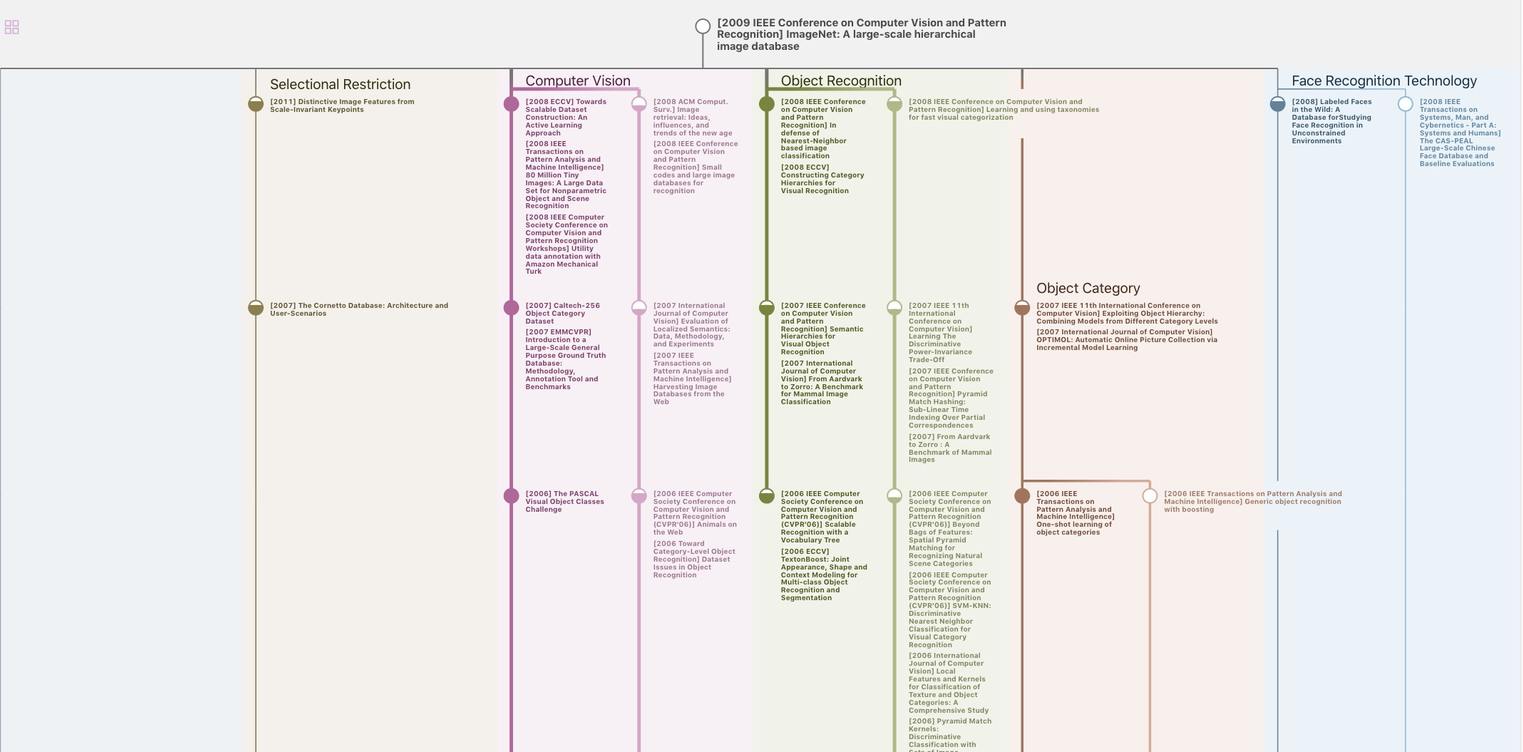
生成溯源树,研究论文发展脉络
Chat Paper
正在生成论文摘要