Bridging the gap between mechanistic biological models and machine learning surrogates.
PLoS computational biology(2023)
摘要
Mechanistic models have been used for centuries to describe complex interconnected processes, including biological ones. As the scope of these models has widened, so have their computational demands. This complexity can limit their suitability when running many simulations or when real-time results are required. Surrogate machine learning (ML) models can be used to approximate the behaviour of complex mechanistic models, and once built, their computational demands are several orders of magnitude lower. This paper provides an overview of the relevant literature, both from an applicability and a theoretical perspective. For the latter, the paper focuses on the design and training of the underlying ML models. Application-wise, we show how ML surrogates have been used to approximate different mechanistic models. We present a perspective on how these approaches can be applied to models representing biological processes with potential industrial applications (e.g., metabolism and whole-cell modelling) and show why surrogate ML models may hold the key to making the simulation of complex biological systems possible using a typical desktop computer.
更多查看译文
关键词
mechanistic biological models,learning
AI 理解论文
溯源树
样例
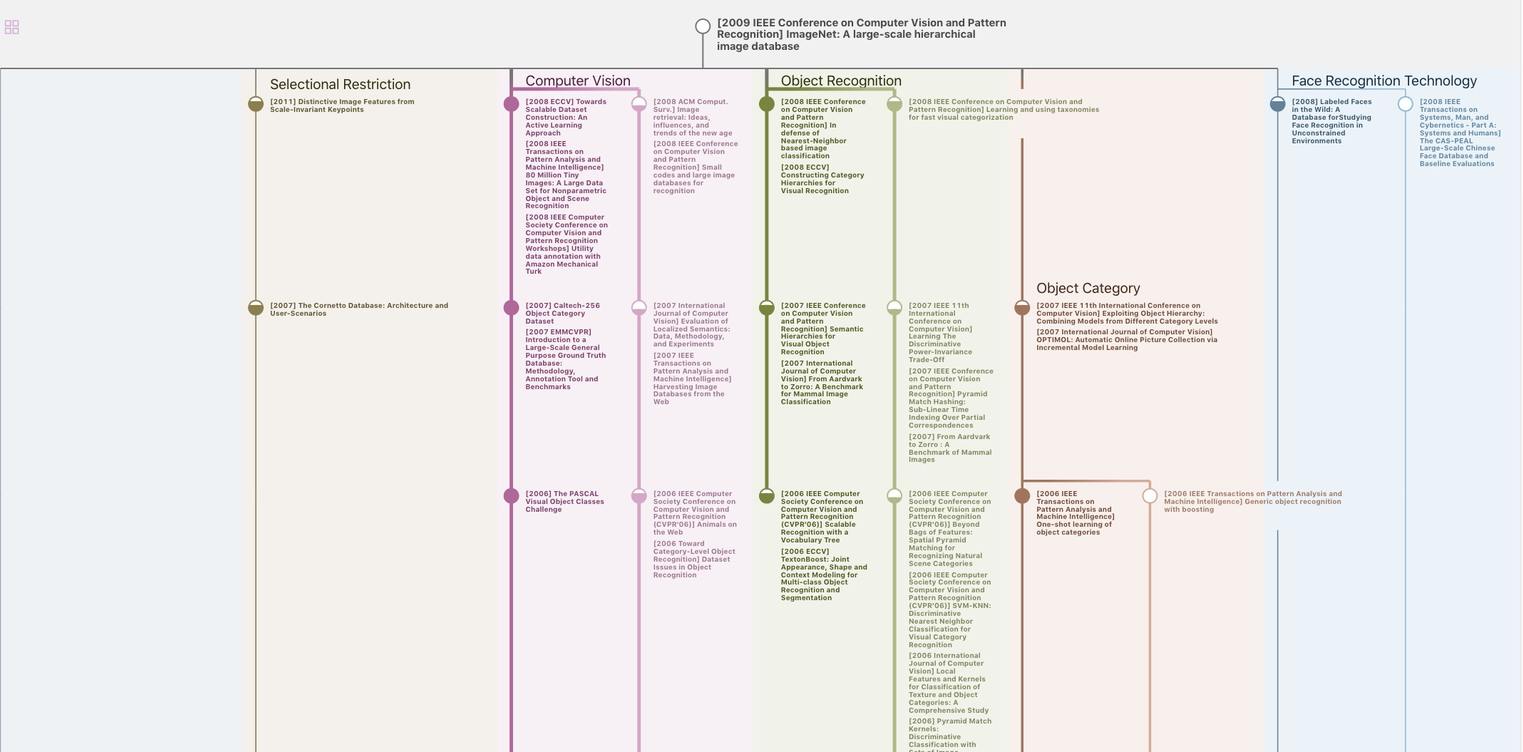
生成溯源树,研究论文发展脉络
Chat Paper
正在生成论文摘要