A multi-directional recurrent graph convolutional network model for reconstructing traffic spatiotemporal diagram
TRANSPORTATION LETTERS-THE INTERNATIONAL JOURNAL OF TRANSPORTATION RESEARCH(2023)
摘要
The Time Space Diagram (TSD) can abstractly represent multiple data sources and the macroscopic state of road traffic. However, the TSDs may be incomplete due to missing data, which seriously affects traffic management. Therefore, this paper proposed a Multi-Directional Recurrent Graph Convolutional Network (MDRGCN) for reconstructing TSDs and estimating missing traffic speeds given sparse data. We designed multi-directional RNN layers for scanning the TSDs from horizontal and vertical directions, which can fully exploit the contextual dependencies of the traffic information. In addition, our model includes graph convolution layers for mining potential spatial correlations in the TSDs. The performance of the model reconstructed from TSDs is validated on the NGSIM dataset. We also provided a comparison with other advanced methods, and the experimental results show that our method can perform well at both low and high missing rates, significantly outperforming the baseline methods.
更多查看译文
关键词
Time space diagram,traffic state reconstruction,traffic data imputation,graph convolutional neural networks,recurrent neural networks
AI 理解论文
溯源树
样例
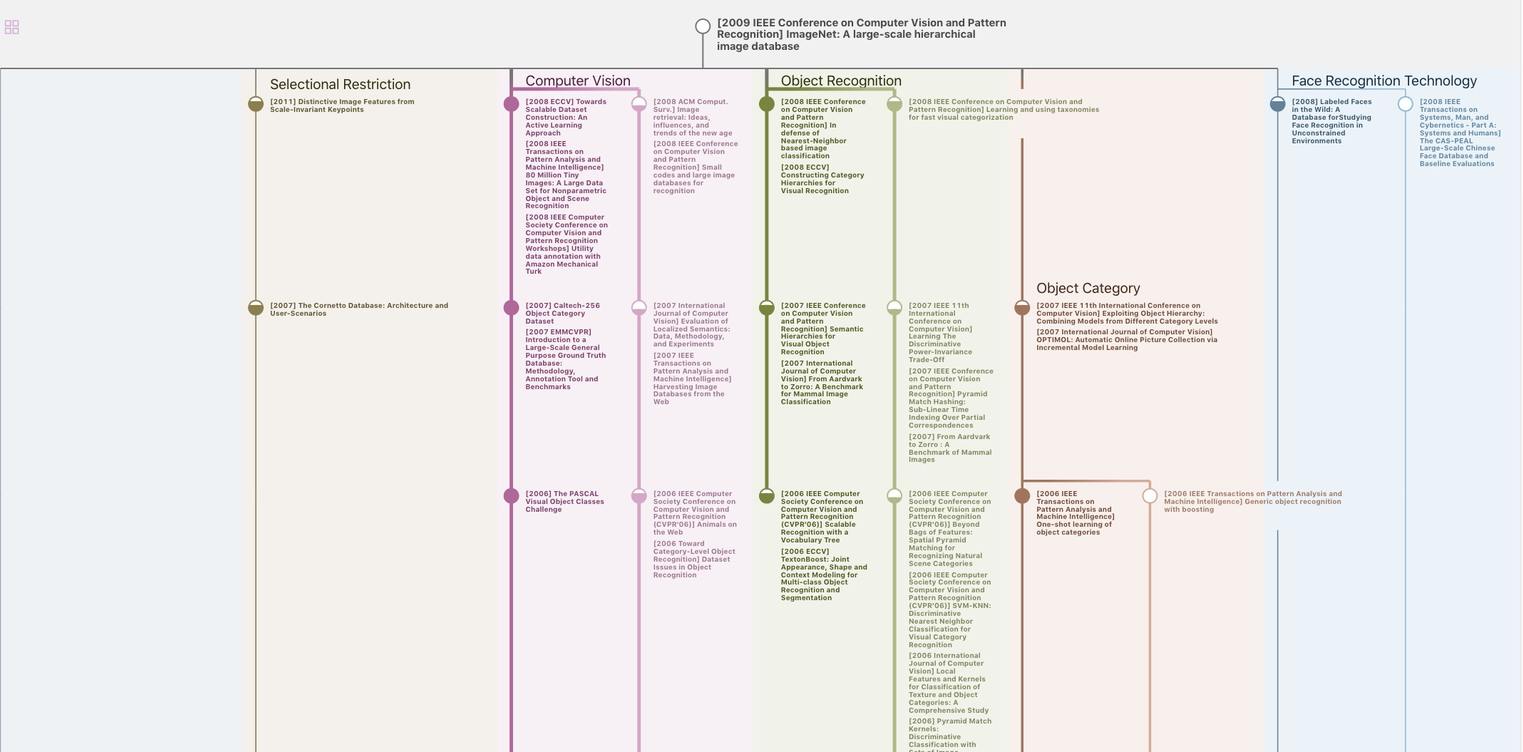
生成溯源树,研究论文发展脉络
Chat Paper
正在生成论文摘要