Inferring untrained complex dynamics of delay systems using an adapted echo state network.
CoRR(2021)
摘要
Caused by finite signal propagation velocities, many complex systems feature time delays that may induce high-dimensional chaotic behavior and make forecasting intricate. Here, we propose an echo state network adaptable to the physics of systems with arbitrary delays. After training the network to forecast a system with a unique and sufficiently long delay, it already learned to predict the system dynamics for all other delays. A simple adaptation of the network's topology allows us to infer untrained features such as high-dimensional chaotic attractors, bifurcations, and even multistabilities, that emerge with shorter and longer delays. Thus, the fusion of physical knowledge of the delay system and data-driven machine learning yields a model with high generalization capabilities and unprecedented prediction accuracy.
更多查看译文
关键词
echo state network,delay systems,untrained complex dynamics
AI 理解论文
溯源树
样例
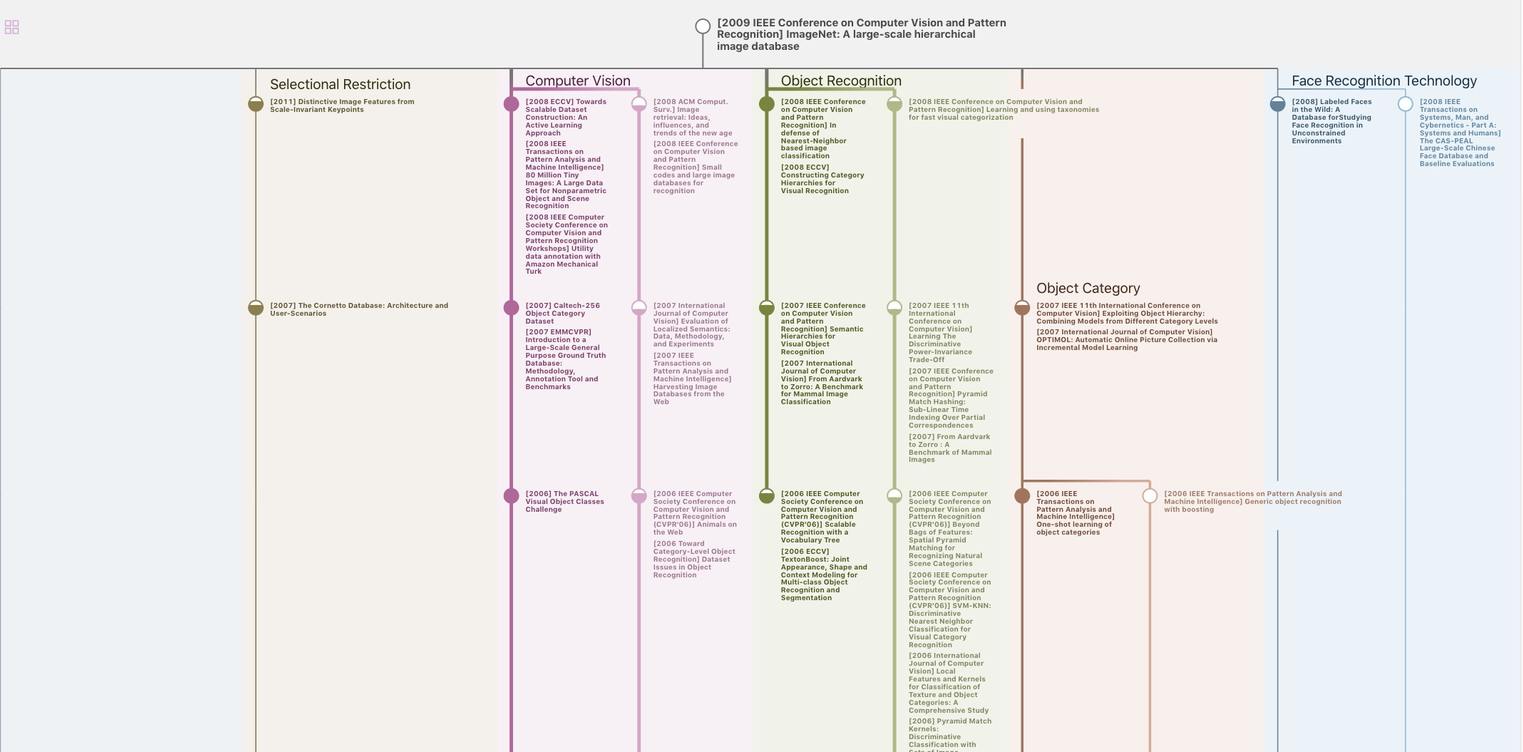
生成溯源树,研究论文发展脉络
Chat Paper
正在生成论文摘要