LMGQS: A Large-scale Dataset for Query-focused Summarization.
CoRR(2023)
摘要
Query-focused summarization (QFS) aims to extract or generate a summary of an input document that directly answers or is relevant to a given query. The lack of large-scale datasets in the form of documents, queries, and summaries has hindered model development in this area. In contrast, multiple large-scale high-quality datasets for generic summarization exist. We hypothesize that there is a hidden query for each summary sentence in a generic summarization annotation, and we utilize a large-scale pretrained language model to recover it. In this way, we convert four generic summarization benchmarks into a new QFS benchmark dataset, LMGQS, which consists of over 1 million document-query-summary samples. We thoroughly investigate the properties of our proposed dataset and establish baselines with state-of-the-art summarization models. By fine-tuning a language model on LMGQS, we achieve state-of-the-art zero-shot and supervised performance on multiple existing QFS benchmarks, demonstrating the high quality and diversity of LMGQS.
更多查看译文
关键词
dataset,large-scale,query-focused
AI 理解论文
溯源树
样例
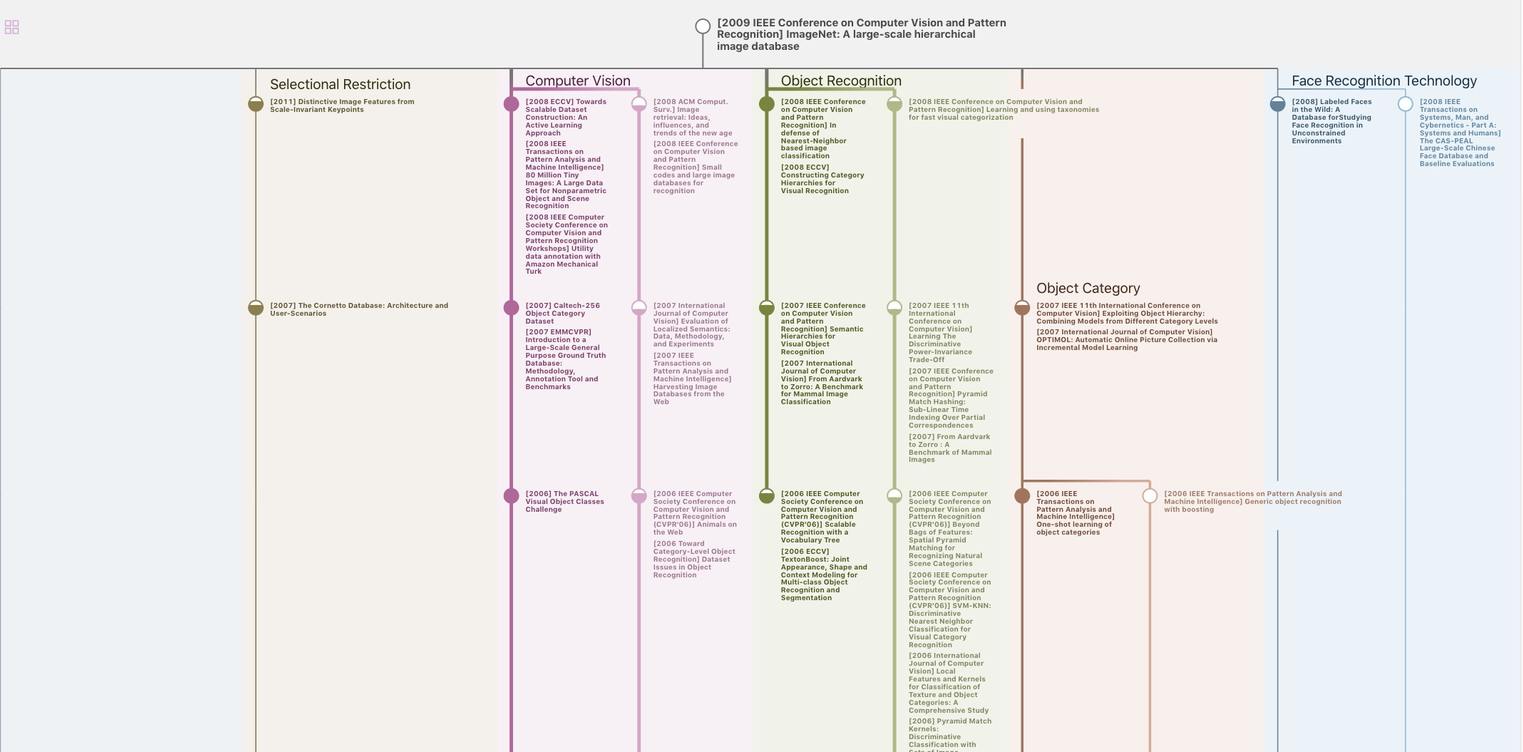
生成溯源树,研究论文发展脉络
Chat Paper
正在生成论文摘要