Investor Preference Analysis: an Online Optimization Approach with Missing Information
Information sciences(2023)
摘要
How to derive an investor's preference is vital for investment advisors and online lending platforms for targeted marketing strategies, e.g., market segmentation and financial product recommendation. However, investor preference analysis usually depends on judgments from human investment experts, which are inherently subjective and costly. Intelligent investment advisors (or Robo-advisors), supported by cutting-edge technologies such as machine learning and artificial intelligence, are established to relieve these pending issues. This paper employs an online optimization framework to obtain investors' preferences for further financial product recommendations. This proposed method allows us to update the investor's preference for newly-arriving data sets and tackle the situation where plenty of missing values in investors' records are present. Unlike the black-box-like machine learning approach, our method can provide more managerial implications regarding why one financial product/service is preferred. Real-world data set from an online financial platform is used to compare the existing approaches and shows the stronger and more stable performance of our method when facing different data-missing types and situations with different missing degrees, followed by a recommendation system application.
更多查看译文
关键词
Decision analysis,Online optimization,Investor preference derivation,Machine learning,Fintech
AI 理解论文
溯源树
样例
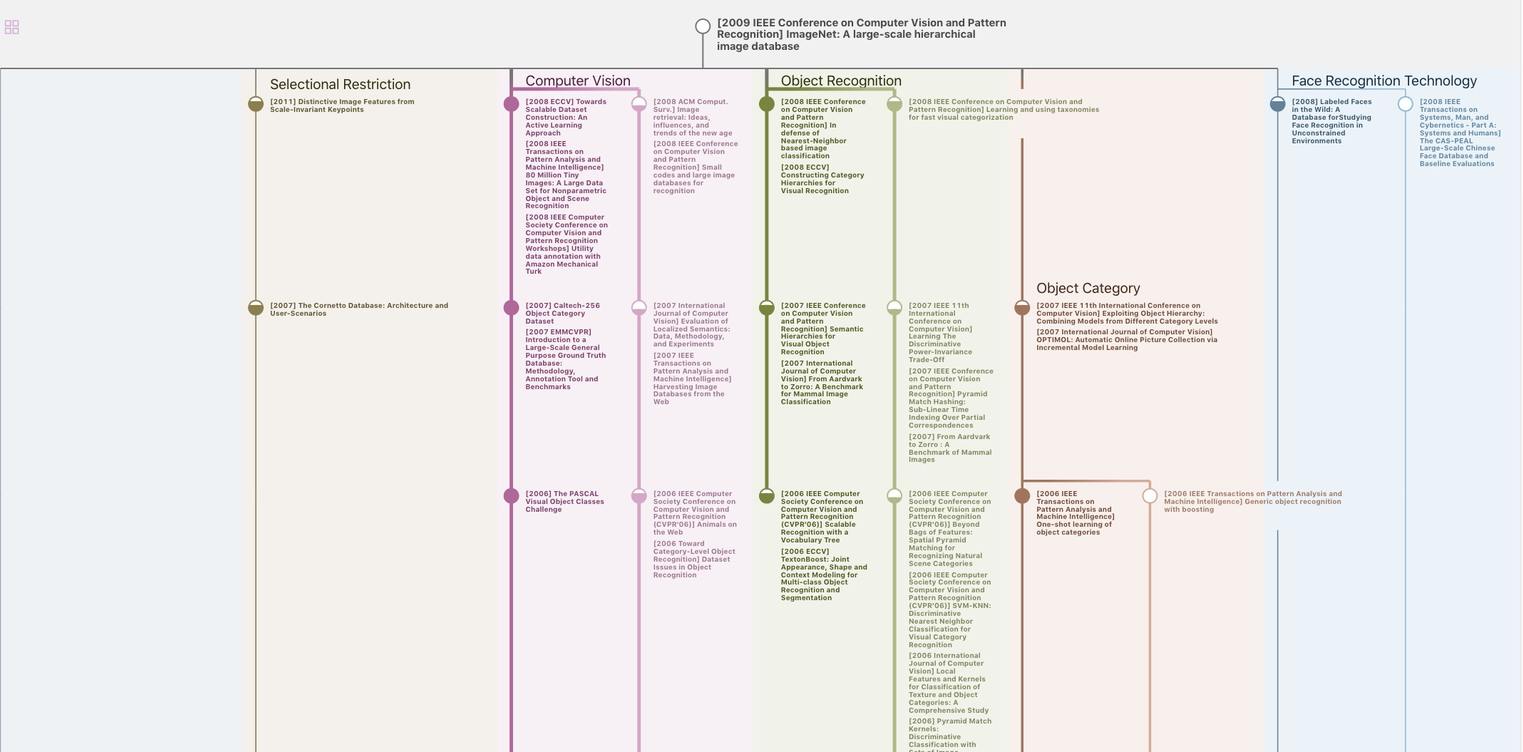
生成溯源树,研究论文发展脉络
Chat Paper
正在生成论文摘要