Is this AI trained on Credible Data? The Effects of Labeling Quality and Performance Bias on User Trust
CHI 2023(2023)
摘要
To promote data transparency, frameworks such as CrowdWorkSheets encourage documentation of annotation practices on the interfaces of AI systems, but we do not know how they affect user experience. Will the quality of labeling affect perceived credibility of training data? Does the source of annotation matter? Will a credible dataset persuade users to trust a system even if it shows racial biases in its predictions? To find out, we conducted a user study (N = 430) with a prototype of a classification system, using a 2 (labeling quality: high vs. low) × 4 (source: others-as-source vs. self-as-source cue vs. self-as-source voluntary action, vs. self-as-source forced action) × 3 (AI performance: none vs. biased vs. unbiased) experiment. We found that high-quality labeling leads to higher perceived training data credibility, which in turn enhances users’ trust in AI, but not when the system shows bias. Practical implications for explainable and ethical AI interfaces are discussed.
更多查看译文
关键词
training data credibility, data labeling quality, labeling source, trust in AI, algorithmic bias
AI 理解论文
溯源树
样例
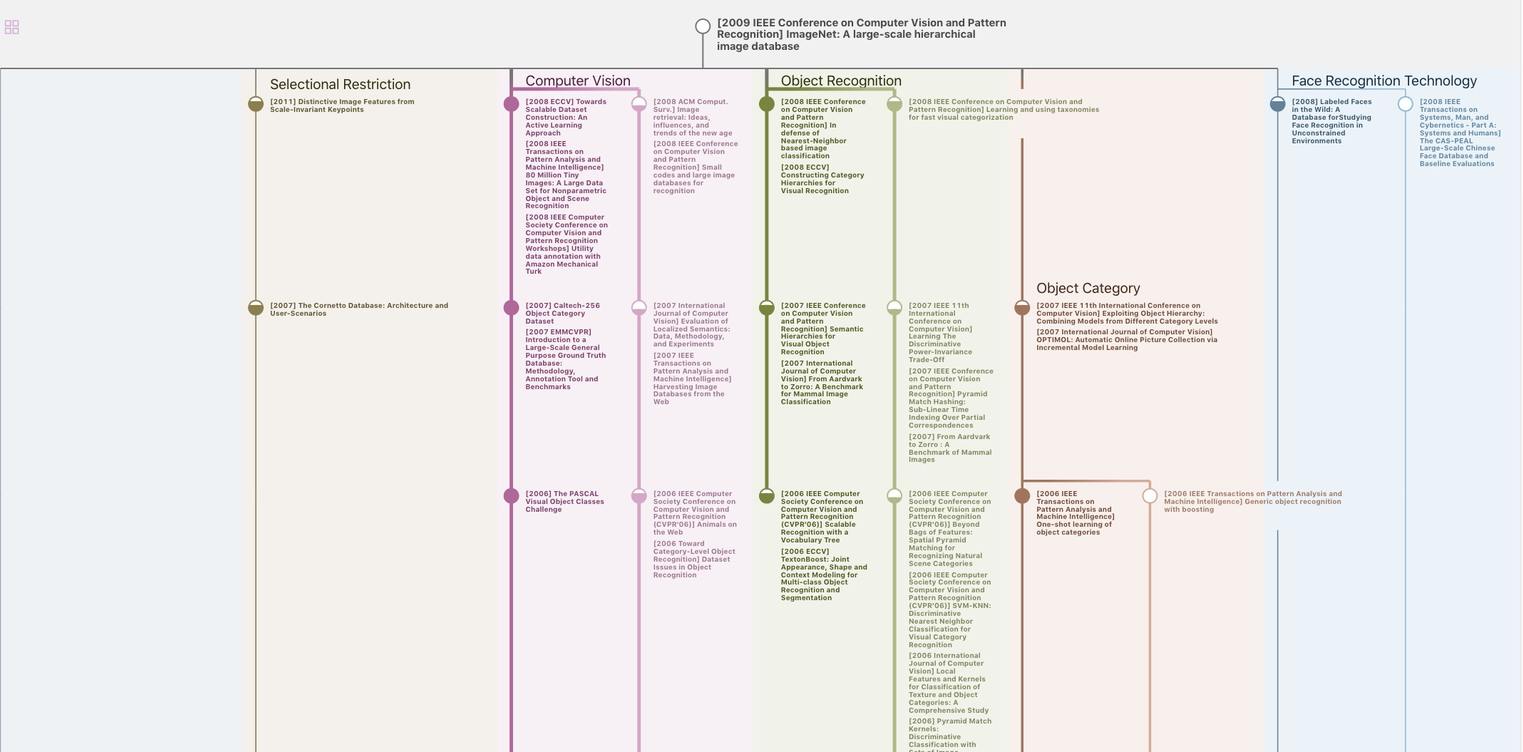
生成溯源树,研究论文发展脉络
Chat Paper
正在生成论文摘要