Tunnel Field-Effect Transistor Triggered Silicon-Controlled Rectifier
IEEE TRANSACTIONS ON ELECTRON DEVICES(2023)
关键词
TFETs,Junctions,Logic gates,Anodes,Integrated circuit modeling,Equivalent circuits,Rectifiers,Band-to-band tunneling (BTBT),electrostatic discharge (ESD),silicon-controlled rectifier (SCR),tunnel field-effect transistor (TFET)
AI 理解论文
溯源树
样例
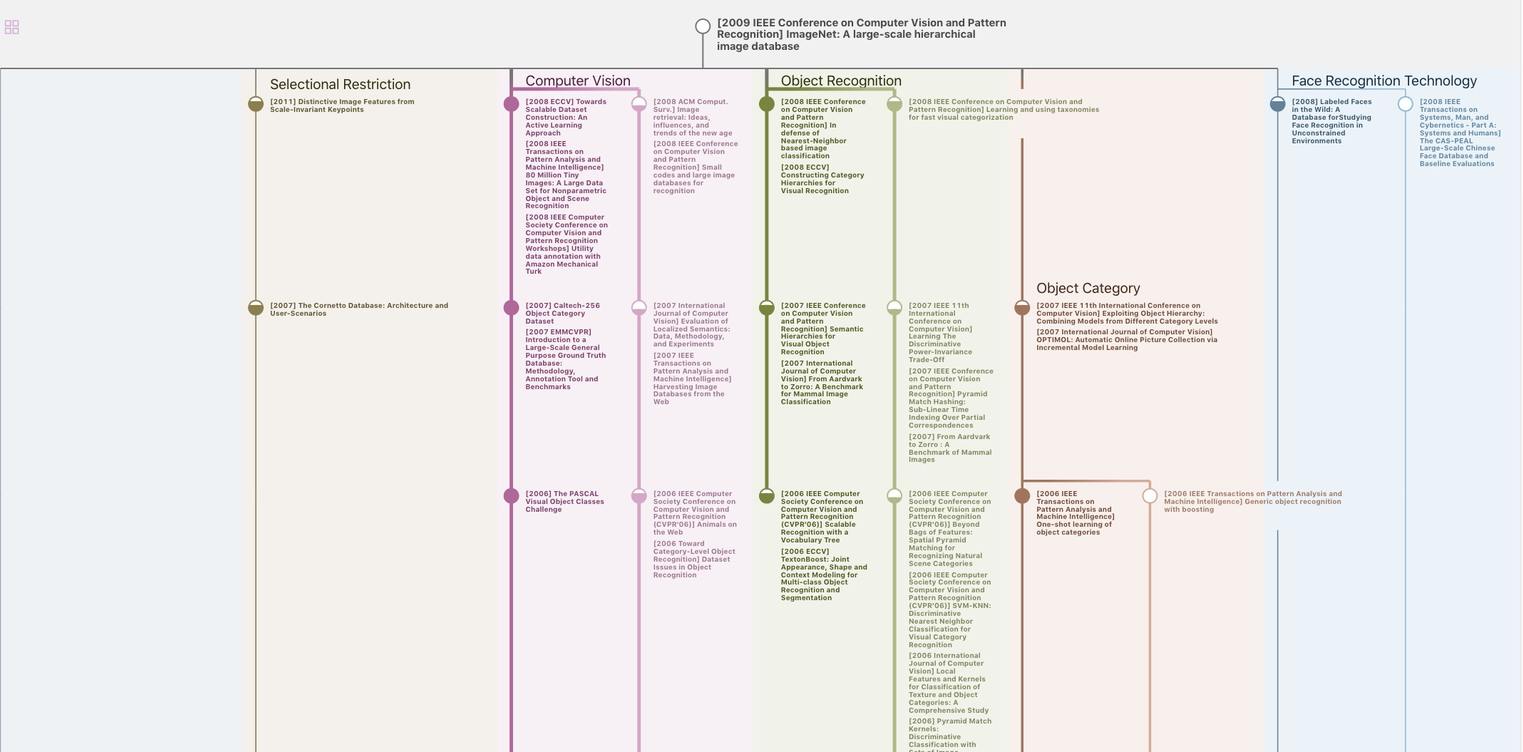
生成溯源树,研究论文发展脉络
Chat Paper
正在生成论文摘要