M3NetFlow: a novel multi-scale multi-hop modular graph AI model for multi-omics data integration and signaling network inference
bioRxiv (Cold Spring Harbor Laboratory)(2023)
摘要
The integration and interpretation of multi-omics data play a crucial role in systems biology for prioritizing key molecular targets and deciphering core signaling pathways of complex diseases, such as cancer, covid-19 and Alzheimer’s disease. However, it remains a challenge that has not been adequately addressed. Graph neural networks (GNN) have been emerged as powerful artificial intelligence models for analyzing data with a graphical structure. Nevertheless, GNN models have not been sufficiently designed for integrative and interpretable analysis of multi-omics data. In this study, we propose a novel multi-scale multi-hop modular GNN model, M3NetFlow, to integrate and interpret multi-omics data to rank key targets and infer core signaling pathways. Specifically, we applied the M3NetFlow model to infer cell-line specific core signaling networks explaining drug combination response. The evaluation and comparison results on drug combination prediction showed that the M3NetFlow model achieved significantly higher prediction accuracy than existing GNN models. Furthermore, M3NetFlow can predict key targets and infer essential signaling networks regulating drug combination response. It is critical for investigating mechanisms of synergy, and guiding the development of personalized precision medicine for patients with drug resistance. This model can be applied to general multi-omics data-driven research.
### Competing Interest Statement
The authors have declared no competing interest.
更多查看译文
关键词
network inference,graph,ai,modular,multi-scale,multi-hop,multi-omics
AI 理解论文
溯源树
样例
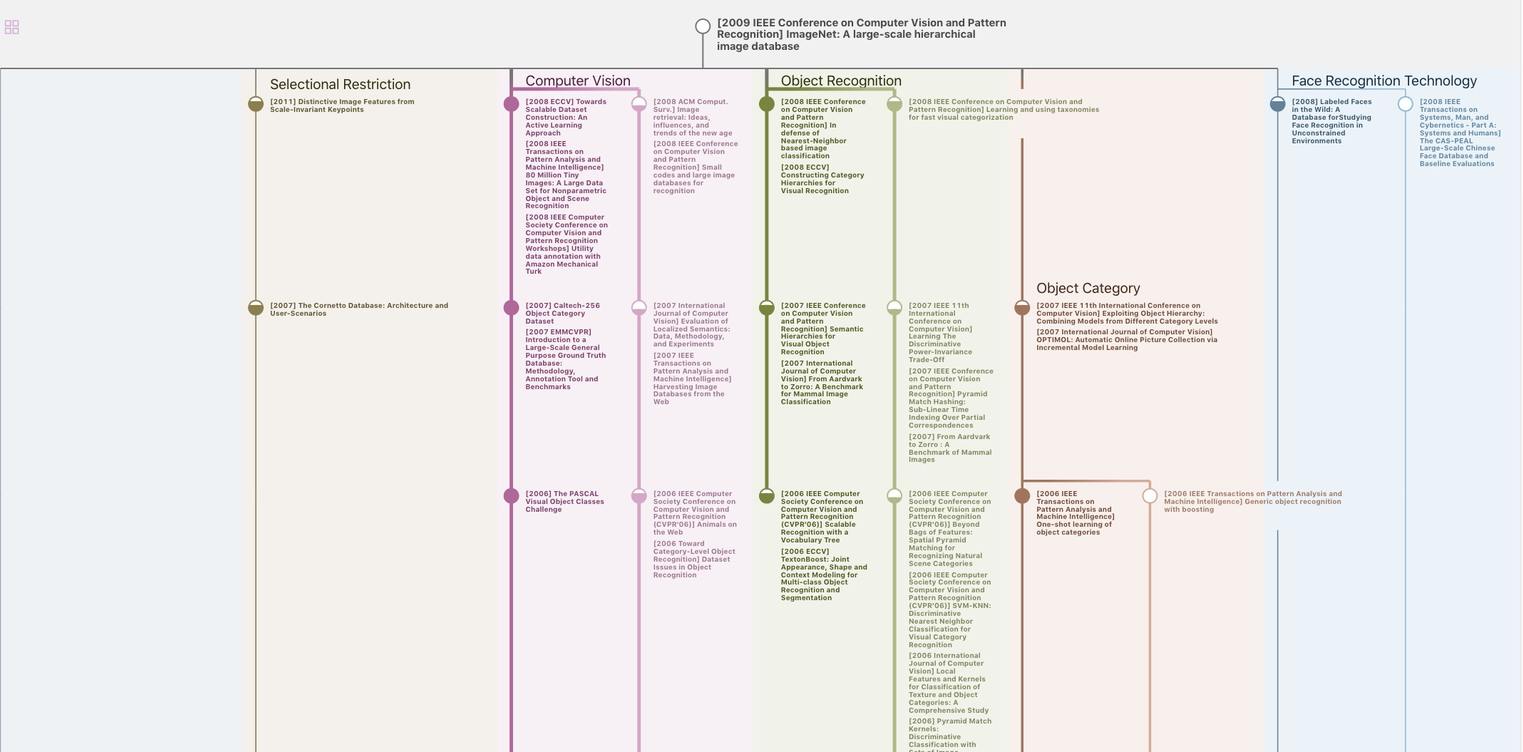
生成溯源树,研究论文发展脉络
Chat Paper
正在生成论文摘要