MS_HGNN: a Hybrid Online Fraud Detection Model to Alleviate Graph-Based Data Imbalance.
CONNECTION SCIENCE(2023)
Hunan Normal Univ | Providence Univ
Abstract
Online transaction fraud has become increasingly rampant due to the convenience of mobile payment. Fraud detection is critical to ensure the security of online transactions. With the development of graph neural network, researchers have applied it to the field of fraud detection. The existing fraud detection methods will solve the class imbalance by sampling, but they do not fully consider the various imbalances in the heterogeneous graph, and the data imbalance will directly affect the performance of the model. This work proposes a hybrid graph neural network model for online fraud detection to address this issue. The three types of imbalance in online transactions are feature imbalance, category imbalance, and relation imbalance, and they are all addressed in the proposed model. The entities with the feature most closely related to the fraudsters will be determined for the feature imbalance, and samples will be taken for further identification in the subsequent training phase. The hybrid model then uses under-sampling in combination with the long-distance sampling to find nodes with high similarity of features for the category imbalance. Finally, we propose a reward/punishment mechanism based on reinforcement learning for relation imbalance, which uses the threshold created by training as the sampling weight between relations. This paper conducts experiments on the public datasets Amazon and Yelp. The experimental results show that the model proposed is 5.61% higher than the best model in the comparison model on Amazon dataset, and 1.58% higher on Yelp dataset.
MoreTranslated text
Key words
Fraud detection,Data imbalance,Graph neural network,Online transaction,Reinforcement learning
求助PDF
上传PDF
View via Publisher
AI Read Science
AI Summary
AI Summary is the key point extracted automatically understanding the full text of the paper, including the background, methods, results, conclusions, icons and other key content, so that you can get the outline of the paper at a glance.
Example
Background
Key content
Introduction
Methods
Results
Related work
Fund
Key content
- Pretraining has recently greatly promoted the development of natural language processing (NLP)
- We show that M6 outperforms the baselines in multimodal downstream tasks, and the large M6 with 10 parameters can reach a better performance
- We propose a method called M6 that is able to process information of multiple modalities and perform both single-modal and cross-modal understanding and generation
- The model is scaled to large model with 10 billion parameters with sophisticated deployment, and the 10 -parameter M6-large is the largest pretrained model in Chinese
- Experimental results show that our proposed M6 outperforms the baseline in a number of downstream tasks concerning both single modality and multiple modalities We will continue the pretraining of extremely large models by increasing data to explore the limit of its performance
Upload PDF to Generate Summary
Must-Reading Tree
Example
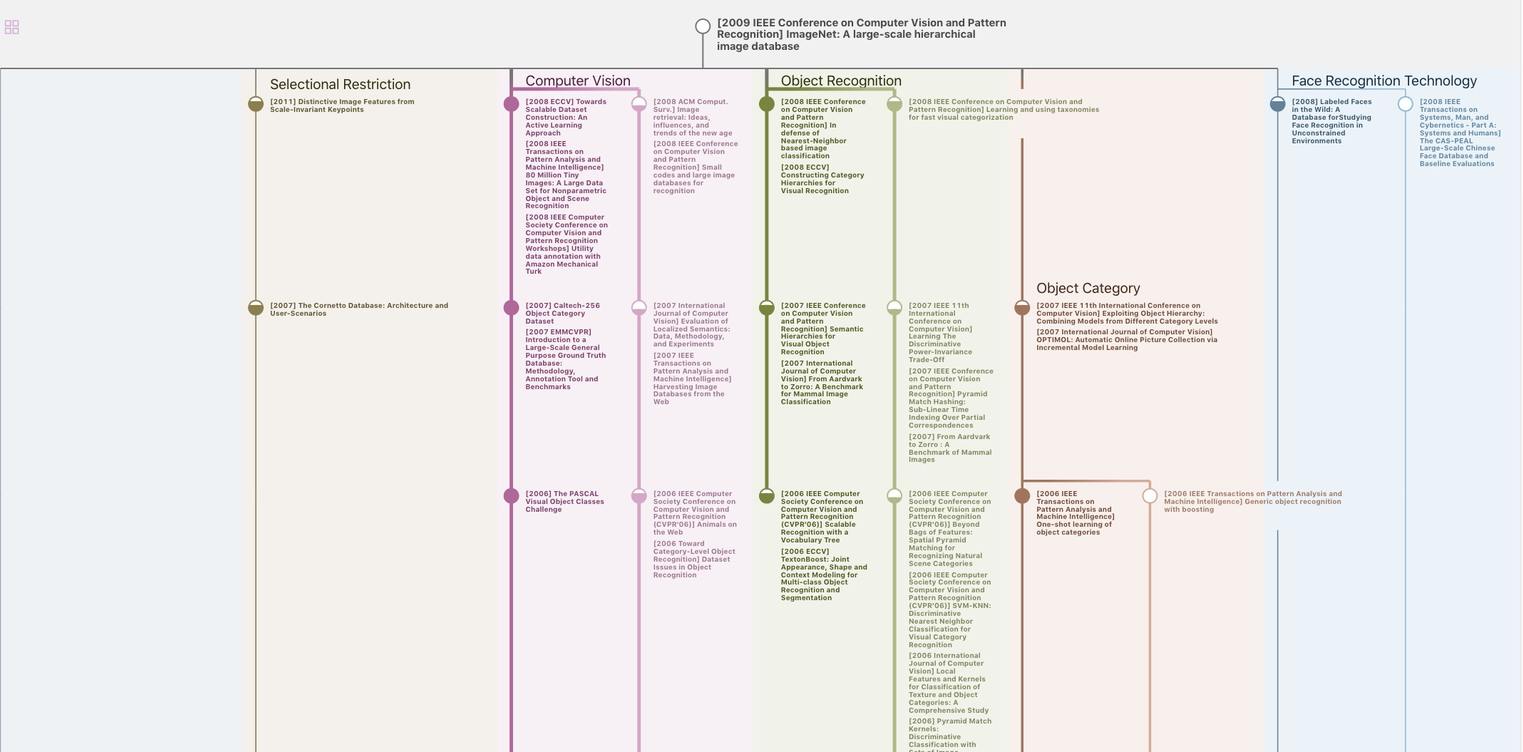
Generate MRT to find the research sequence of this paper
Related Papers
2018
被引用371 | 浏览
2018
被引用23 | 浏览
2019
被引用543 | 浏览
2019
被引用215 | 浏览
2019
被引用156 | 浏览
2019
被引用86 | 浏览
2019
被引用8 | 浏览
2019
被引用73 | 浏览
2020
被引用304 | 浏览
2020
被引用169 | 浏览
2020
被引用101 | 浏览
2022
被引用190 | 浏览
2021
被引用40 | 浏览
2021
被引用18 | 浏览
2021
被引用12 | 浏览
2023
被引用32 | 浏览
2020
被引用420 | 浏览
Data Disclaimer
The page data are from open Internet sources, cooperative publishers and automatic analysis results through AI technology. We do not make any commitments and guarantees for the validity, accuracy, correctness, reliability, completeness and timeliness of the page data. If you have any questions, please contact us by email: report@aminer.cn
Chat Paper