Hierarchical matching and reasoning for multi-query image retrieval
NEURAL NETWORKS(2024)
摘要
As a promising field, Multi-Query Image Retrieval (MQIR) aims at searching for the semantically relevant image given multiple region-specific text queries. Existing works mainly focus on a single-level similarity between image regions and text queries, which neglect the hierarchical guidance of multi-level similarities and result in incomplete alignments. Besides, the high-level semantic correlations that intrinsically connect different region- query pairs are rarely considered. To address above limitations, we propose a novel Hierarchical Matching and Reasoning Network (HMRN) for MQIR. It disentangles MQIR into three hierarchical semantic representations, which is responsible to capture fine-grained local details, contextual global scopes, and high-level inherent correlations. HMRN consists of two modules: Scalar-based Matching (SM) module and Vector-based Reasoning (VR) module. Specifically, the SM module characterizes the multi-level alignment similarity, which consists of a fine-grained local-level similarity and a context-aware global-level similarity. Afterwards, the VR module is developed to excavate the potential semantic correlations among multiple region-query pairs, which further explores the high-level reasoning similarity. Finally, these three-level similarities are aggregated into a joint similarity space to form the ultimate similarity. Extensive experiments on the benchmark dataset demonstrate that our HMRN substantially surpasses the current state -of -the -art methods. For instance, compared with the existing best method Drill -down, the metric R@1 in the last round is improved by 23.4%. Our source codes will be released at https://github.com/LZH-053/HMRN.
更多查看译文
关键词
Multi-query image retrieval,Multi-level alignment,High-level semantic correlation,Hierarchical structure
AI 理解论文
溯源树
样例
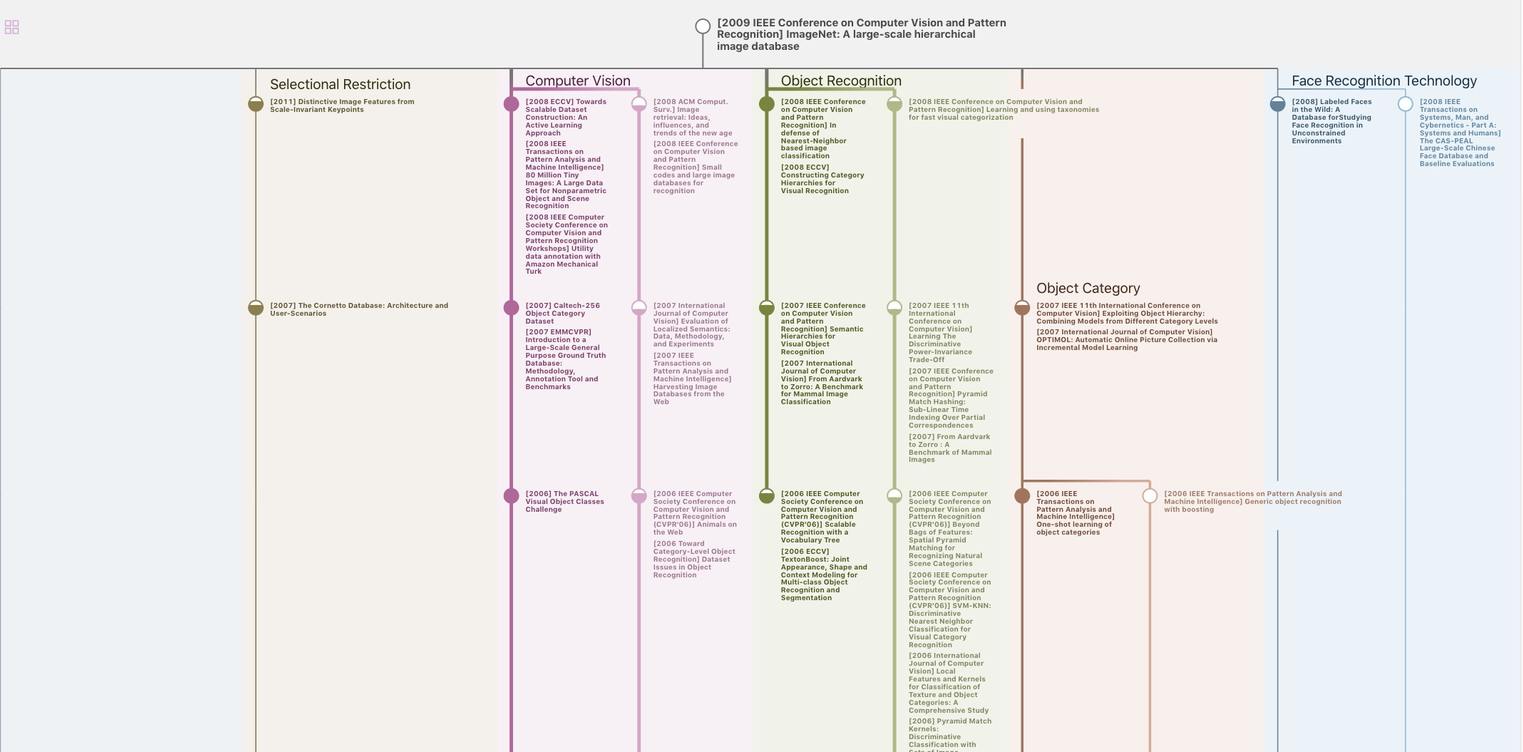
生成溯源树,研究论文发展脉络
Chat Paper
正在生成论文摘要