Optimizing Spaced Repetition Schedule by Capturing the Dynamics of Memory
IEEE TRANSACTIONS ON KNOWLEDGE AND DATA ENGINEERING(2023)
摘要
Spaced repetition, namely, learners review items in a given schedule, has been proven powerful for memorization and practice of skills. Most current spaced repetition methods focus on either predicting student recall or designing an optimal review schedule, thus omitting the integrity of the spaced repetition system. In this work, we propose a novel spaced repetition schedule framework by capturing the dynamics of memory, which alternates memory prediction and schedule optimization to improve the efficiency of learners’ reviews. First, the framework collects logs from students’ reviews and builds memory models with Markov property to capture the dynamics of memory. Then, the spaced repetition optimization is transformed a stochastic shortest path problem and solved via the value iteration method. We also construct a new benchmark dataset for spaced repetition, which is the first to contain time-series information during learners’ memorization. Experimental results on the collected data from the real world and the simulated environment demonstrate that the proposed approach reduces 64% error and 17% cost in predicting recall rates and optimizing schedules compared to several baselines. We have publicly released the dataset containing 220 million rows and codes used in this paper at: https://github.com/maimemo/SSP-MMC-Plus .
更多查看译文
关键词
Language learning,Markov decision process,recurrent neural networks,time-series features,spaced repetition
AI 理解论文
溯源树
样例
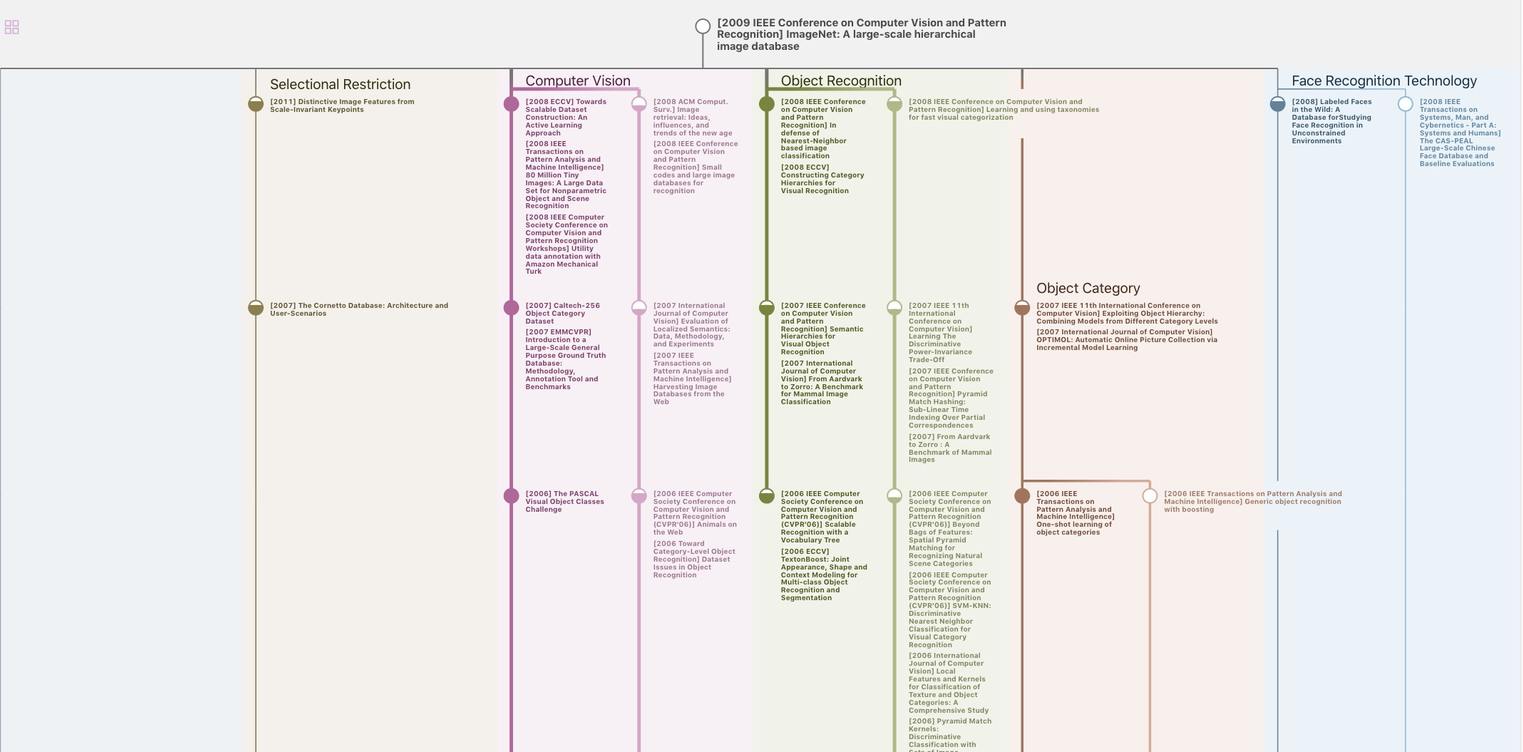
生成溯源树,研究论文发展脉络
Chat Paper
正在生成论文摘要